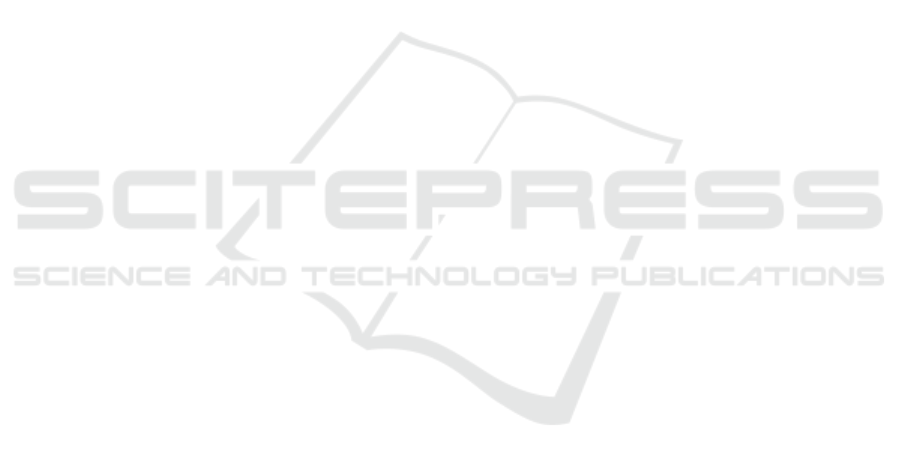
for myoelectric movement classification. IEEE Tran-
sactions on Neural Systems and Rehabilitation Engi-
neering, 23(1):73–83.
Castellini, C., Fiorilla, A., and Sandini, G. (2009). Multi-
subject/daily-life activity EMG-based control of me-
chanical hands. Journal of neuroengineering and re-
habilitation, 6:41.
Cheok, M., Omar, Z., and Jaward, M. (2017). A review
of hand gesture and sign language recognition techni-
ques. International Journal of Machine Learning and
Cybernetics.
C
ˆ
ot
´
e-Allard, U. et al. (2018). Deep Learning for elec-
tromyographic hand gesture signal classification by
leveraging transfer learning. ArXiv e-prints.
Du, Y., Jin, W., Wei, W., Hu, Y., and Geng, W. (2017).
Surface EMG-based inter-session gesture recogni-
tion enhanced by deep domain adaptation. Sensors,
17(3):458.
Englehart, K. and Hudgins, B. (2003). A robust, real-time
control scheme for multifunction myoelectric cont-
rol. IEEE Transactions on Biomedical Engineering,
50(7):848–854.
Englehart, K., Hudgins, B., Parker, P., and Stevenson, M.
(1999). Classification of the myoelectric signal using
time-frequency based representations. Medical Engi-
neering and Physics, 21(6):431–438.
Geng, W., Du, Y., Jin, W., Wei, W., Hu, Y., and Li, J.
(2016). Gesture recognition by instantaneous surface
EMG images. Scientific Reports, 6(36571).
Gijsberts, A., Atzori, M., Castellini, C., M
¨
uller, H., and
Caputo, B. (2014). Movement error rate for evalua-
tion of Machine Learning methods for sEMG-based
hand movement classification. IEEE Transactions
on Neural Systems and Rehabilitation Engineering,
22(4):735–744.
Glorot, X. and Bengio, Y. (2010). Understanding the diffi-
culty of training deep feedforward Neural Networks.
In Proceedings of the 13th International Conference
on Artificial Intelligence and Statistics (AISTATS),
Sardinia, Italy.
Goodfellow, I., Bengio, Y., and Courville, A. (2016). Deep
Learning. MIT Press, Cambridge, MA.
Hudgins, B., Parker, P., and Scott, R. (1993). A new strategy
for multifunction myoelectric control. IEEE Tran-
sactions on Biomedical Engineering, 40(1):82–94.
Kuzborskij, I., Gijsberts, A., and Caputo, B. (2012). On the
challenge of classifying 52 hand movements from sur-
face electromyography. In 2012 Annual International
Conference of the IEEE Engineering in Medicine and
Biology Society, pages 4931–4937. IEEE.
Li, Y., Wang, N., Shi, J., Liu, J., and Hou, X. (2016). Revi-
siting Batch Normalization for practical domain adap-
tation. ArXiv e-prints.
Park, K. and Lee, S. (2016). Movement intention decoding
based on Deep Learning for multiuser myoelectric in-
terfaces. In 2016 4th International Winter Conference
on Brain-Computer Interface (BCI), pages 1–2. IEEE.
Scheme, E. and Englehart, K. (2011). Electromyogram
pattern recognition for control of powered upper-limb
prostheses: State of the art and challenges for clini-
cal use. The Journal of Rehabilitation Research and
Development, 48(6):643–659.
Sergey, I. and Szegedy, C. (2015). Batch Normalization:
Accelerating Deep Network training by reducing in-
ternal covariate shift. ArXiv e-prints.
Shim, H., An, H., Lee, S., Lee, E., Min, H., and Lee, S.
(2016). EMG pattern classification by split and merge
deep belief network. Symmetry, 8(12):148.
Shim, H. and Lee, S. (2015). Multi-channel electromyo-
graphy pattern classification using deep belief net-
works for enhanced user experience. Journal of Cen-
tral South University, 22(5):1801–1808.
Srivastava, N., Hinton, G., Krizhevsky, A., Sutskever, I.,
and Salakhutdinov, R. (2014). Dropout: A simple way
to prevent Neural Networks from overfitting. Journal
of Machine Learning Research, 15:1929–1958.
Wei, W., Wong, Y., Du, Y., Hu, Y., Kankanhalli, M., and
Geng, W. (2017). A multi-stream Convolutional Neu-
ral Network for sEMG-based gesture recognition in
muscle-computer interface. Pattern Recognition Let-
ters.
Zhai, X., Jelfs, B., Chan, R., and Tin, C. (2017). Self-
recalibrating surface EMG pattern recognition for
neuroprosthesis control based on Convolutional Neu-
ral Network. Frontiers in Neuroscience, 11:379–390.
PhyCS 2018 - 5th International Conference on Physiological Computing Systems
114