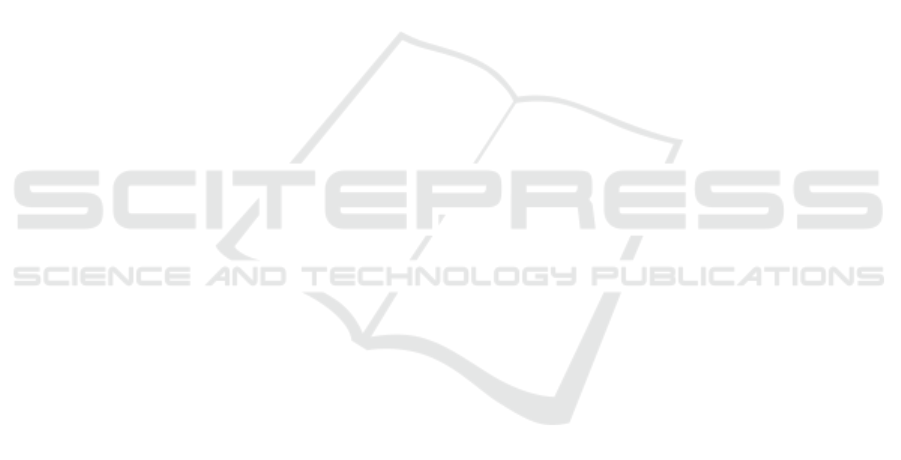
Comparison of Typical DS Conflict Evidence Improved Algorithms
Sunqing Xu, Junbao Geng, Shuhuan Wei and Kejia Wei
College of Power Engineering, Military Key Laboratory for Naval Ship Power Engineering, Wuhan 430033, China
College of Power Engineering, Naval University of Engineering, Wuhan 430033, China
xusunqing123@163.com
Keywords: Evidence theory, conflict, improved algorithm.
Abstract: Aiming at there will generate a counter-intuitive conclusion when DS evidence theory handle the highly
conflict evidence information, some of the existing methods of improvement do not solve these problems
well, and there is no unified, widely accepted scheme in the academia. Therefore, several typical improved
algorithms are introduced in this paper, and an example is given to show which method is more reasonable,
and the effect to handle conflict evidence is better. The results of the paper can provide the ideas for the
further research to solve the conflict of evidence.
1 INTRODUCTION
Dempster-Shafer (DS) theory of evidence was first
proposed by Dempster in 1967 in studying the
multi-valued mapping issues (Dempster, 1967).
Shafer further developed it into a systematic theory
of uncertain reasoning in Shafer (1976). DS
evidence theory is a common and efficient method
used to handle uncertain information. After Jeff
Barnett introduced the name “Dempster-Shafer” in
1981, the theory quickly acquired textbook status in
artificial intelligence (Barnett, 1981). The theory is
used in many branches of technology. Articles on
the theory and its applications appear in a
remarkable number of journals and recurring
conferences. Books on the theory continue to appear.
However, in some special situations, especially
when dealing with combination of the conflicting
evidences, Dempster’s combination rule may
produce the counter-intuitive result. As an inherent
problem, the rule is incapable of managing the high
conflicts from various information sources at the
step of normalization and may generate
counter-intuitive results as first highlighted by
Zadeh (1986). In the actual data processing, the
situation of evidence conflict is often encountered,
so it is necessary to try to avoid the errors caused by
the combination of conflicting evidence, otherwise
unpredictable consequences can be caused.
Therefore, it is an important research topic in this
field to study the method of combination of
conflicting evidence. By studying the improved
methods, they can be divided into two main
categories: One methodology is to modify
Dempster’s combination rule, which had more
satisfactory behaviour compared with Dempster’s
combination rule. The representative method is
Yager’s method(Yager, 1983). This method can be
also divided into two kinds, which are completely
reliable evidence and incompletely reliable evidence.
In the case of incomplete reliable evidence, the main
issue is how to allocate the conflict, including
conflict will be assigned to a subset of what
proportion. The unified belief function method
represented by Lefevre (2002) is essentially the
process of redistributing global conflicts. The above
methods, they are based on the closed framework,
when the recognition framework is not complete,
can not effectively deal with the conflict. Smets
(1990) believes that in an unknown environment
could not get a poor and complete recognition
framework, he put forward the concept of the open
framework, the transferable belief model, will be
part of the conflict assignment to the empty set. The
literature (Yager, 1987; Dubois, 1998) presented the
combination method in the open recognition
framework. But these methods only for the group
with the rest of the empty set reliability value for
processing, not considered in BBA generation will
be set into the system. On this basis, Deng Yong
(2004) systematically put forward the generalized
evidence theory. Scholars who put forward this
method believe that the cause of high conflict
evidence combination failure is due to some defects
178
Xu, S., Geng, J., Wei, S. and Wei, K.
Comparison of Typical DS Conflict Evidence Improved Algorithms.
In 3rd International Conference on Electromechanical Control Technology and Transportation (ICECTT 2018), pages 178-181
ISBN: 978-989-758-312-4
Copyright © 2018 by SCITEPRESS – Science and Technology Publications, Lda. All rights reserved