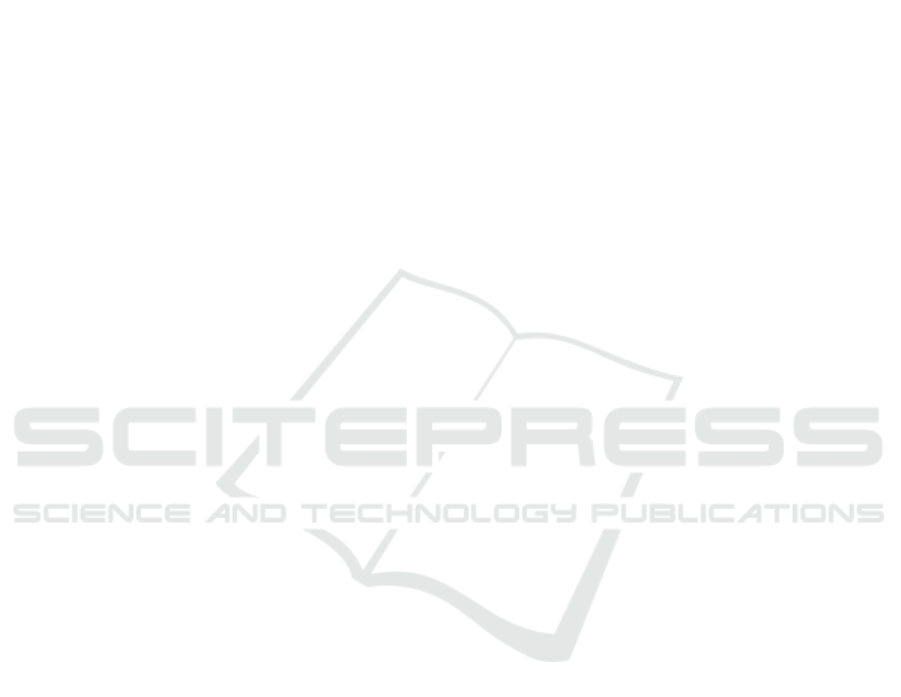
Analysis of Poverty Data in Bengkulu City by Small Area Estimation
using Penalized Splines Regression
Idhia Sriliana
1
, Etis Sunandi
1
and Ulfasari Rafflesia
2
1
Department of Statistics, the University of Bengkulu, Bengkulu, 38125, Indonesia
2
Department of Mathematics, the University of Bengkulu, Bengkulu, 38125, Indonesia
Keywords: Bengkulu City, Penalized Splines Regression, Poverty Data, Small Area Estimation.
Abstract: This study aims to analyze poverty data in Bengkulu City. The method of this study is Small Area Estimation
(SAE) with penalized splines regression approach. Then descriptive statistical analysis is carried out. The data
used is the Bureau of Statistics (BPS) Of Bengkulu with some poverty indicators as predictor variables. The
results showed the best spline model is a model that is considered linear spline with some node points.
Evaluation of model used optimal GCV. The results of descriptive statistical analysis of the average of per
capita outcome at the village level in the city of Bengkulu using the extensive estimation method with the p-
spline regression approach has an average value of Rp.1,009,817.20. About 75% of urban villages in the city
of Bengkulu have an average per capita yield of Rp 1,244,188.15 and the twenty-five percent of urban villages
in Kota Bengkulu have an average per capita outcome about Rp 753.527.25. The high average per capita
outcome is in the Kebun Dahri Village Rp. 3.115.614,20 and the lowest outcome from the Padang Nangka
Village that is Rp 439.830.40.
1 INTRODUCTION
In general, the level of poverty in Bengkulu Province
period 2009-2017 decreased both in terms of quantity
and percentage of exceptions, except in March 2012
and March 2013. Based on the Central Bureau of
Statistics (BPS), the number of poor people in
Bengkulu Province in September 2017 reached
302,620 people (15, 59 %). There was a decreasing
number of poor people by 14,360 people compared to
March 2017. Meanwhile in the last September, the
number of poor people decreased by 22,980 people.
Nevertheless, poverty problem is still be a challenge
for local governments of Bengkulu. The poverty
alleviation becomes the priority programs in
Bengkulu Province, including in Bengkulu City.
Bengkulu city is the capital of Bengkulu province
which the high rate of poverty equals to 20.72%
(BPS, 2018).
Besides policies and programs of poverty
alleviation, poverty data validation is also necessary.
Thus, the program launched is on target. The poverty
is a multi-dimensional problem. It is not easy to
measure and need appropriate measurement
approach. BPS uses the concept of basic needs
approach to measure the poverty data. Through this
approach, poverty is figured as an economic inability
to meet the basic needs of food and not food measured
by expenditure. As result, poor people are people who
the average per capita outcome is lower than the
poverty line (BPS, 2012).
The method that can be used to estimate the
average of per capita outcome as an indicator of
poverty measurement is the Small Area Estimation
(SAE). SAE is a statistical method for predicting
parameters at a subpopulation where the number of
samples is smaller or nonexistent. This estimation
technique uses data from large domains to predict
parameters at smaller domains that can be a village,
sub-district, district, ethnic group, or age group. SAE
methods have concept indirect estimation of
parameters in a relatively small area in survey
sampling, which the direct estimation does not
provide adequate accuracy if the sample size is in a
small area, thus the statistics result will have a large
variance, or the predictions cannot be made because
they are not represented in the survey (Prasad and
Rao, 1990)
Generally, the SAE uses parametric modeling to
link a small area statistic with supporting variables.
However, the SAE model can also be made using the
nonparametric approach. This modeling is more
Sriliana, I., Sunandi, E. and Rafflesia, U.
Analysis of Poverty Data in Bengkulu City by Small Area Estimation using Penalized Splines Regression.
DOI: 10.5220/0008520302530259
In Proceedings of the International Conference on Mathematics and Islam (ICMIs 2018), pages 253-259
ISBN: 978-989-758-407-7
Copyright
c
2020 by SCITEPRESS – Science and Technology Publications, Lda. All rights reserved
253