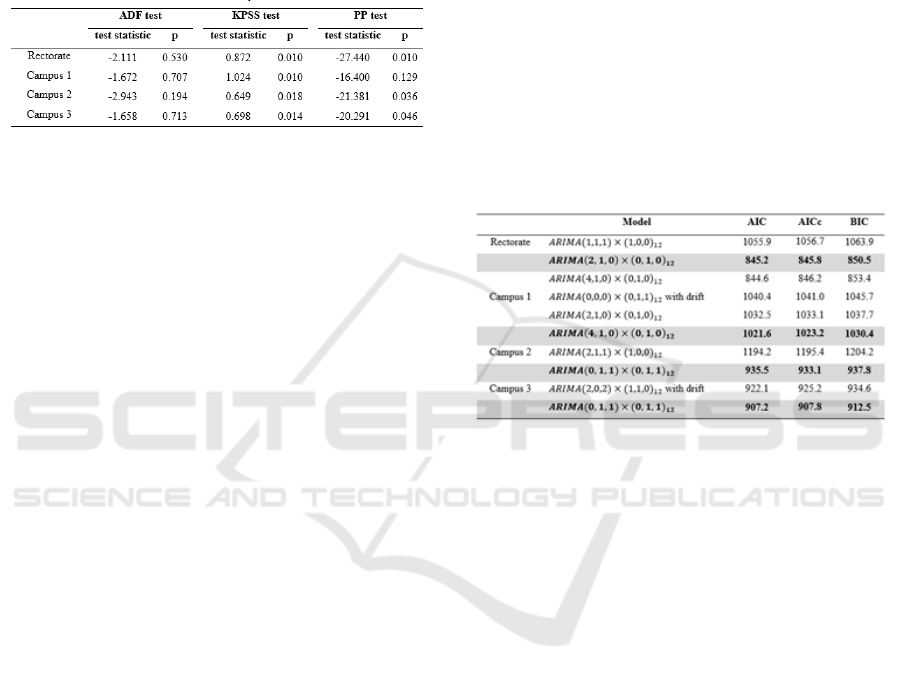
rejected. The results of KPSS tests are significant
meaning the null hypothesis of stationary process is
rejected. Therefore, we need to take first difference
to the time series data. The general upward trend has
disappeared after we take first difference to the data
but the strong seasonality is still present.
Table 3: ADF, KPSS, and PP tests for the time series data.
Observing both ACF and PACF plots of the
series after taking first and seasonal difference (see
Appendix), we come up with several potential
models for each building as summarized in Table 4.
For electricity consumption pattern in Rectorate
building, the PACF shows a clear spike at lag 2 or 4.
A non-seasonal AR(2) or AR(4) may be useful part
of the model. In the ACF, there appears no
significant lag. Thus, the proposed model for the
series of electricity consumption in Rectorate
building is
or
.
For electricity consumption pattern in Campus 1
building, the PACF also shows a clear spike at lag 2
or 4. A non-seasonal AR(2) or AR(4) may be useful
part of the model. Thus, the proposed model for the
series of electricity consumption in Campus 1
building is
or
.
For electricity consumption pattern in Campus 2
building, the ACF shows a clear spike at lag 1. A
non-seasonal MA(1) may be useful part of the
model. In the PACF, there’s a cluster of (negative)
spikes around lag 12 and then not much else. This
might indicate the need for a seasonal MA(1)
component. Thus, the proposed model for the series
of electricity consumption in Campus 2 building is
.
For electricity consumption pattern in Campus 3
building, the ACF shows a clear spike at lag 1. A
non-seasonal MA(1) may be useful part of the
model. In the PACF, there’s a cluster of (negative)
spikes around lag 12 and then not much else. This
might indicate the need for a seasonal MA(1)
component. Thus, the proposed model for the series
of electricity consumption in Campus 2 building is
.
Automatic procedure to select the order of
seasonal and non-seasonal component was also
performed with R by using auto.arima function. The
comparisons of the proposed models are shown in
Table 4.4. Based on AIC and BIC values, the best
fitted model for electricity consumption pattern in
Rectorate building is
.
The best fitted model for electricity consumption
pattern in Campus 1 building is
with drift. The best fitted model for
electricity consumption pattern in Campus 2
building is
. The best
fitted model for electricity consumption pattern in
Campus 3 building is
.
Table 4: AIC and BIC comparison for the proposed
models.
Based on AIC and BIC values, the best fitted
model for electricity consumption pattern in
Rectorate building is
.
The best fitted model for electricity consumption
pattern in Campus 1 building is
with drift. The best fitted model for
electricity consumption pattern in Campus 2
building is
. The best
fitted model for electricity consumption pattern in
Campus 3 building is
.
Using the Maximum Likelihood estimator, the
model parameters are estimated. Table 5 summarizes
the estimated coefficient and standard error of the
best fitted seasonal ARIMA models.
Diagnosis analyses are also performed to the four
models to evaluate the model assumption such as no
correlation in the residual series. Assumption of no
correlation in residuals is investigated by performing
Ljung-Box test (Table 6). The result of Ljung-Box
for the residual series from the model fitted to the
Rectorate data are not significant since the test fails
reject the null hypothesis of no autocorrelation in the
residual series (p = 0.422). The result of Ljung-Box
for the residual series from the model fitted to the
Campus 1 data are not significant since the test fails
reject the null hypothesis of no autocorrelation in the
Energy Saving Potential Prediction and Anomaly Detection in College Buildings
19