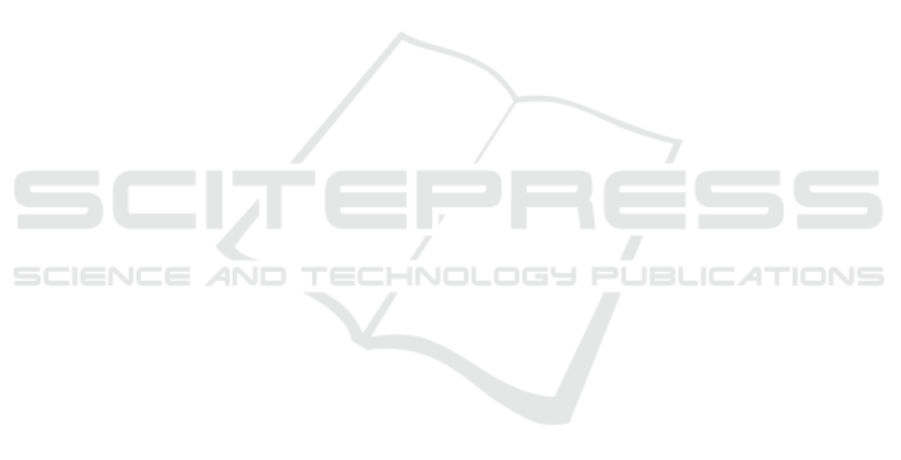
5 CONCLUSIONS
Based on, the experiment that has been performed,
batik classification into two classes and three classes
get the best results when using GLCM feature
extraction method with an accuracy of 96% for two
type of batik motifs, accuracy of 88.89% for three
kinds of batik motifs and accuracy of 77.14% for four
kinds of batik motifs. This indicates that the feature
extraction using GLCM method is better than the
DWT method to recognize batik pattern based on the
pattern.
ACKNOWLEDGMENTS
A big thank you to Allah SWT for giving blessings
and guidance in the life of the author, UINSA which
has provided an opportunity for the author to seek
knowledge.
REFERENCES
Achjadi, J., 1999. Batik Spirit of Indonesia. Jakarta: PT.
Buku Antar Bangsa.
Babasaheb G. P., Mane, N. N., and Subbaraman, S., 2012.
IRIS Feature Extraction and Classification using
FPGA. International Journal of Electrical and
Computer Engineering (IJECE), Vol 2, No 2, April
2012, 214–222.
Chen, D.-R., Chang, R.-F., Kuo, W.-J., Chen, M.-C., &
Huang, Y. .-L., 2002. Diagnosis of breast tumors with
sonographic texture analysis using wavelet transform
and neural networks. Ultrasound in Medicine &
Biology, 28(10), 1301–1310.
Chih-Wei Hsu, 2002. A comparison of methods for
multiclass support vector machines. IEEE Transactions
on Neural Networks, 12(2), 425–547.
Embaugh, S. E., 2017. Digital Image Processing and
Analysis Applications with MATLAB and CVIPtools
(Third Edition). Boca Raton: CRC Press.
Fanani, A., Yuniarti, A., & Suciati, N., 2014. Geometric
Feature Extraction of Batik Image Using Cardinal
Spline Curve Representation. TELKOMNIKA
(Telecommunication Computing Electronics and
Control), 12(2), 397.
He, X., Wang, Z., Jin, C., Zheng, Y., & Xue, X., 2012. A
simplified multi-class support vector machine with
reduced dual optimization. Pattern Recognition Letters,
33(1), 71–82.
Jayaraman, S., Esakkirajan, S., & Veerakuma, T., 2011.
Digital Image Processing. New Delhi: Tata McGraw
Hill Education Private Limited.
Karthikeyan, S., & Rengarajan, N., 2013. Performance
analysis of gray level cooccurrence matrix texture
features for glaucoma diagnosis. American Journal of
Applied Sciences, 11(2), 248–257.
Moertini, V. S. & Sitohang, B., 2005. Algorithms of
Clustering and Classifying Batik Images Based on
Color, Contrast and Motif. ITB Journal of Engineering
Science, 37(2), 141–160.
https://doi.org/10.5614/itbj.eng.sci.2005.37.2.5
Mohanaiah, P., Sathyanarayana, P., & Gurukumar, L.,
2013. Image Texture Feature Extraction Using GLCM
Approach. International Journal of Scientific &
Research Publication, 3(5), 1–5.
Musman, A., & Arini, A. B., 2011. Batik Warisan
Adhiluhung Nusantara. Yogyakarta: G Media.
Öztürk, Ş., & Akdemir, B., 2018. Application of Feature
Extraction and Classification Methods for
Histopathological Image using GLCM, LBP,
LBGLCM, GLRLM and SFTA. Procedia Computer
Science, 132 (ICCIDS), 40–46.
Prasetyo, E., 2014. Data Mining Mengolah Data Menjadi
Informasi Menggunakan MATLAB. Yogyakarta: Andi.
Putra, R. E., Suciati, N., & Wijaya, A. Y., 2011.
Implementing Content Based Image Retrieval for Batik
Using Rotated Wavelet Transform And Canberra
Distance. Bali International Seminar on Science and
Technology, 1–5.
Rangkuti, A. H., 2014. Content based batik image
classification using wavelet transform and fuzzy neural
network. Journal of Computer Science, 10(4), 604–613.
Shi, Y. Q., & Jeon, B., 2006. Digital Waternarking. Korea:
Springer.
Suresh, A., 2012. Image Texture Classification using Gray
Level Co-Occurrence Matrix Based Statistical
Features. European Journal of Scientific Research,
75(4), 591–597.
Tzotsos, A., and Ardialas, D., 2008. Support vector
machine classification for object-based image analysis.
Final Paper. Retrieved from http://datascienceassn.
org/sites/default/files/A%20Support%20Vector%20M
achine%20Approach%20for%20Object%20Based%20
Image%20Analysis.pdf, accessed on December 8,
2018.
Wulandari, A., 2012. Batik Nusantara - Makna Filosofis,
Cara Pembuatan & Industri Batik. Yogyakarta: Andi.
Zulpe, N., & Pawar, V., 2012. GLCM textural features for
Brain Tumor Classification. International Journal of
Computer Science, 9(3), 354–359.
Batik Classification using Texture Analysis and Multiclass Support Vector Machine
71