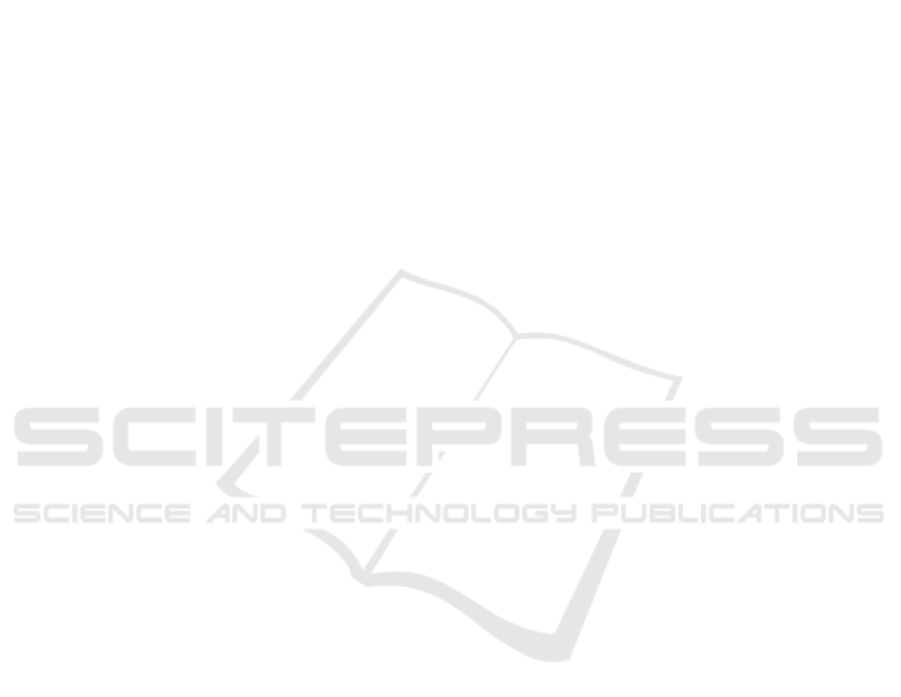
On Trimmed Data Effect in Parameter Estimation of
Some Population Growth Models
Windarto, Eridani and Utami Dyah Purwati
Department of Mathematics, Faculty of Science and Technology, Universitas Airlangga, Surabaya, Indonesia
Kampus C Universitas Airlangga, Mulyorejo, Surabaya 60115, Indonesia
Keywords: Growth Model, Parameter Estimation, Chicken Weight, Trimmed Data.
Abstract: Logistic model, Gompertz model, Richard model, Weibull model and Morgan-Mercer-Flodin model are
commonly used to describe growth model of a population. In this paper, we study the effect of trimmed data
on parameter estimation results of those models. We use chicken weight data cited from literature.
Parameter values of the models from the complete data and the trimmed data are compared. Then, the
sensitivity index of all parameters is evaluated. We found that that sensitivity order of the models from the
highest sensitivity was the Morgan-Mercer-Flodin, Weibull, Richards, logistic and Gompertz growth model.
For practical applications, Gompertz model and Richards are recommended in order to modelling growth of
a population.
1 INTRODUCTION
Mathematical growth models have been widely
applied to explain body weight and age relationship
in veterinary sciences. From the mathematical
growth model, one can evaluate some important and
practical parameters, e.g. the mature weight, the
maturing rate and the growth rate of an animal. The
parameters are beneficial tool to give estimations of
the daily feed needs or to evaluate the effect of
environmental condition on the weight growth of an
animal. In addition, the mathematical growth models
could be applied to forecast the optimum slaughter
age. Therefore, mathematical growth models could
be considered as an optimization instrument for the
animal production (López et al., 2000; Vázquez et
al., 2012; Teleken et al., 2017).
The mathematical growth model could be
classified into two groups, namely empirical growth
models and the empirical growth model and
dynamical growth models (the growth model
derived from ordinary differential equations). The
empirical growth models include Weibull growth
model and MMF (Morgan-Mercer-Flodin) growth
model. The Weibull and the MMF growth model
have been applied to describe chicken growth
dynamic (Topal and Bolukbasi, 2008). The
dynamical growth model includes logistic growth
model, Gompertz growth model, and Richards
growth model. These dynamical growth models have
been used to describe the growth kinetics of many
animals, including chicken (Aggrey, 2002),
mammal (Franco et al., 2011), fish (Santos et al.,
2013), reptile (Bardsley et al., 1995) and
amphibian (Mansano et al., 2013).
Topal and Bolukbasi reported that the MMF,
Weibull and Gompertz the MMF, Weibull and
Gompertz growth model can be useful for describing
chicken growth performance, since these models
were the best fitted models (Topal and Bolukbasi,
2008). Aggrey found that the Richards and
Gompertz growth model have the best fitted model
in explaining rooster and hen growth dynamics
(Aggrey, 2002). Zadeh and Golshani also reported
that the Richards growth model provided the best fit
to the growth curve of Iranian Gulian sheep (Zadeh
and Golshani, 2016).
A mathematical growth model could be said as
a good model if the model give accurately
predicted result and it is robust with trimmed
data. In this context, we compare robustness of
some mathematical growth model due to
trimmed data effect. We use sensitivity index to
measure robustness performance of the models.
We use chicken weight data cited from literature.
This paper is organized as follows. Section 2
briefly presents some mathematical growth models.
Section 3 presents effect of trimmed data on
robustness performance of the selected models.
Finally, conclusions are presented in Section 4.
Windarto, ., Eridani, . and Purwati, U.
On Trimmed Data Effect in Parameter Estimation of Some Population Growth Models.
DOI: 10.5220/0008516300050008
In Proceedings of the International Conference on Mathematics and Islam (ICMIs 2018), pages 5-8
ISBN: 978-989-758-407-7
Copyright
c
2020 by SCITEPRESS – Science and Technology Publications, Lda. All rights reserved
5