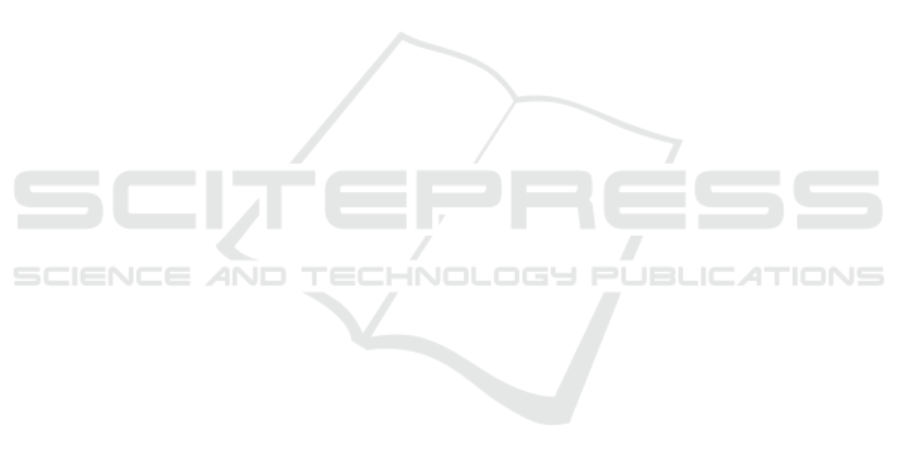
collection‘ from Anqing data set, which indicates that respondents across the two cities, in general,
are concerned about the improvement of attribute levels and a relatively reasonable experimental
design we have. The model fit of model 2 for each data set is improved by incorporating the
interaction terms. Model 2 is highly consistent with model 1 in terms of signs and significance of
attribute coefficients. It reveals that assuming other factors remain constant, improving the levels of
‗waste classification‘, ‗frequency of waste collection‘ and ‗frequency of waste transportation‘ would
increase respondents‘ utility while increasing the fee would decrease their utility. But respondents
from different data sets have different preferences for attributes, e.g., respondents in Nanchang tend
to focus on ‗waste classification‘ and ‗frequency of waste transportation‘ while respondents in
Anqing are inclined to ‗frequency of waste collection‘. It should be noted that the coefficient on
‗frequency of waste collection‘ has the largest value but is not significantly different from zero at the
10% level in Anqing data set, and its accuracy and reliability deserve the following further study.
The interaction terms have different signs and significance across the two data sets. Model 2 for
Nanchang manifests respondents who have higher household income, more environmental
knowledge, higher awareness of environmental protection, or more positive subjective perception of
the environmental behaviors of their neighbors are willing to pay more for improving MSWM
services, ceteris paribus. As to Anqing data set, education, household income, environmental
awareness, and subjective perception of the environmental behaviors of neighbors have significantly
improved households‘ willingness to contribute money. These findings are consistent with previous
studies [20, 21, 31, 32]. However, older people in Anqing are more reluctant to pay, which is
intuitively correct since older people have low level of environmental awareness and are more
conservative in spending in China.
Despite widely used, MNL model has severe limitations with respect to the well-known
assumption of independence of irrelevant alternatives and its ability to capture random taste
heterogeneity across individuals [33, 34]. Hence, we will move to apply a less restrictive and more
flexible model to the following analysis.
3.2.2. The results of random parameter logit (RPL) model. As RPL model tends to be unstable and
identification issues arise when all coefficients vary over the population, the coefficient on fee is
fixed while other coefficients are allowed to vary in our analysis (see Goett et al., Revelt and Train)
[35, 36]. Considering some respondents may be satisfied with the status quo, e.g., respondents need
not have to spend time and energy on waste separation nor have to pay extra money, we specify the
coefficients on ‗waste classification‘, ‗frequency of waste collection‘ and ‗frequency of waste
transportation‘ to be normally distributed. Attributes showing insignificant standard deviation are
then respecified as nonrandom parameters. At last, ‗frequency of waste collection‘ and ‗frequency of
waste transportation‘ are specified as random parameters and other variables remain nonrandom
parameters in estimation models for the two data sets. To identify the potential sources of
heterogeneity, random parameters are interacted with socio-economic and attitudinal variables.
Initially, all possible interactions are put into estimation model for each city and finally only
interactions that are significant at the 10% level or less are retained. The estimation results are shown
in Table 5.
The estimation of RPL model results in considerable improvement of model fit over the MNL
model. All parameter estimates related to the attributes and ASC are statistically significant and have
the expected signs including the ‗frequency of waste collection‘ for Anqing data set, on which the
coefficient is insignificant in the MNL model. The ASC and ‗waste classification‘ are nonrandom
parameters for the two cities, implying that respondents generally agree to improve the services of
MSWM at the expense of extra spending and waste separation, which is out of our expectation. One
possible explanation is that, along with the increasing negative impacts of waste on residents and
Estimating Willingness to Pay for Improving Municipal Solid Waste Management Using a Choice Experiment: A Case Study of Central
China
387