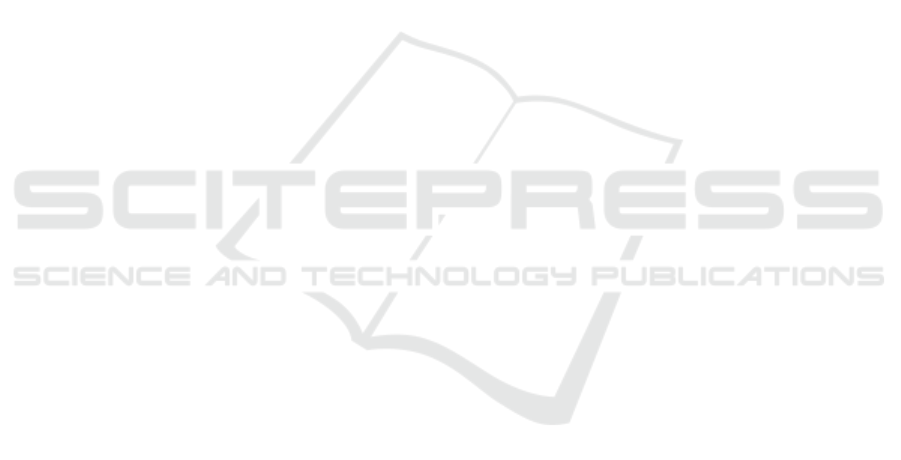
When multiple cameras are setup for instance in
an airport, it is possible to track the persons with
these features. From a forensic perspective the
likelihood ratio (LR) is used and can also be used to
combine evidence (Tripathi 2017, Haraksim 2014).
Current research is focused on how to estimate the
LR and how using this in practice on video. In court
one should be aware of possible pitfalls when using
this. (Dawid 2017, Morrison 2017)
Also the current research in facial biometrics is
aware of issues with facial recognition system as
well as experts. The combination of experts and AI
improves the quality of the results (Phillips P
Jonathon et al. 2018).
4 INTERNET OF THINGS
With the internet of things it is thought that all
devices of a person are connected to the internet and
communicate to each other. For instance the fridge
might order milk automatically if needed, Since we
have ipv6 the idea is that every device or object has
it own ip-address (An Overview of IPv6 2012). For
lawful data interception of the Internet of Things this
is ideal (Layer 3 Connectivity: IPv6 Technologies
for the IoT 2013) (Dihulia and Farooqui 2017),
however for privacy protection one should protect
the privacy of the user, and might consider privacy
enhancing technologies(Lee 2015).
The idea of all sensors around persons also
deviating events including a murder can be detected,
and more digital evidence can be collected after the
crime happened (Lund 2014). Ideally of course the
system would warn the police that a crime is going
to happen based on prediction models, to prevent the
crime from happening at all(Schaefer and Mazerolle
2017)
With the internet of things it is expected that data
is only temporary available due to the high amount.
One might think of a driverless car that processes
2000 Terbytes per year, however only limited data is
stored. For this reason live forensic investigation
should be researched further.
5 ANTI FORENSICS
Since people become more aware of digital traces, it
is also for privacy purposes necessary to wipe the
information or alter the information to mislead
investigation(Moon 2013, Moon 2015).
It is important in forensic casework to determine
if anti forensics tools have been used in a crime.
6 CONCLUSION AND
DISCUSSION
The field of digital evidence and forensic big data
analysis has grown quick in the last decades.
In the past most of the evidence was not
encrypted and easy to collect. Nowadays strong
encryption is used on the mobile phones, and the
cost to extract the information is higher.
Cloud storage still gives opportunities of
accessing the data by law enforcement. The real
challenge is processing multiple data source nearly
real time, and also use predictive tools to prevent
crime from happening. A good balance between
privacy and predictive policing is necessary if those
methods become feasible.
For digital investigation it is necessary in most
cases to do a triage of the information available,
since it is not feasible and economical to analyze all.
Digital forensic software can help in the analysis of
the traces, though one should know anti forensic
methods. Since software and hardware change, it is
important to cooperate internationally and do
continuous validation tests and research on new
development.
REFERENCES
An Overview of IPv6. 2012. IN: Linear and Nonlinear
Video and TV Applications: Using IPv6 and IPv6
Multicast. John Wiley & Sons, Inc., pp. 45–94.
Casey, E. 2011. Digital evidence and computer crime:
Forensic science, computers, and the internet.
Academic press.
Dawid, A.P. 2017. Forensic likelihood ratio: Statistical
problems and pitfalls. Science & justice : journal of
the Forensic Science Society, 57(1), pp.73–75.
Dihulia, S. and Farooqui, T. 2017. ITCP based Security
Enhancement for IoT Devices in IPV6 Protocol.
International Journal of Computer Applications,
178(2).
Geradts, Z. 2011. ENFSI Forensic IT Working group.
Digital Investigation, 8(2).
Gloe, T., Fischer, A. and Kirchner, M. 2014. Forensic
analysis of video file formats. Digital Investigation,
11.
Habibian, A., Mensink, T. and Snoek, C.G.M. 2017.
Video2vec Embeddings Recognize Events When
Examples Are Scarce. IEEE transactions on pattern