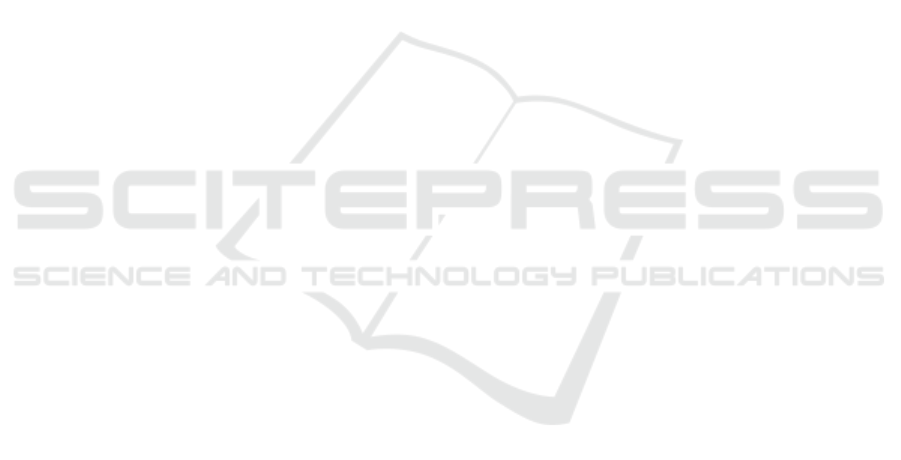
attractiveness of the city and housing market prices.
Mundula and Auci (2013) verified the robustness of
the city smartness indicators proposed by Giffinger et
al. (2007) in explaining the efficiency of cities in
Europe. Meanwhile, Caragliu et al. (2011) conducted
a study with the same purpose as this present study,
by analysing the effect of the application of the smart
city concept on the economic performance of the city.
Although having similar objectives to the study
conducted by Caragliu et al. (2011), the use of
different methods and variables in this study are novel
in this area of research. A study in Indonesia carried
out by Ramadhan (2017) aimed to rank the results of
smart city implementation in metropolitan cities in
Indonesia using the analytical hierarchy process
method. This differs from the present study which
aims to discover the success of smart city concept
implementation by looking at the relationship
between the application of the concept and the
economic performance of cities and districts in
Indonesia, both those that have and have not
implemented the smart city concept, and not solely
metropolitan cities. The study period of 2012 to 2016
is also longer than previous studies, and in each year
cities that have not and have applied the concept of
‘smartness’ are researched, while the research
conducted by Ramadhan (2017) only looked at the
year 2015.
In this study, the city smartness indicators used to
analyse the concepts and definitions of the smart city
are the concepts proposed by Giffinger (2007),
namely smart economy, smart people, smart
governance, smart environment, smart mobility and
smart living. This set of urban qualities based on the
characteristics of ‘smartness’ proposed by Giffinger
et al. (2007) can explain the differences in economic
performance of each city. Furthermore, the
application of smartness concepts is adjusted to the
conditions of cities in Indonesia.
Each city has different characteristics and smart
city programme implementations, but their goals are,
of course, the same: to improve the performance of
the city in solving urban problems effectively and
efficiently to create improved welfare and quality of
life in urban areas. Economic performance can be
described through the per capita gross domestic
regional product (GDRP) of each city. Based on data
from the Central Bureau of Statistics (2018),
Indonesia's economy in 2017, measured by per capita
GDP, reached Rp 51.89 million or US$3,876.8,
which means that Indonesia’s economy in 2017 grew
5.07 per cent, a higher rate than the 2016 achievement
of 5.03 per cent. In terms of production, the highest
growth, of 9.81 per cent, was achieved in the
information and communication business sector. This
coincides with the increase in the number of cities
implementing the smart city concept since the
movement towards 100 Smart Cities programme was
launched in 2017. It is therefore necessary to examine
the influence of the application of the smart city
concept on the economic performance of cities in
Indonesia. The results prove that the implementation
of the smart city concept in districts or cities in
Indonesia has a significant positive effect on their
economic performance.
This paper is organized as follows: the first
section provides an overview of the importance of the
research and its novel aspects. The second offers
theoretical background and empirical studies from the
subject literature. The third section describes the data
and variables construction along with empirical
modelling and related estimation issues. The fourth
section provides results and analysis and the final
section provides our conclusions.
2 LITERATURE REVIEW
The concept of the smart city typically
emphasizes the use of technology and the internet.
Several definitions of the smart city have been put
forward, one of which is that, according to Giffinger
et al. (2007) a smart city is one with good
performance and a forward-looking attitude, and
which displays six characteristics (or dimensions)
built from a combination of ‘smartness’,
independence and public awareness. The ‘smart’
dimensions proposed by Giffinger et al. (2007) are
smart economy, smart people, smart governance,
smart mobility, smart environment and smart living.
In addition to these six dimensions, Nam and Pardo
(2011) developed a further three dimensions of the
smart city, including technology (hardware and
software infrastructure), population (creativity,
diversity and education), and institutions
(government and policy). From these various
dimensions it can be seen that technology and the
internet are not only the goals of smart cities; rather,
they are also tools that support the application of the
smart city concept. According to Caragliu et al.
(2011), a city becomes smart when it invests in
human capital, social aspects, transportation and
information and communication technology so as to
encourage sustainable economic growth and a high
quality of life, combined with wise natural resource
management and government participation.
Therefore, the label ‘smart city’ should indicate the
delivery of smart solutions which allow cities to
Economic Performance of Cities in Indonesia: Impact Analysis of Smart City Concept Implementation
123