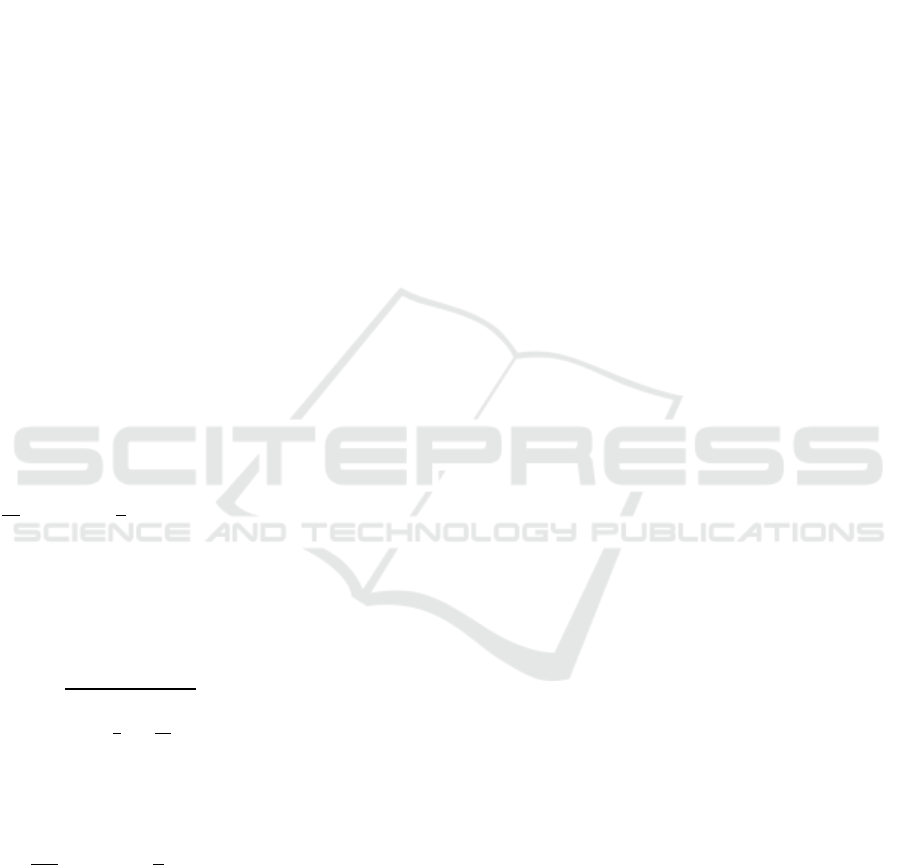
Implementation of Fractional Logistic Growth Model in Describing
Rooster Growth
Windarto, Eridani and Utami Dyah Purwati
Department of Mathematics, Faculty of Science and Technology, Universitas Airlangga, Surabaya, Indonesia
Keywords: fractional order, logistic growth model, particle swarm optimization method, rooster growth.
Abstract: Fractional order calculus was used in the study of viscoelastic medium (a medium with viscosity and elasticity
properties), image signal processing, and population growth modeling. In this paper, the fractional order of
logistic growth model was used to describe the dynamic growth of rooster, by which the rooster growth data
was cited from the literature. We also used the particle swarm optimization method to estimate parameters in
the fractional order logistic model. We found that the fractional order model is more accurate than the classical
logistic growth model in describing the rooster growth.
1 INTRODUCTION
Logistic growth model is widely used to describe a
life organism growth. The logistic growth of a single
species is governed by the following differential
equation.
(1)
Here represents the number of population of the
species at time and correspond to per capita
growth rate and carrying capacity respectively. If the
initial value
is positive, then analytical solution of
the logistic growth model in Eq. (1) given by Aggrey
(2002) and Windarto et al. (2014) is as follows.
(2)
where
The logistic growth ordinary differential equation
in Equation (1) has been generalized into the
fractional order logistic differential equation given by
El-Sayed et al. (2007).
(3)
Here, is fractional order where For
any positive initial value
, the exact solution of
fractional order logistic differential equations cannot
be determined. In this situation, heuristic method such
as simulated annealing, genetic algorithm and particle
swarm optimization method can
be applied to
estimate parameter values from the fractional order
logistic differential equation.
Particle swarm optimization is an optimization
method based on a population-based stochastic
(probabilistic) search process (Eberhart R. &
Kennedy, 1995; Kuo et al., 2011). Particle swarm
optimization method has been widely applied in many
areas, including performance improvement of
Artificial Neural Network (Salerno, 1997; Zhang et
al., 2000), scheduling problems (Koay and
Srinivasan, 2003; Weijun et al., 2004), traveling
salesman problems (Wang et al, 2003), vehicle
routing problems (Wu et al., 2004) and clustering
analysis (Kuo et al., 2011).
In this paper, particle swarm optimization method
was applied for predicting the parameters in fractional
logistic growth model. The remainder of this paper is
organized as follows. Section 2 briefly presents
particle swarm optimization method. Section 3
presents the implementation of fractional logistic
growth model for describing poultry growth. In
addition, parameters in the fractional logistic growth
was estimated by using particle swarm optimization
method. Finally, conclusions are presented in Section
4.
Windarto, ., Eridani, . and Purwati, U.
Implementation of Fractional Logistic Growth Model in Describing Rooster Growth.
DOI: 10.5220/0007547505830586
In Proceedings of the 2nd International Conference Postgraduate School (ICPS 2018), pages 583-586
ISBN: 978-989-758-348-3
Copyright
c
2018 by SCITEPRESS – Science and Technology Publications, Lda. All rights reserved
583