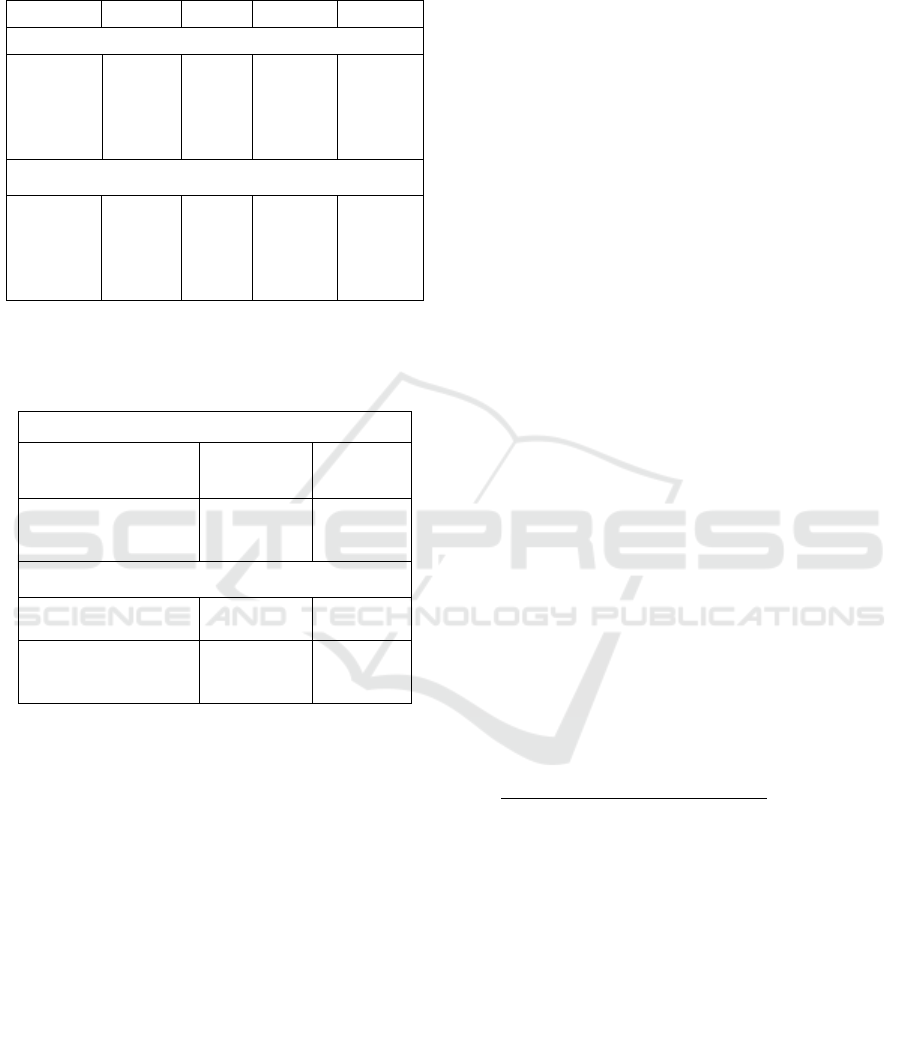
Table 2: The average of norm of error covariance matrices
for different numbers of ensembles in the disturbance
detection using EnKF.
Detection one disturbance
Average of
Norm of
Error
Covariance
matrices
Detection two disturbances
Average of
Norm of
Error
Covariance
matrices
Table 3. Comparison of the average of norm of error
covariance matrices between EnKF (
and KF in
detection of one and two disturbances.
Detection one disturbance
Average of Norm of
Error Covariance
matrices
Detection two disturbances
Average of Norm of
Error Covariance
matrices
4 CONCLUSIONS
In this study, the KF and EnKF method succeed to
detect the heat disturbances in cylindrical-shaped
metal chip. Detection of heat disturbances has been
carried out for 1–4 disturbances in different
positions. Based on the average of norm of error
covariance matrices, the EnKF is more accurate
detect the disturbance than KF. The heat
disturbances can be detected more clearly if the
temperature of disturbance is large enough,
especially for detection in the edge of chip (close to
inner radius and outer radius). In addition, the more
number of grids, the more accurate the position of
detection.
REFERENCES
Apriliani, E. and Sofiyanti, W., 2011. The Sensitivity of
Ensemble Kalman Filter to Detect the Disturbance of
One Dimensional Heat Transfer, Jurnal Matematika &
Sains, Desember 2011, Vol. 16 (3), pp. 133–139.
Butala, M. D., Yun, J., Chen, Y., Frazin, R. A. and
Kamalabadi, F., 2008. Asymptotic Convergence of
The Ensemble Kalman Filter, 15th IEEE International
Conference on Image Processing.
Carslaws, H. dan Jeager, J., 1959. Conduction of Heat In
Solids, second Edition, London: The Clerendon Press.
Emara-Shabaik, H. E., Khulief, Y. A. and Hussaini, I.,
2002. A non-linear multiple-model state estimation
scheme for pipeline leak detection and isolation.
Proceedings of the Institution of Mechanical
Engineers, Part I: Journal of Systems and Control
Engineering, 216:497.
Evensen, G., 2003. The Ensemble Kalman Filter:
Theoretical formulation and practical implementation.
Ocean Dynamic, 53, 343–367.
Gland, F. L., Monbet, V. and Tran, V. D., 2009. Large
Sample Asymptotics for Ensemble Kalman Filter,
Institut National De Recherche En Informatique En
Automatique.
Hamilton, J. D., 1994. Time Series Analysis, Princeton
University Press, Princeton New Jersey.
Kalman, R. E., 1960. A New Approach to Linear Filtering
and Predictions Problems, Journal of Basic
Engineering 82, 34–45.
Lienhard, J. H., 1930. A heat Transfer Textbook 3
rd
ed./
John H. Lienhard IV and John H. Lienhard V,
Cambridge, MA: J.H. Lienhard V, c2000.
Liu, M., Zang, S. and Zhou, D., 2005. Fast leak detection
and location of gas pipelines based on an adaptive
particle filter. International Journal of Applied
Mathematics and Computer Science, 15(4), pp. 541-
550.
Mandel, J., Cobb, L. and Beezley, J. D., 2009. On the
Convergence of the Ensemble Kalman filter,
University of Colorado Denver CCM Report 278
http://www.arXiv.org/abs/0901.2951.
Tan, M., 2011, Mathematical Properties of Ensemble
Kalman Filter, Dissertation of Faculty of the USC
Graduate School University of California, California.
ICMIs 2018 - International Conference on Mathematics and Islam
14