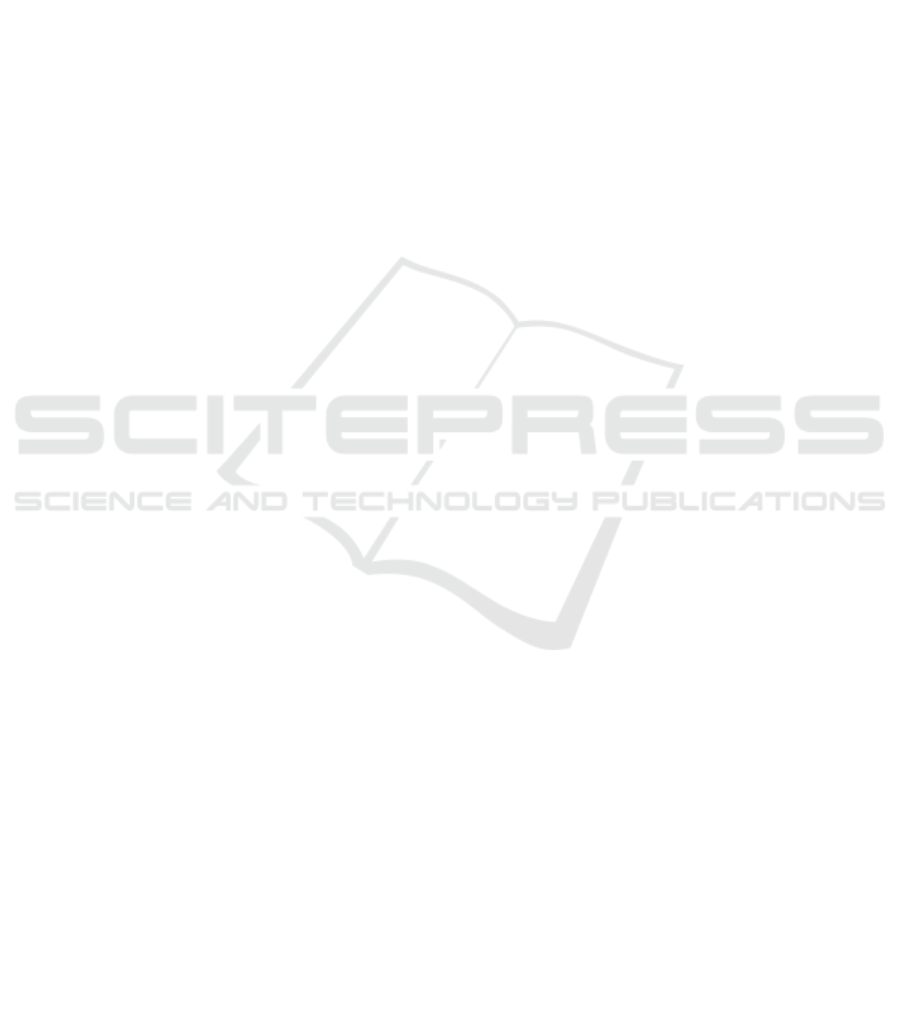
Modeling on Electricity Consumption’s Average of Households
Group in Surabaya with Nonparametric Approach based on Fourier
Estimator
Eko Tjahjono, Sediono, M. Fariz Fadillah Mardianto
and Ajeng Novy Lestari
Department of Mathematics, University of Airlamgga, Surabaya, Indonesia
Keywords: Electricity, Temperature, Nonparametric Regression, Fourier Series.
Abstract: Demand of electrical power increase in line with the number of populations, technological advances, and
information. Household groups are the biggest users of electricity every year. The case study in this research
that be used about electricity usage in Surabaya city. Surabaya is the capital of the province with very high
electrification that equal to 99,79%. This proves that Surabaya can be used as an indicator of electricity. One
factor that influence the high usage of electricity is temperature. The higher the air temperature caused the
use of electrical equipment is increasing so that the used of electricity increases. Prediction of electrical used
is needed to anticipate electricity supply as well as anticipation action against power outage which can disturb
human activities. In this study, electricity consumption of household group in Surabaya was observed
monthly, then predicted using nonparametric regression method based on Fourier series estimator. One of the
advantages of the Fourier series is that it can overcome data that has repeating pattern. This study results a
minimum GCV value when the oscillation parameter reaches 11. The chosen model based on minimum GCV
has met the model goodness criteria. Then, the selected model used to predict the household electricity usage
in Surabaya for some time ahead.
1 INTRODUCTION
Electricity is one of the most important and vital
energy for human life, so it cannot be separated every
day. Nowadays the need for electric energy is
increasing along with the increase of population and
technological progress and information. Currently
electrical energy has been classified as the basic
needs of an area used by five groups of power users.
Household groups are the largest group of electric
energy users in each year. The average population
growth of Surabaya City in 2007 was 0,1172 with a
population of 2.720.156 people and the number of
households was 755.914. The condition of
electrification ratio in 2007 is very high, amounting
to 99,79%. Thus, this is the basic research why use
the city of Surabaya.
Determination of the future of electrical energy is
influenced by various factors. Weather changes cause
changes to consumer comfort and affect the use of
equipment including electrical equipment. The higher
temperature of the air will cause the use of electrical
equipment is greater so that electricity consumption
is increasing.
In this research, electricity consumption’s average
of households group in Surabaya is modeled using
nonparametric regression approach based on Fourier
series estimator. One of the advantages of
nonparametric regression approach using Fourier
series can overcome data having trigonometric
spreading, in this case sine and cosine. The data
patterns corresponding to the Fourier series approach
are repetitive data patterns. Research on the Fourier
series includes Bilodeau (1992), Tripena and
Budiantara (2006).Tjahjono (2009) studied Fourier
series estimators on nonparametric regression, and
Tjahjono et al., (2018) developed an estimated
nonparametric regression model of the Fourier bi-
response series.
2 FOURIER SERIES ESTIMATOR
Fourier series is a trigonometric polynomial function
that has a high flexibility. It is because the pattern of
Tjahjono, E., Sediono, ., Mardianto, M. and Lestari, A.
Modeling on Electricity Consumption’s Average of Households Group in Surabaya with Nonparametric Approach based on Fourier Estimator.
DOI: 10.5220/0008518501470150
In Proceedings of the International Conference on Mathematics and Islam (ICMIs 2018), pages 147-150
ISBN: 978-989-758-407-7
Copyright
c
2020 by SCITEPRESS – Science and Technology Publications, Lda. All rights reserved
147