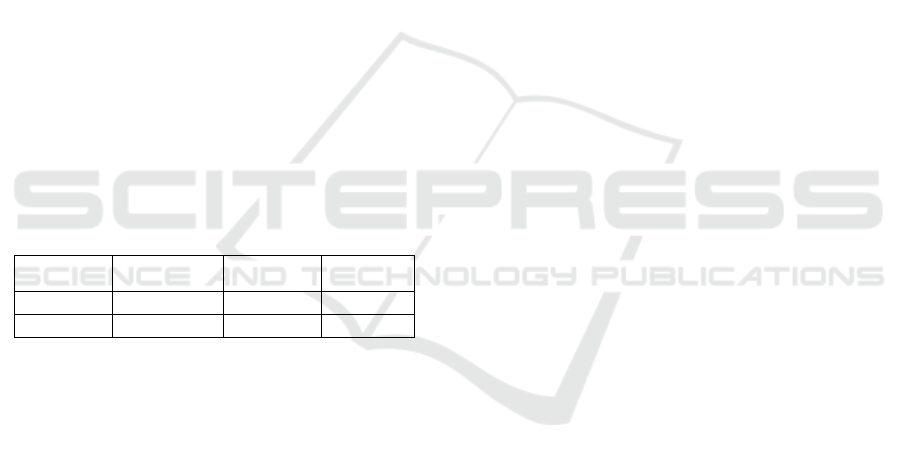
selected from all training samples. The remaining 1
sample is tested, and the average error of the l
prediction is taken as the objective function of the
optimization, so that the model is added to the model
generalization ability. Therefore, the prediction
accuracy of inulinase concentration for unknown
samples can be significantly improved by the LOO-
PSO method, which is the reason why it takes longer
time to find the optimal results than PSO method.
4.2 Comparison of SVM Regression
Soft-sensor
This section mainly compares the prediction effect
of LS-SVM regression soft-sensor and standard
SVM regression soft-sensor.
The parameter optimization effect of LOO-PSO
algorithm has been proved before, so in order to
guarantee the rationality of the contrast, in this
section, the LOO-PSO parameter optimization
method is adopted for the two soft measurement
models. The PSO parameter setting is the same as
that of the 4.1 section. See Table 1 for details.
The predictive effect of inulinase concentration
in standard SVM and LS-SVM regression soft-
sensor is shown in Table 3.
Table 3: Comparison of different soft-sensors based on
support vector machines.
It can be seen from the previous table that there
are not significant difference between standard SVM
model and LS-SVM model for the inulinase
concentration estimation results at the same LOO-
PSO parameter condition. But the prediction effect
of the LS-SVM regression soft-sensor is better than
the standard SVM regression soft-sensor.
5 CONCLUSIONS
According to the type of input variables and the
amount of samples, a soft-sensor is established to
predict inulinase concentration during the Pichia
pastoris fermentation process based on LS-SVM
regression. The prediction effect of LS-SVM soft-
sensor is better than the standard SVM regression
soft-sensor for unknown inulinase concentration.
To find the best parameters of LS-SVM soft
measurement model, this paper proposed LOO-PSO
parameter optimization method combined with PSO
and LOO method. By comparing the experimental
results with the GS method and the PSO, it is found
that the proposed LOO-PSO parameter optimization
method has the advantages of fast convergence
speed and high prediction precision in predicting the
accuracy of inulinase concentration of unknown
samples.
The soft-sensor can provide references and
guidance for the implementation of real-time control
strategy and improvement of fermentation
technique. Since fermentation process has the
character of shift and variety with time, the soft-
sensor should consider adjusting online to fit new
condition in the future.
ACKNOWLEDGEMENTS
Financial support for this work was provided by the
Key Research and Development Program of
Shandong Provence (No.2016ZDJS02B02).
REFERENCES
Aazi, F. Z., Abdesselam, R., Achchab, B., Elouardighi, A.,
2016. Feature selection for multiclass support vector
machines [J]. AI Communications, 29(5): 583-593.
Bogaerts, P., Wouwer, A. V., 2003. Software sensors for
bioprocesses[J]. ISA transactions, 42(4): 547-558.
Chapelle, O., Vapnik, V., Bousquet, O. et al., 2002.
Choosing multiple parameters for support vector
machines[J]. Machine learning, 46(1-3): 131-159.
Cortes, C., Vapnik, V., 1995. Support-vector networks[J].
Machine learning, 20(3): 273-297.
Huang, C. L., Wang, C. J., 2006. A GA-based feature
selection and parameters optimization for support
vector machines[J]. Expert Systems with applications,
31(2): 231-240.
Lin, S. W., Ying, K. C., Chen, S. C. et al., 2008. Particle
swarm optimization for parameter determination and
feature selection of support vector machines[J]. Expert
systems with applications, 35(4): 1817-1824.
Suykens, J. a. K., Vandewalle, J., 1999. Least Squares
Support Vector Machine Classifiers[J]. Neural
Processing Letters, 9(3): 293-300.
Xi, M. L., Sun, J., Liu, L. et al., 2016. Cancer Feature
Selection and Classification Using a Binary Quantum-
Behaved Particle Swarm Optimization and Support
Vector Machine[J]. Computational and Mathematical
Methods in Medicine, 10(115):9.
Zhang, L., Zhao, C., Zhu, D. et al., 2004. Purification and
characterization of inulinase from Aspergillus niger
AF10 expressed in Pichia pastoris[J]. Protein
expression and purification, 35(2): 272-275.
MEEP 2018 - The Second International Conference on Materials Chemistry and Environmental Protection
18