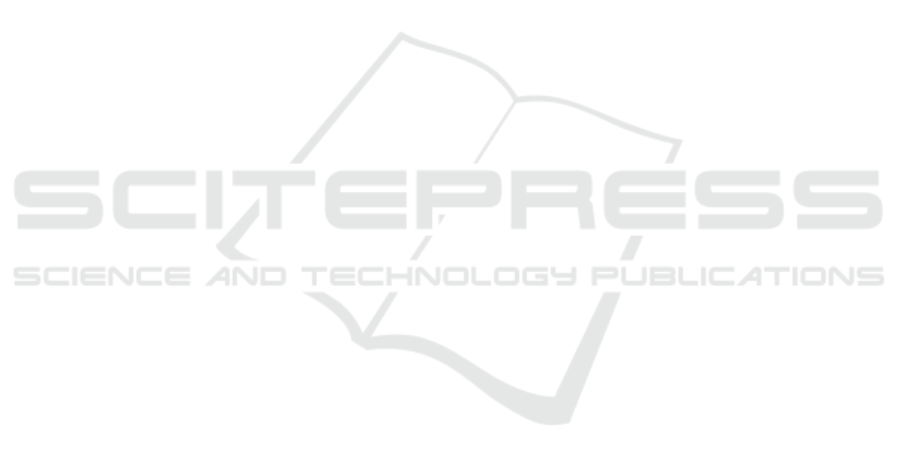
3.3. Discussion
Above all, we get a result of mean accuracy 82.85% for the forecasting of appliances with hourly
data by using supervised AFHMM model, which is more accurate than other similar analyses.
Czarnek N. [16] compares the results for some public datasets with frequency of 1/s and 1/min by
several models, such as KNN, random forest, linear support vector machine and SVM with radial
kernel, where the accuracy ranges from 8% to 92%. Where only the accuracies of AMPds data
obtained by KNN and random forest models are larger than our work, but the data frequency is 1/min,
which is too large to be widely shared. Kolter J. Z [17] analyses the hourly Plugwise data with
supervised sparse coding model, but he only got a result with 55% accuracy. For the appliances,
lighting and movement have almost 90% accuracy, while for air conditioner and management, the
accuracy is only about 65% because of large fluctuation, meaning that we need improve the
performance of model with more attention on the usage of air conditioner and management. The
model in this work uses both data of appliances and aggregation for training, but in forecasting only
aggregation and influence factors data are used, which is possible to be applied in buildings without
appliance collectors only if we include the information of buildings in the training model.
4. Conclusions
In this paper, a supervised additive factorial hidden Markov model is developed by including
exogenous influences to forecast the electricity usage in appliance level. The model adopts EM
method to train the training dataset and obtain optimized parameters for submodel of each appliance
with both disaggregate and aggregate electricity data, and exogenous influence data. While in testing
process, the fitted parameters of submodels and exogenous influence data are used to forecast the
usage of appliances, and the aggregate electricity is used to adjust the values. The accuracy of the
model in training and testing dataset is calculated by comparing the estimated and measured values.
By applying the model to a dataset of one year hourly electricity consumption data for five types of
appliances in one building collected by smart meters in Shanghai, we get optimized parameters for
submodels with first 7,000 values as training set and forecast the appliances usage with last values as
testing set. The performance of model on training set is perfect with relative errors smaller than 7%
except for the usage of others. The performances of model in final forecasting results differ a lot for
different appliances, where the relative errors are 12.46%, 10.52%, 37.53%, 32.02%, 32.25% for the
usage of lighting, movement, air conditioner, management and others, respectively. The performance
of lighting and movement is best, while that of air conditioner is worst because of the large
randomness and fluctuation of usage in air conditioner for the diversity of human behaviors. We need
to pay more attention on the behaviour analysis on the usage of air conditioner to improve the
performance of model. The mean accuracy of all appliances is 82.85%, which is much higher than
analyses with same frequency data, and even larger than most analyses with higher frequency data.
The model proposed in this paper can forecast the usage of main appliances in hourly frequency
perfectly. In the future, we need to collect data of more types of buildings and include more influence
factors to extend the model and optimize the parameters of the model, in order to forecast the usage
of appliances for buildings without sub-collectors. The information about usage of appliances
feedback to users and energy suppliers can improve the efficiencies of energy-saving and monitoring,
which is a good way to overcome environmental pollution and shortages of resources.
Acknowledgment
This research is supported by the National 863 High Technology Research and Development Program of
China (2015AA050203) and the Science Project of State Grid Shanghai Municipal Electric Power
Company (52094016001Z).
References
[1] Kazandjieva and et al 2010 Technical Report CSTR 2010-03. Stanford University
Forecasting Electricity Consumption of Appliances based on the Additive Factor Hidden Markov Model
199