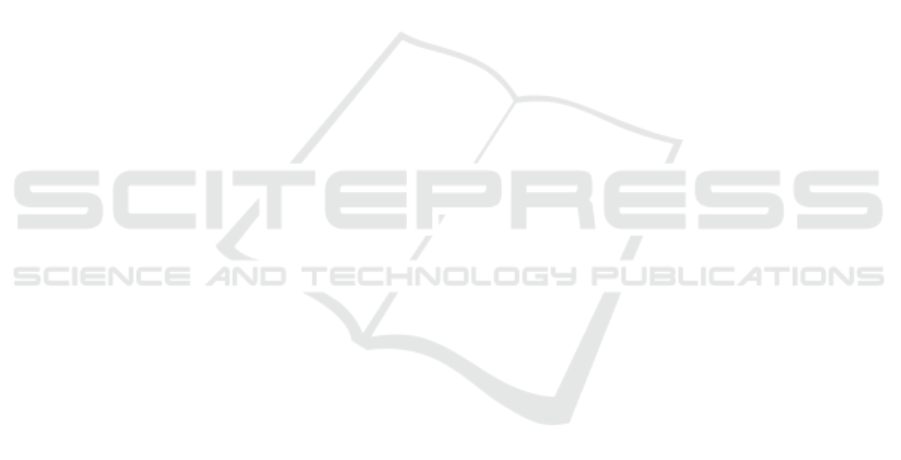
4.3. Multi-target evaluation coupling model
Once the indexes were ranked and weighted, a number of individual indexes were compiled into a
multi-target model. In this study, an analytic hierarchy process (AHP) and structural equation model
(SEM) were applied to compile the indexes[3].
4.3.1. Analytic hierarchy process. The overall objective of the AHP is to utilize the calculated
indexes to perform a comprehensive evaluation. The process is defined as follows: first, the research
objective is divided into several analysis criteria, i.e., reservoir sedimentation, sediment flow
transport, backward erosion and tributary backflow. Next, the importance of each individual criterion
was determined through use of the secondary indexes. Expert rankings were used to determine the
index weights, as mentioned in section 4.2. The corresponding evaluation system R
I
is acquired by
comparing simulation results using simulated results and measured data. Through corresponding
weight matrix, the second-level indexes are weighted statistically processed and A
I
is calculated:
A
I
=B
i
*R
I
(1)
Where R
I
is the expert scores of second-level indexes, B
i
is weight matrix of layer P
i
~ P
ij
. The
value of E can be acquired by coupling B and A. Based on the overall evaluation and the ranking of
different indexes, the evaluation result under different objectives can be acquired.
E=B*A (2)
This process combines statistical and error analysis theory with expert index rankings to create a
weighted index matrix. Once the weighted index matrix is established, the weighted treatment of
qualitative index fuzzy quantification method is used to establish the membership function to
describe the differences and connections of each index, which can better resolve the relevance and
ambiguity of comprehensive evaluation.
4.3.2. Structural equation modelling. Structural equation modelling is a recently developed statistical
modeling method. It is particularly suitable for factors that are more subjective and difficult to
quantify, such as model comprehensive performance, visualization effects, and evaluating
curve-fitting processes. First, the structural equation for the comprehensive model evaluation is
constructed. Applying the structural equation modelling method includes five main steps: model
construction, fitting, evaluation, correction and application. This model combines measurable flow
and sand observation variables with potential variables that are difficult to measure. Thus, a
multi-objective evaluation method for decision-making is constructed. Variables which are difficult
to directly measured are terms “latent variables”. In this assessment, parameters such as reservoir
siltation, sediment transport, backward erosion, and tributary backflow are used as the primary
assessment criteria. Currently, there are no direct methods to measure these criteria. Thus, observable
variables must be utilized to quantify them. In SEM, “observed variables” are variables that can be
quantified using measured data, such as mass of sediment released from the reservoir, deposition
thickness, etc[4].
The SEM evaluation is constructed with the following system of equations:
(3)
Where denotes the impact of the latent and observational variable matrices on the final evaluation.
The matrix elements were weighted and random error was minimized. The definition of equation
parameters and variable names are shown in Table 4.
IWEMSE 2018 - International Workshop on Environmental Management, Science and Engineering
130