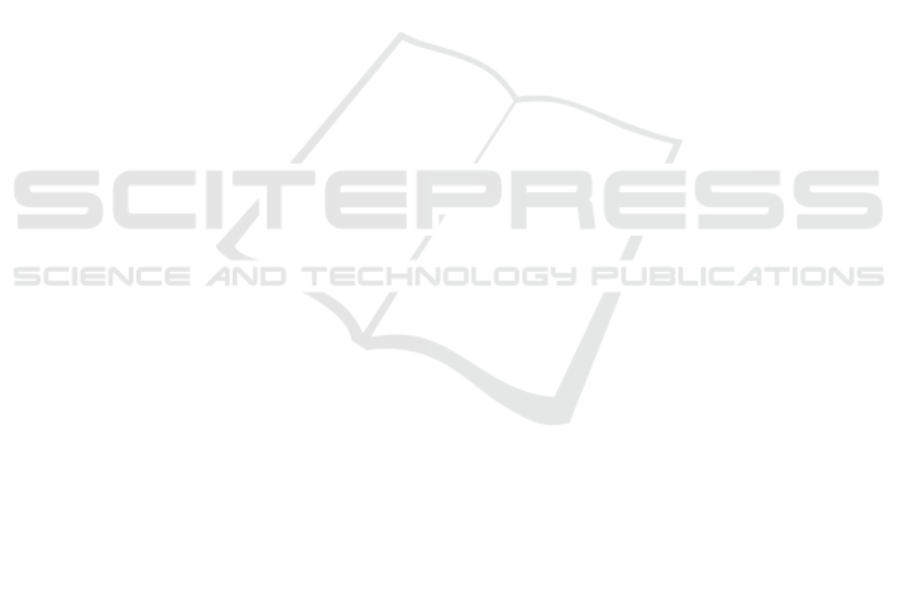
Index (SPI) (Mckee et al.,1993). Therefore, it is
necessary to forecast rainfall as basic information in
determining the index of drought in the future.
Some research on rainfall forecasting has been
done by some researchers such as Lusiani (Abraham
and Ledolter, 1983) modeling rainfall ARIMA in
Bandung, Ukhra (2014) modeling and forecasting
time series data with SARIMA and Retnaningrum
(2015) application of STAR (Space Time
Autoregressive) and ARIMA for forecasting rainfall
data in Jember district. These studies are limited to a
single point of forecasting data without considering
a certain probability interval. Prediction intervals is
important part in forecasting process to knowing
indication of uncertainty in the approximate point.
Research on Prediction intervals has been done
among others Yar and Chatfield (1990) prediction
intervals for Holt-Winters forecasting procedures,
Chatfield (1993) calculating prediction intervals, and
Safitri (1995) prediction intervals for time series
models. In addition to determining future rainfall
predictions required research on the level of drought
that occurred in a region. Mutjahiddin (2014)
concluded that the drought was due to a deviation of
weather conditions from normal conditions
occurring in a region. Such deviations can be
reduced rainfall compared to normal conditions.
Kurniawan (2016) studied the combination of
ARIMA and Standardized Precipitation Index (SPI)
to determine the drought index in Boyolali district.
The location of the diverse topography and
natural conditions that experienced a very dynamic
temperature changes causes Jember district made
several efforts to reduce the impact that occurred.
Based on the above research researchers want to
provide new research. Researchers want to provide
forecasting interval for rainfall with time series
model containing seasonal parameter and
determining dryness level from rainfall prediction
result. This research data use rainfall data 77 rain
stations in Jember Regency spread based on
topography location. Finally, calculate the prediction
interval of forecasting value by using SARIMA
model and analyze the drought rate that will occur
with SPI method.
2 MATERIALS AND METHODS
2.1 Data Set
Rainfall data from 77 rain stations in Jember
Regency from January 2005 to December 2017.
Rainfall data is divided into two kinds, namely in-
sample data and out-sample data. In-sample data is
rainfall data from January 2005 to December 2016.
While the out-sample data is rainfall data from
January 2017 to December 2017. Variables used in
this study based on research that has been done by
Hadi (2017) namely:
X
1
(t) : Average rainfall in Jember region zone 1
X
2
(t) : Average rainfall in Jember region zone 2
X
3
(t) : Average rainfall in Jember region zone 3
X
4
(t) : Average rainfall in Jember region zone 4
2.2 SARIMA Model
Seasonal ARIMA Model is an ARIMA model used
to complete a seasonal time series consisting of two
parts, i.e. non-seasonal (seasonal) and seasonal parts.
The non-seasonal part of this method is the ARIMA
model. The general SARIMA Model is
. (1)
Estimate of parameters is done by using Maximum
Likelihood Estimation (MLE). The assumption
required in the MLE method is the error (time error
value t) is normally distributed (Box and Jenkins,
1976):
(2)
Best selection model is based on the AIC. Best
model derived from smaller AIC. M is a data to be
predicted. then AIC calculation is formulated with
the following equation (Wei, 2016; Bowerman and
O’onner, 1987):
(3)
2.3 Prediction Interval
An observed time series, n observations, is
denoted by
). Suppose we wish to
forecast the value of the series k steps ahead. This
means we want to forecast the observe value at time
(t+k). The point forecast of the value at time (t+k)
data up to time n is denoted by
(4)
When the value later becomes available, we can
know the corresponding forecast error, denoted by
forecast error, like that for the point forecast,
specifies both the horizon and the time period when
the forecast was made.
ICMIs 2018 - International Conference on Mathematics and Islam
102