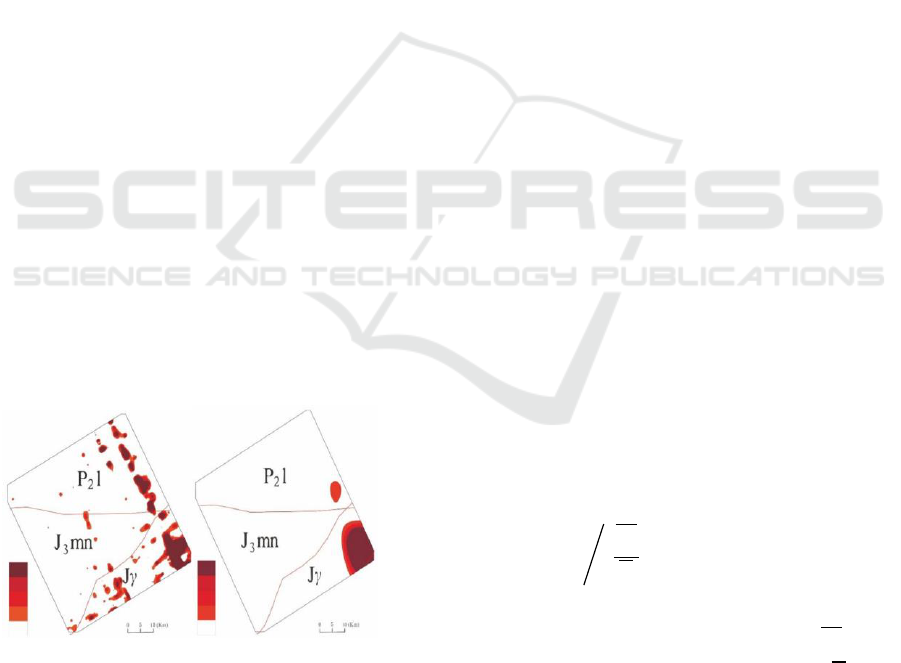
3.2 Extraction of Uranium Elemental
Anomaly Information
This paper uses 2-D wavelet transform method to
extract anomalies and compares it with the
traditional one. Because the airborne gamma-ray
spectrometry data is discrete, the frequently-used
wavelet basis functions that can be used for discrete
wavelet transform are Biorthogonal (biorNr.Nd),
Coiflets (coifN), Daubechies (dbN), Meyer (mey r)
and ReverseBior (rbioNr.Nd) (the functions in the
modules that contain wavelet basis functions in
MATLAB can directly be called). Use the above
five discrete wavelet basis functions to decompose
and reconstruct the data, respectively, and calculate
the CV of each processed data by formula (5). The
results are shown in Figure 4.
Figure 4 shows that when decomposition level is
the same, the CV of each data possessed by different
wavelet basis functions are quite different. The CV
of data processed by the same wavelet basis function
have a negative correlation with the decomposition
level at level 1-4, and when the decomposition level
is at 5, the CV of the data reaches the maximu m;
when the decomposition level is at 1-4, the change
rate of CV is s mall and the overall trend is relatively
stable, which show that wavelet decomposition has a
small impact on the whole data.
The author uses traditional statistical method and
2-D wavelet method (wavelet basis function is
bior6.8; deco mposition level is at 5) to extract
anomalies from the data and compare the extracted
anomalies. The results are shown in Figure 5.
Figure 5(a): Traditional method to extract the
anomaly area. (left)
Figure 5(b): 2-D wavelet to extract the anomaly area.
(right)
As shown in Figure 5(a), the ano malous
informat ion extracted by the traditional statistical
method has a large area, and the banded anomaly
interference in formation is obvious and scattered.
The reason is that the original data does not have an
approximately normal distribution, and the high-
value point data is missing during iterative reject
phase, resulting in the anomaly threshold value
being determined only by the background value.
The advantage of the 2-D wavelet method is that
it can co mbine the element content information of
survey points with the spatial position information;
there are two ore occurrences in the survey area, one
is lead-zinc ore with high U content and one is iron
ore with low U content. In Figure 5(b), there are
only two anomalies. The central location and
anomaly shape of the anomalous areas are consistent
with the actual ore occurrences, indicating that the
results do not contain false anomalies. Since the
low-frequency parts only contain ore-bearing and
background information, and the ore-bearing
informat ion are represented by high value ano malies.
Therefore, the ano maly a reas in the figure may be
ore-bearing areas.
3.3 Verification of Uranium Elemental
Anomaly Information
The paleo-uraniu m abundance refers to the uranium
content of an area in its beginning of diagenesis. In
the initial stage of diagenesis, U and Th have the
same chemical properties and no migration occurs ;
When the later environ ment beco mes an oxidation
environment, U migrates, while the chemical
properties of Th are relatively stable and remain in
place. The uran iu m ano maly areas indicated by
paleo-uraniu m abundance are source beds. Formula
6 is the fo rmula for the calculation o f paleo-uraniu m
abundance(Dai, 2002):
(6)
In formu la (6):
is the thoriu m content of a
certain survey point in the survey area;
is the
average thorium content in the survey area;
is the
average uranium content in the survey area.
Using formu la (6) to calculate the paleo-uraniu m
abundance in the survey area, the result is shown in
Figure 6.
At the beginning of diagenesis, uraniu m is
mainly concentrated in granite. The main lithology
IWEG 2018 - International Workshop on Environment and Geoscience
286