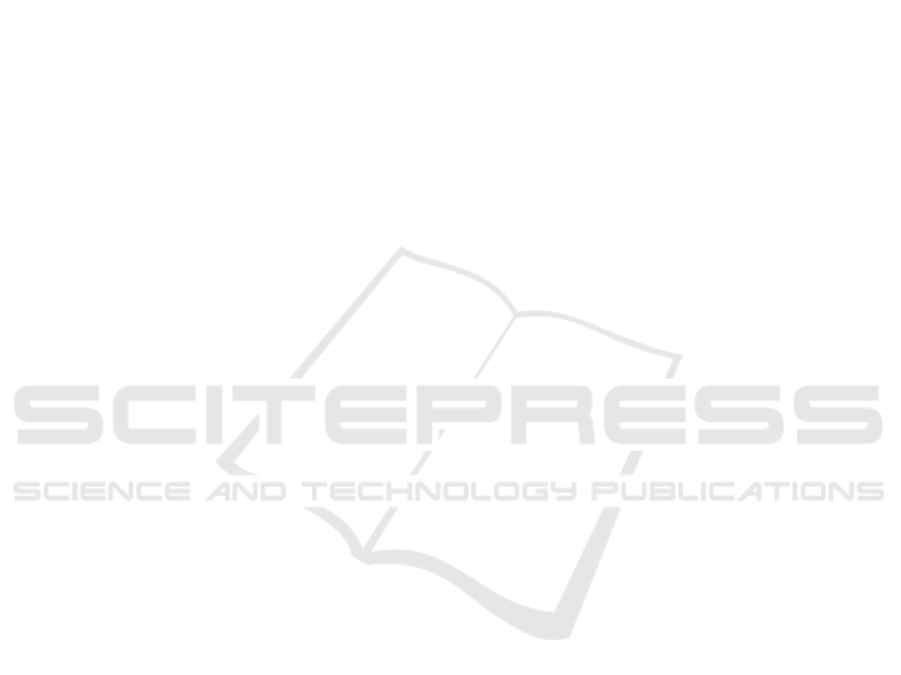
Regression for Trend-Seasonal Longitudinal Data Pattern:
Linear and Fourier Series Estimator
M. Fariz Fadillah Mardianto
1
, Sri Haryatmi Kartiko
2
and Herni Utami
3
1
Department of Mathematics, University of Airlangga, Surabaya, Indonesia
1
Ph.D. Candidate in Department of Mathematics, University of Gadjah Mada, Yogyakarta, Indonesia
2,3
Department of Mathematics, University of Gadjah Mada, Yogyakarta, Indonesia
Keywords: Longitudinal Data, Trend Seasonal Pattern, Regression, Linear Estimator, Fourier Series Estimator.
Abstract: Longitudinal data is a pattern that consists of time series and cross section data pattern. In a research with
longitudinal and panel data often be used combination between trend and seasonal or trend-seasonal pattern,
for example the relationship between profit and demand for seasonal commodities, in education insurance,
meteorology case and many more for many subjects. Recently, we develop Fourier series estimator to
approach curve regression for longitudinal data. Fourier series that be used, not only include trigonometric
Fourier series which usual be used in Mathematics, but also linear function. In this research we compare
performance of new estimator with linear estimator that often be used in panel data regression or parametric
regression for longitudinal data. The trend-seasonal data that be used in this analysis is gotten from
simulation process based on Box et.al., (1976). The Fourier series estimator gives better result with
goodness indicator smaller Mean Square Error (MSE) and greater determination coefficient than linear
estimator.
1 INTRODUCTION
Recently, longitudinal data analysis develops for
some Statistical method. Longitudinal data is a
pattern that consists of more than one subject. Each
subject is observed more than one time. Therefore,
in longitudinal data structure, consist of time series
and cross section data pattern (Weiss, 2005).
In regression analysis, one of statistical method
that be used to model the relationship between
responses and predictors, longitudinal data analysis
often be used. Panel data regression is one of the
linear regressions for longitudinal data. The
differences between longitudinal and panel data,
panel data is longitudinal data with the number of
observations and periods are same for every subject
(Baltagi, 2005).
Regression analysis that be developed is not only
regression with linear estimator, but also
nonparametric regression. Nonparametric regression
is a Statistical modeling that be used to overcome
the relationship between responses and predictors
which have unknown pattern. Nonparametric
regression is an alternative method that be used
when the result of regression analysis with certain
function, such as linear regression, cannot suitable
with goodness criteria of regression analysis
(Takezawa, 2006). The advantage of nonparametric
regression is having high flexibility. Flexibility
means that the pattern of data that presented on the
scatter plot can determine the shape of regression
curve based on estimators in the nonparametric
regression (Budiantara et.al., 2015). Based on plot,
we can identify the pattern of data, the pattern of
pairs data, a response versus a predictor variable
data, have trend, oscillation, uncertain pattern, and
combination pattern.
The pattern of data that often be found is
combination between trend and seasonal or trend-
seasonal data pattern. In research with longitudinal
and panel data this pattern often be encountered.
Some example like, the relationship between profit
and demand for seasonal commodities, in education
insurance, meteorology case and many more for
many subjects.
Trend – seasonal data pattern popular in time
series data analysis. This pattern will pass some
procedure when time series analysis be used, be-
because there are some assumptions must be
satisfied. Time series – regression approach is an
350
M. Fariz Fadillah Mardianto, ., Kartiko, S. and Utami, H.
Regression for Trend-Seasonal Longitudinal Data Pattern: Linear and Fourier Series Estimator.
DOI: 10.5220/0008521803500356
In Proceedings of the International Conference on Mathematics and Islam (ICMIs 2018), pages 350-356
ISBN: 978-989-758-407-7
Copyright
c
2020 by SCITEPRESS – Science and Technology Publications, Lda. All rights reserved