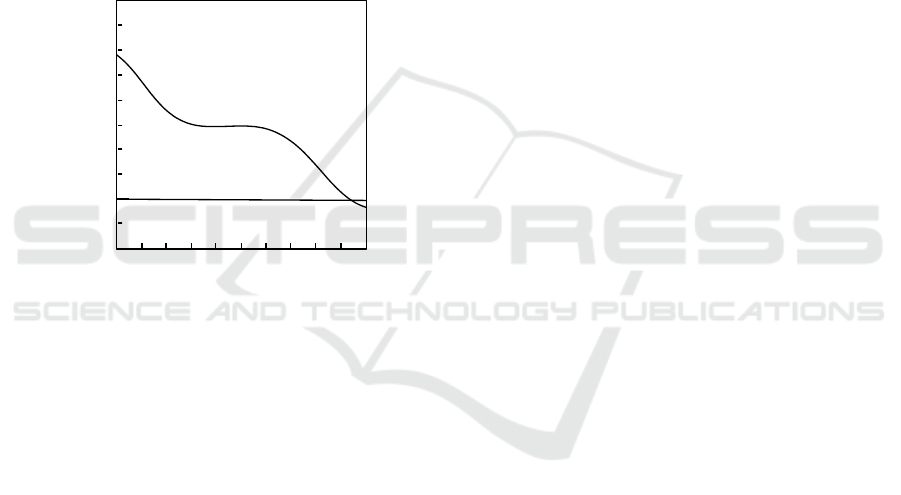
Fig. 3 is a fuzzy neural network prediction, the
function output corresponds to the discharge
capacity. In the sample size, the battery discharge
capacity can reach 100%. In order to facilitate the
observation error, the error curve is drawn by the
difference between the predicted output and the
expected output to predict the lithium battery fault
diagnosis. According to the prediction output , the
lithium battery fault could be diagnosed and the
result is credible.
After constructing the network, 150 training
samples are input for training. In order to avoid
over-learning, the training error precision is set, and
the learning process is stable and convergent. After
75 cycles, the allowable error range has been
reached. The BP network training error curve is
shown in Fig. 4.
Performance=0.004227
Goal=0.0015
Training Error
Iterations
Fig. 4 Training error curve.
5 CONCLUSION
Based on the complexity and uncertainty of
lithium battery faults in electric vehicles, this paper
proposes a lithium battery fault diagnosis method
based on fuzzy neural network. The method makes a
preliminary diagnosis of lithium battery fault
through neural network, and then uses the
combination rule to fuse different neural network
outputs, which can successfully diagnose the fault
state of the lithium battery, and the diagnostic
accuracy is higher than the single fault diagnosis
method, and the diagnosis is improved. Sexuality,
the result of the diagnosis is met, and the accurate
judgment of the fault state of the lithium battery of
the electric vehicle is obtained.
REFERENCES
1. WEI He,NICHOLAS williard,CHEN Chaochaoet
a1. State of Charge Estimation for Lion Batteries
Using Neural Network Modeling and Unscented
Kalman Filter-based Error Cancellation[J].
International Journal of Electrical Power and Energy
Systems,2014.
2. MATrHlEU D , LIAW B Y . Identify Capacity
Fading Mechanism in a Commercial LiFeP04
Cell[J].Journal of Power Source 2009,194:541-
549.
3. XU Jie.State of Charge Estimation Online Based on
EKF.An Method for Lithium.ion Power Battery[C]
2009 International Congress on Image and Signal
Processing(CISP 09),2009,11:1-5.
4. Steve R. Support vector machines for classification
and regression [D]. 2004,University of Southampton
5. Baker J.E.. Adaptive selection methods for genetic
algorithms[J], Proc. of the Intel Conf. on Genetic
Algorithms and Their Applications, 2008:101~111
6. ZHOU Meilan, ZHAO Qiang, ZHOU Yangqin. State
of Charge Estimation for LiFeP04 Cell Using
Improved PSO-BP Neural Network [J], Journal of
Harbin University of Science and Technology,
2015(4):88~92