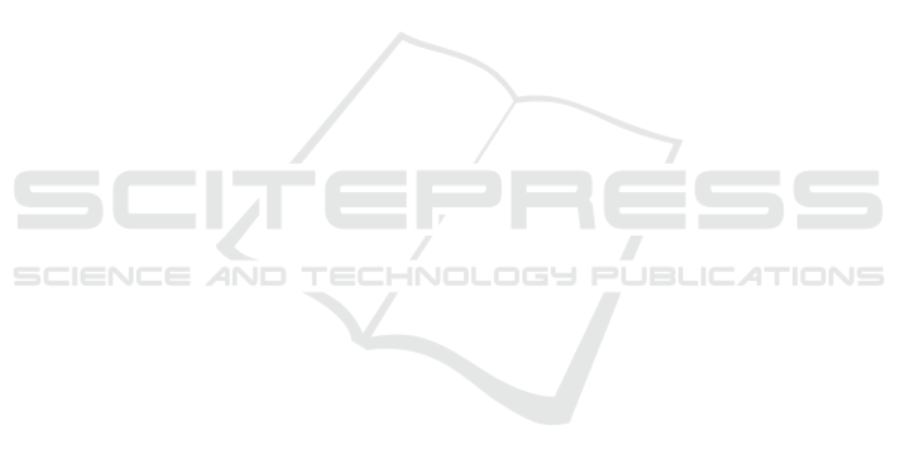
plant disease degree [9]. Cai, et al. used image texture features to classify soil samples with different
degrees of salinization, and a higher correct classification rate has been obtained [10]. They
considered that when the soil samples were similar in spectral features, texture features would play a
positive role in the sample recognition, and combined the information of spectral and texture features
can help to improve the classification accuracy. Ma, et al. using HSI technique to distinguish healthy,
greening disease infected and zinc-deficient citrus [9]. As the leaf spectra of greening disease
infected and zinc-deficient citrus were partially overlapped, and the leaf texture features of greening
disease infected and zinc-deficient citrus were similar, utilization of spectral information or texture
features for modelling cannot achieve good classification results. However, data fusion combining
spectral information and texture features greatly improved the correct classification rate for the three
kinds of citrus. To our knowledge, comprehensive utilization of spectral information and image
texture features for the classification of soil types was seldom reported.
Hyperspectral image generates an immense amount of data. Some of them may contribute more
co-linearity, redundancies, and noise than relevant information to calibration models, which is a huge
challenge for the application of HSI technique [11]. Effective wavelength selection, aiming to select
only a few wavelengths which carry the most of useful information with minimum collinearity and
redundancy from full spectrum, is believed to reduce amount of data, computational task, and build a
simple and robust model [12, 13]. Successive projections algorithm (SPA) is a popular tool for
wavelength selection in multivariate calibration and classification [14]. It is able to select a small
representative set of spectral wavelengths with a minimum of collinearity. In machine visual systems,
the most popular method for texture feature analysis is Gray level co-occurrence matrix (GLCM)
method [15]. GLCM, created through calculating how often a pixel with a particular gray level value
occurs at a specified distance and angle from its adjacent pixels, is able to take into account the
specific position of a pixel relative to another. In this work, SPA and GLCM were adopted to select
effective wavelengths and extract texture features, respectively.
The objective of this work was to investigate the feasibility of classifying soil types using HSI
technique. The specific objective was to build classification models for soil types in utilization of
spectral information and image texture features.
2. Materials and methods
2.1. Soil samples and laboratory reference measurement
Total 183 soil samples sampled from the upper soil layer (0-30 cm) of Shangyu City, Zhejiang
province, People’s Republic of China, were used in this study. All the samples were air dried and
sieved with a diameter of 1 mm. Then they were air dried again at 60°C for 48 h. A small portion of
each sample was sent to the agricultural testing center of Zhejiang Provincial Academy of
Agricultural Sciences (ZPAAS) for soil classification analyses. The remaining samples were used for
HSI measurement.
According to the classification and codes for Chinese soil (National standard of China,GB/T
17296–2009), the soil samples belonged to three major soil types of this area, namely, paddy soil (84
samples), red soil (57 samples) and seashore saline soil (42 samples).
2.2. Hyperspectral image acquisition
The hyperspectral images of soil samples were captured by a near-infrared HSI system with the
wavelength range of 874-1734 nm and 256 bands. The system was composed of an imaging
spectrograph (ImSpector N17E; Spectral Imaging Ltd., Oulu, Finland), a CCD camera (Xeva 992;
Xenics Infrared Solutions, Leuven, Belgium), two 150W quartz tungsten halogen lamps (Fiber-Lite
DC950 Illuminator, Dolan Jenner Industries Inc., USA), and a conveyer belt which was driven by a
stepper motor for sample movement (Figure 1). The entire system was fixed in a darkroom. The soil
Classification of Soil Types Using Hyperspectral Imaging Technology
503