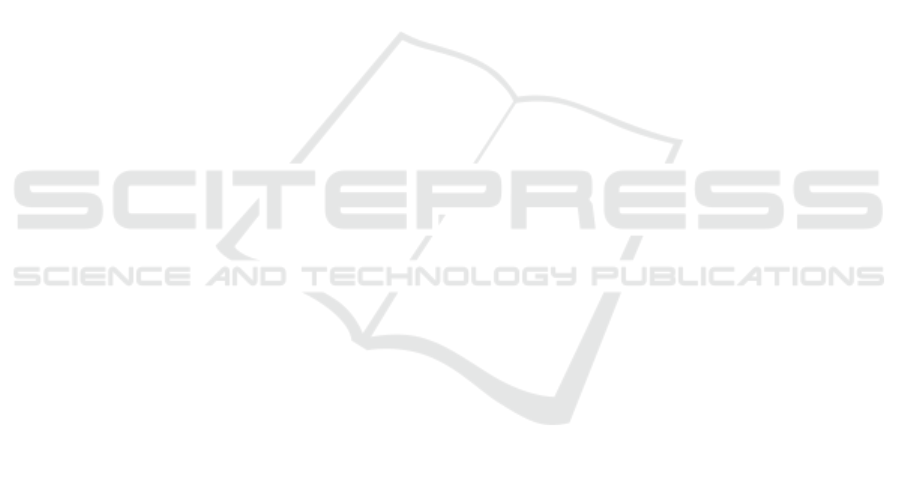
is going to have Branch library with a confidence
level of 96.55%, and lift ratio value is 3.4113.
From the lift ratio value, we can see how strong
the rule formed by the apriori pattern mining
algorithms. The value of lift ratio is positive (≥
0). If the lift ratio value equals to 1, then the rule
{City includes Losangeles} => {Location
type=Branch library} often occurred together but
independently.
d. Rule{City includes Baltimore} => {Location
type=Branch library}
Rules with support value is 0.0012, confidence is
0.9167 and lift ratio is 3.`2387. It’s mean that
0.12% or about 33 Branch library of the whole
location type happen in Baltimore, Baltimore is
going to have Branch library with a confidence
level of 91.67%, and lift ratio value of 3.2387.
From the lift ratio value, we can see how strong
the rule formed by the apriori pattern mining
algorithms. The value of lift ratio is positive (≥
0). If the lift ratio value equals to 1, then the rule
{City includes Baltimore} => {Location
type=Branch library} often occurred together but
independently.
e. Rule{City includes Sandiego} => {Location
type=Branch library }
Rules with support value is 0.0013, confidence is
0.8293 and lift ratio is 2.9299. It’s mean that
0.13% or about 33 Branch library of the whole
location type happen in Sandiego, Sandiego is
going to have Branch library with a confidence
level of 91.67%, and lift ratio value of 3.2387.
From the lift ratio value, we can see how strong
the rule formed by the apriori pattern mining
algorithms. The value of lift ratio is positive (≥
0). If the lift ratio value equals to 1, then the rule
{City includes Sandiego} => {Location
type=Branch library} often occurred together but
independently.
f. Rule{City includes Miami} => {Location
type=Branch library}
Rules with support value is 0.0001, confidence is
0.80 and lift ratio is 2.8265. It’s mean that 0.1%
or about 27 Branch library of the whole location
type happen in Miami, Miami is going to have
Branch library with a confidence level of
91.67%, and lift ratio value of 3.2387. From the
lift ratio value, we can see how strong the rule
formed by the apriori pattern mining algorithms.
The value of lift ratio is positive (≥ 0). If the lift
ratio value equals to 1, then the rule {City
includes Miami} => {Location type=Branch
library} often occurred together but
independently.
4 CONCLUSION
The results of association rules analysis can be
concluded that the most recommended rules are
Rules{City includes Chicago} => {Location
type=Branch library}; Rules{City includes
Brooklyn} => {Location type=Branch library};
Rules{City includes Losangeles} => {Location
type=Branch library}; Rules{City includes
Baltiomore} => {Location type=Branch library};
Rules{City includes Sandiego} => {Location
type=Branch library}; Rules{City includes Miami}
=> {Location type=Branch library}. From the six
rules mentioned above, we known that the most
visited library in these six states in US is Branch
Library. It’s because the location of the Branch
Library usually near to people’s houses. Therefore,
to be visited by more people, the main branch library
should be developed near to the people’s house.
Management of other types of libraries should refer
to branch library management. It’s shown by the
result of the association rules analysis, which
confirmed that the Branch Library is the library that
most visited by population in that area.
REFERENCES
ALA Library, 2014. The Nation's Largest Public
Libraries: Top 25 Rankings. American Library
Association.
Agrawal, R., Imielinski, T., and Swami, A., 1993. Mining
association rules between sets of items in large
databases. Proceedings of the 1993 ACM SIGMOD
International Conferenceon Management of Data,
SIGMOD ’93, New York, NY, USA, pp.207–216.
ACM Press.
Berry, J. K., 1993. Beyond Mapping: Concepts,
Algorithms and Issues in GIS, Fort Collins, CO: GIS
World Books.
Brin, S., Motwani, R., and Silverstein., 1997. Beyond
Market Baskets: Generalizing Association Rule to
Correlations. Proceedings ACM SIGMOD Conference
on Management of Data (SIGMOD1997), pp. 265 –
276.
Han, J. and Kamber, M., 2006. Data Mining Concepts and
Techniques Second Edition. Morgan Kauffman: San
Francisco, 2006.
Jiawei, H., and Micheline, K., 2006. Data Mining:
Concepts and Techniques. MORGAN KAUFMANN
PUBLISHER, An Imprint of Elsevier.
Nath, A. 2015. Big Data Security Issues and Challenges.
International Journal of Innovative Research In
Advanve Enggineering, 2(2), 15–20
Apriori Algorithm for Frequent Pattern Mining for Public Librariesin United States
63