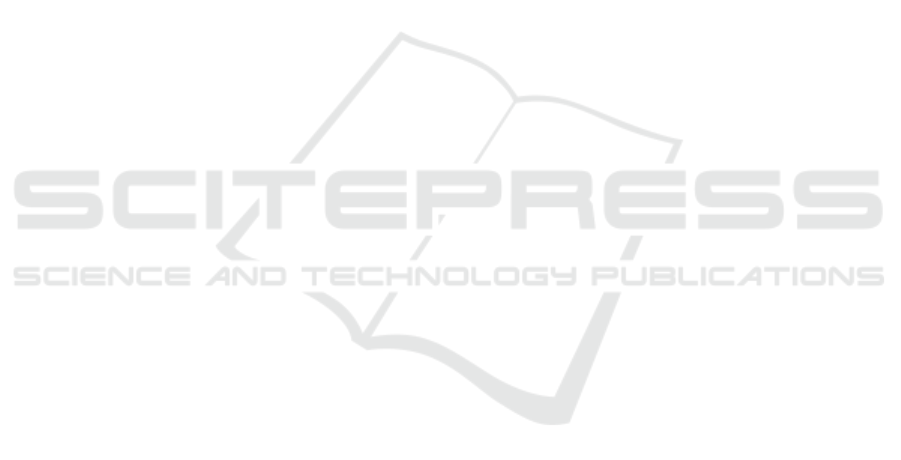
Hemmatian, F. and Karim Sohrabi, M. (2017). A survey on
classification techniques for opinion mining and sen-
timent analysis.
Hu, M. and Liu, B. (2004). Mining and summari-
zing customer reviews. In Proceedings of the Tenth
ACM SIGKDD International Conference on Know-
ledge Discovery and Data Mining, KDD ’04, pages
168–177, New York, NY, USA. ACM.
Huang, M., Qian, Q., and Zhu, X. (2017). Encoding syn-
tactic knowledge in neural networks for sentiment
classification. ACM Trans. Inf. Syst., 35(3):26:1–
26:27.
Jung, H., Park, H.-A., and Song, T.-M. (2017). Ontology-
based approach to social data sentiment analysis: De-
tection of adolescent depression signals. 19:e259.
Kalyani, Gupta, E., Rathee, G., Kumar, P., and Singh Chau-
han, D. (2015). Mood swing analyser: A dynamic
sentiment detection approach. 85:149–157.
Lee, D., Ju, H., Yu, H., Park, J.-m., and Kim, K.-Y. (2018).
Dualsentinet: Dual prediction of word and document
sentiments using shared word embedding. In Procee-
dings of the 12th International Conference on Ubiqui-
tous Information Management and Communication,
IMCOM ’18, pages 34:1–34:9, New York, NY, USA.
ACM.
Lin, B., Zampetti, F., Bavota, G., Di Penta, M., Lanza, M.,
and Oliveto, R. (2018). Sentiment analysis for soft-
ware engineering: How far can we go? In Procee-
dings of 40th International Conference on Software
Engineering.
Liu, B. (2012). Sentiment Analysis and Opinion Mining.
Morgan & Claypool Publishers.
Liu, B. (2015). Sentiment Analysis: Mining Opinions, Sen-
timents, and Emotions. Cambridge University Press.
Ma, Y., Peng, H., and Cambria, E. (2018). Targeted
aspect-based sentiment analysis via embedding com-
monsense knowledge into an attentive lstm. In AAAI.
Mantyla, M. V., Graziotin, D., and Kuutila, M. (2018). The
evolution of sentiment analysisa review of research to-
pics, venues, and top cited papers. Computer Science
Review, 27:16 – 32.
Missen, M. M. S., Boughanem, M., and Cabanac, G.
(2012). Opinion mining: reviewed from word to do-
cument level. Social Network Analysis and Mining,
3:107–125.
Muhammad, A., Wiratunga, N., and Lothian, R. (2016).
Contextual sentiment analysis for social media gen-
res. Knowledge-Based Systems, 108:92 – 101. New
Avenues in Knowledge Bases for Natural Language
Processing.
Musto, C., Semeraro, G., and Polignano, M. (2014). A
comparison of lexicon-based approaches for senti-
ment analysis of microblog. 1314:59–68.
Najar, D. and Mesfar, S. (2017). Opinion mining and senti-
ment analysis for arabic on-line texts: application on
the political domain. 20:1–11.
Pablos, A. G., Cuadros, M., and Rigau, G. (2017).
W2VLDA: almost unsupervised system for aspect ba-
sed sentiment analysis. CoRR, abs/1705.07687.
Peng, H., Ma, Y., Li, Y., and Cambria, E. (2018). Learning
multi-grained aspect target sequence for chinese sen-
timent analysis. Knowledge-Based Systems, 148:167
– 176.
Pham, D.-H. and Le, A.-C. (2018). Learning multiple
layers of knowledge representation for aspect based
sentiment analysis. Data & Knowledge Engineering,
114:26 – 39. Special Issue on Knowledge and Systems
Engineering (KSE 2016).
Rathan, M., Hulipalled, V., K R, V., and Patnaik, L. (2017).
Consumer insight mining: Aspect based twitter opi-
nion mining of mobile phone reviews.
Ravi, K. and Ravi, V. (2015). A survey on opinion mining
and sentiment analysis. Know.-Based Syst., 89(C):14–
46.
Shams, M. and Baraani-Dastjerdi, A. (2017). Enriched lda
(elda): Combination of latent dirichlet allocation with
word co-occurrence analysis for aspect extraction. Ex-
pert Systems with Applications, 80:136 – 146.
Studer, R., Benjamins, V., and Fensel, D. (1998). Know-
ledge engineering: Principles and methods. Data &
Knowledge Engineering, 25(1):161 – 197.
Sun, S., Luo, C., and Chen, J. (2017). A review of natu-
ral language processing techniques for opinion mining
systems. Information Fusion, 36:10 – 25.
Tang, D., Qin, B., Wei, F., Dong, L., Liu, T., and Zhou,
M. (2015). A joint segmentation and classification
framework for sentence level sentiment classification.
IEEE/ACM Transactions on Audio, Speech, and Lan-
guage Processing, 23(11):1750–1761.
Thakor, P. and Sasi, S. (2015). Ontology-based sentiment
analysis process for social media content. Procedia
Computer Science, 53:199 – 207. INNS Conference
on Big Data 2015 Program San Francisco, CA, USA
8-10 August 2015.
Tripathy, A., Agrawal, A., and Rath, S. K. (2016). Clas-
sification of sentiment reviews using n-gram machine
learning approach. Expert Systems with Applications,
57:117 – 126.
Turney, P. D. (2002). Thumbs up or thumbs down?: Se-
mantic orientation applied to unsupervised classifica-
tion of reviews. In Proceedings of the 40th Annual
Meeting on Association for Computational Linguis-
tics, ACL ’02, pages 417–424, Stroudsburg, PA, USA.
Association for Computational Linguistics.
Yang, B. and Cardie, C. (2014). Context-aware learning for
sentence-level sentiment analysis with posterior regu-
larization. In Proceedings of the 52nd Annual Meeting
of the Association for Computational Linguistics (Vo-
lume 1: Long Papers), pages 325–335. Association
for Computational Linguistics.
Zhang, L., Wang, S., and Liu, B. (2018). Deep learning for
sentiment analysis: A survey. Wiley Interdisciplinary
Reviews: Data Mining and Knowledge Discovery.
Sentiment Analysis Approaches based on Granularity Levels
331