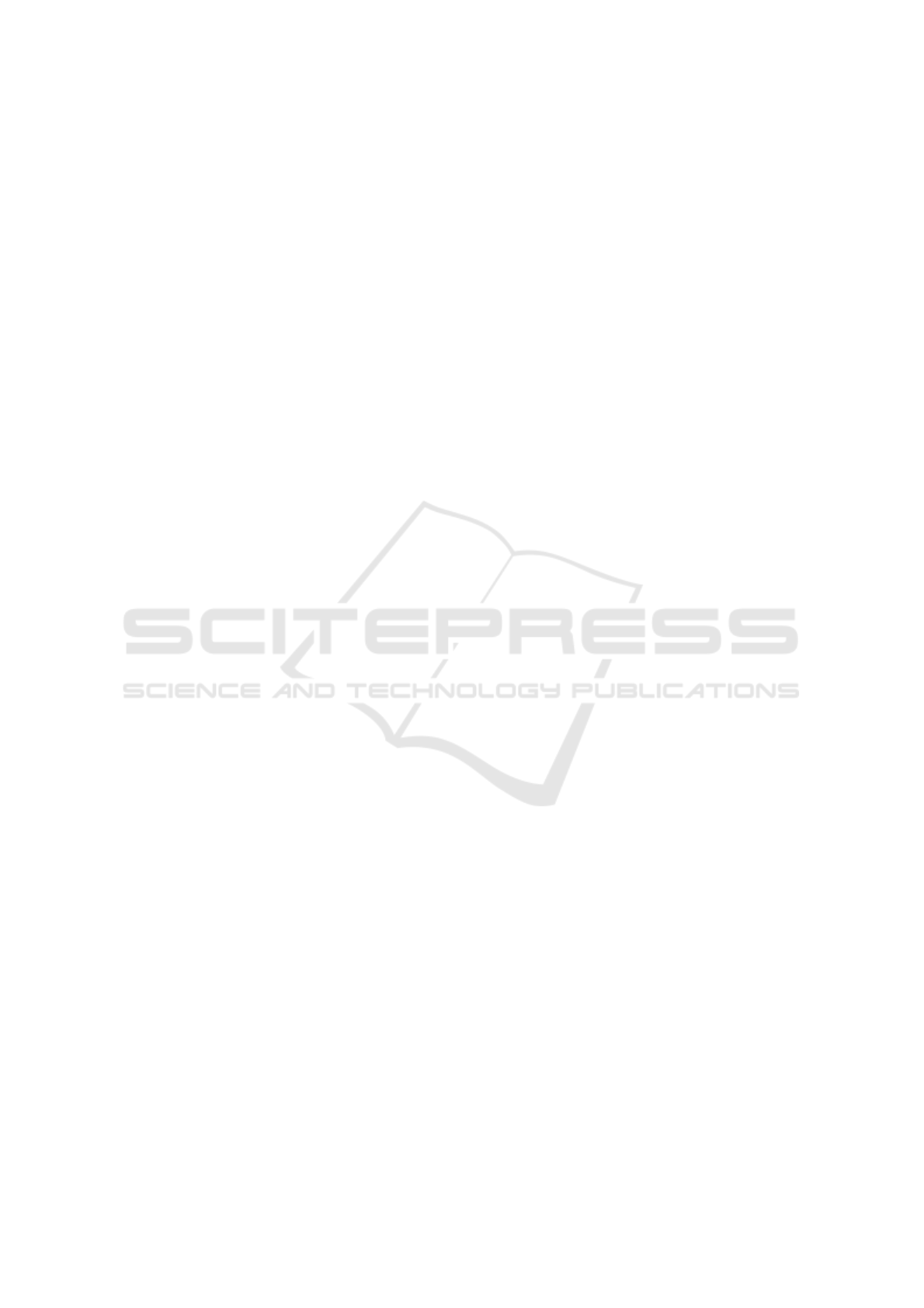
Iqbal Munawar (2001), Islamic and Conventional Banking
in the Nineties: A Comparative Study, Journal of
Islamic Economic Studies, Vol.8, No.2.
Jaizah Othman & Mehmet Asutay (2017), Integrated early
warning prediction model for Islamic: the Malaysian
case, J Bank Regul, DOI 10.1057/s41261-017-004005,
Macmillan Publisher Ltd
Jaizah Otman (2013), Analysing Financial Distress in
Malaysian Islamic Banks: Exploring Integrative
Predictive Methods, Durham theses, Durham
University.
James S Sagner, (2014), Working Capital Management –
Application and Cases, John John Wiley & Sons, Inc.,
ISBN 978-1-118-93383-1 (cloth); ISBN 978-1-118-
93384-8 (epub); 978-1-118-93385-5 (epdf).
Jonathan Berk, Peter DeMarzo, Jarrad Harford, (2012),
Fundamentals of Corporate Finance, 2nd Edition,
Prentice Hall, ISBN 978-0-13-214823-8
Kahya, E. & Theodossiou, P. (1996), Predicting Corporate
Financial Distress: A Time-Series CUSUM
Methodology, Review of Quantitative Finance &
Accounting, 13,71-93
Keasey, K. & Watson, R. (1991), The State of the Art of
Small Firm Failure Prediction: Achievements and
Prognosis, International Small Business Journal, 9(4),
11.
Kim, M. H. & Partington, G. (2014). Dynamic forecasts of
financial distress of Australian firms, Australian
Journal of Management, 40 (1), 135-160
Knauer, T & Wöhrmann, A. (2013), Working capital
management and firm profitability, Journal of
Management Control, Vol. 24 No. 1, pp. 77-87.
Konstantaras, Konstantinos and Siriopoulos, Costas,
(2011), Estimating financial distress with a dynamic
model: Evidence from family-owned enterprises in a
small open economy, Journal of Multinational
Financial Management, 21, issue 4, p. 239-255
Kpodoh, B. (2009), Bankruptcy and Financial Distress
Prediction in the Mobile Telecom Industry: The case of
MTN – Ghana, Millicom – Ghana and Ghana
Telecom, School of Management, Blekinge Institute of
Technology
Lemma W. Senbet dan Tracy Yue Wang (2012), Corporate
Financial Distress and Bankruptcy: A Survey,
Foundations, and Trends in Finance
M. Adnan Aziz, Humayon A. Dar, (2006), Predicting
corporate bankruptcy: where we stand?, Corporate
Governance: The international journal of business in
society, Vol. 6 Issue: 1, pp.18-33
Marcos Antônio de Camargos, Mirela Castro Santos
Camargos, Luciano de Castro Garcia Leão, (2014),
Empirically Testing The "Flueriet's Model": Evidence
of Bazilian Market, Journal of Business and
Management Review ISSN: 2047 – 0398
Marttonen-Arola, Salla, Viskari, Sari and Kärri, Timo
(2013), The missing link between maintenance
contracts and flexible asset management.
International, Journal of Procurement Management, 6
(6). pp. 649-665
McLeay, S. & Omar, A. (2000), The Sensitivity of
Prediction Models to the Non-Normality of Bounded
and Unbounded Financial Ratios, The British
Accounting Review, 32(2), 213-230.
Michael C. Jensen and Clifford W. Smith, Jr, (1984), The
Modern Theory of Corporate Finance, Editors, (New
York: McGraw-Hill Inc., 1984) pp. 2-20.
Mikhail Smirnov, (2012), Dynamic Leverage as a
Generalization of Traditional Value- At-Risk Measures
and its Use in Portfolio Construction, Columbia
University, SSRN-id2173865
Miranda GJ and Silva Duarte, (2016), Indicators of
Working Capital Management before and after the
adoption of the IAS in Brazil, Journal of Education and
Research in Accounting, ISSN: 1981-8610
Modigliani, Franco, and Miller, Merton H., (1958), The
Cost of Capital, Corporate Finance, and the Theory of
Investment, the American Economic Review, 48/3, 261
– 297.
Modigliani, Franco, and Miller, Merton H., (1963), The
Cost of Capital, Corporate Income Taxes and the Cost
of Capital: A Correction, the American Economic
Review, 53/3, 433 – 443
Muller GH, Steyn-Bruwer B.W & Hamman W.D, (2012),
What is the best way to predict financial distress of
companies, An Article
Muller, G. H.; Steyn-Bruwer, B. W. & Hamman, W. D.
(2009), Predicting Financial Distress of Companies
Listed on the JSE - A Comparison of Techniques,
South African Journal of Business Management,
40(1), 21-32.
Myers, S., (1984), The Capital Structure Puzzle, Journal of
Finance, Vol. 39.
Ohlson, J. A. (1980), Financial Ratios and the
Probabilistic Prediction of Bankruptcy, Journal of
Accounting Research, 18(1), 109-131.
Otoritas Jasa Keuangan, (2017), Indonesia Sharia
Financial Development Roadmap 2017-2019,
Financial Services Authority.
Oyelere, P., Kuruppu, N. & Laswad, F. (2003), The
Efficacy of Liquidation and Bankruptcy Prediction
Models for Assessing Going Concern, Managerial
Auditing Journal, 18(6/7),
Pappas Vasileios and Izzeldin Marwan (2011),
Determinants of Survival in Islamic Banks, Dept of
Economics, Lancaster University Management School.
Pappas Vasileios, Izzeldin Marwan and Fuertes Maria-Ana
(2012), Failure Risk in Islamic and Conventional,
Lancaster University Management School, UK.
Peirson, G. (2006), Business Finance, 9th Edition.
McGraw-Hill Australia Pty Limited.
Peter De Marzo and Zhiguo He, (2016), Leverage
Dynamics without Commitment, Stanford University,
Graduate School of Business, and NBER
Platt, H. D. & Platt, M. B. (2002), “predicting Corporate
Financial Distress: Reflections on Choice-based
Sample Bias, Journal of Economics and Finance,
26(2), 184-199.
An Early Warning Model of Financial Distress Sharia Banks in Indonesia
731