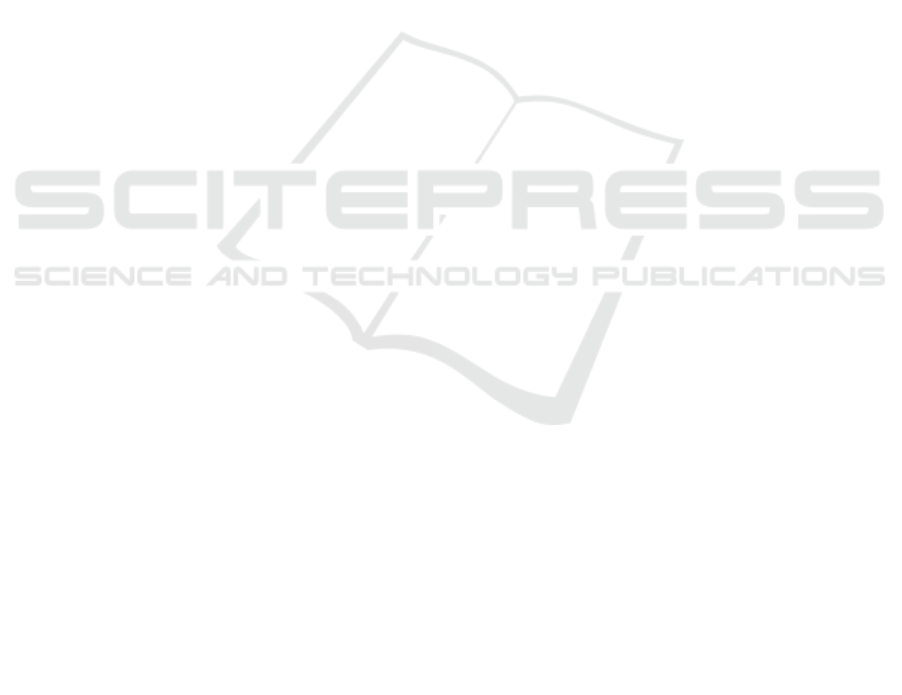
truncated spline estimator. The data used for the
implementation of the estimation of the
semiparametric spline truncated bi-respon regression
model is the CBNE Value in the Department of
Computer Engineering Network of VHS in West
Nusa Tenggara Province in 2017.The correlation test
between the two response variables was carried out,
namely the score of Mathematics CBNE with the
score of competency skill CBNE, using the following
hypothesis: Zero hypothesis (H0) that is if both
variables do not have a linear relationship
;
the alternative hypothesis (H
1
) is if both have a linear
relationship
Based on the results of the
correlation test obtained p-value <0.05 then reject H
0
so that it can be concluded that there is a correlation
between responses.
The next step is modeling the value CBNE of
VHS in the Province of West Nusa Tenggara in 2017
using a semiparametric Bi-response spline truncated
regression model at each knot point. The knot point is
a joint fusion point where data behavior changes.
Optimal knot points are obtained from the minimum
GCV value. Based on the analysis carried out, the best
model is the bi-response semiparametric regression
model based on the truncated spline estimator
resulting in the minimum GCV of 0.00000691 with
three knots. After obtaining the minimum GCV score,
the next step calculates the estimate for the
semiparametric bi-response spline truncated
regression model with three knot points as follows:
1 1 2 3 1
0,24 3,66 5,22 1,74 0,47 y D D D x
2 1 1
1 1 1 1
+26,5t 7,89 11,19( 2) 3,88( 3)t t t
1 2 1
1 2 2 2
+0,64( 5) 53,01 6,26 16,04( 5)t t t t
1 1 2
2 2 3 3
+15,19( 6) 5,43( 7) 6,01 0,11t t t t
11
33
15,57( 66) 27,37( 67) 12,07tt
2 1 2 3 1
136,37 2,14 3,87 3,08 0,26 y D D D x
2 1 1
1 1 1 1
-11,09t 3,51 6,10( 2) 3,5( 3)t t t
1 2 1
1 2 2 2
-1,42( 5) 36,55 4,49 13,31( 5)t t t t
11
2 2 3
+14,28( 6) 5,59( 7) 0,14 1,08t t t
1 1 1
3 3 3
( 83) 0,84( 84) 0,46( 87)t t t
Furthermore, for the criteria of goodness, the
semiparametric bi-response regression model of
truncated spline obtained MSE value of 48.92 with R
2
of 0.90.Based on the two best models, it can be
interpreted as follows: 1) every increase in one unit of
math report card and report card competency skill, it
will result in an increase in CBNE scores in each of
these subjects. 2) The value of CBNE in each subject
is based on the gender of the students, so for male
students it is more than female students. 3) Based on
the value of school accreditation, the school exam
scores on the competency skill subjects are increased
based on the value of school accreditation, meaning
that students of VHS with an accreditation at the
school examination scores on the subjects of
competence are higher than B accreditation; then B
accreditation is higher than C accreditation. 4) then
based on the school distance variable fluctuated at
knots 66, 67, and 68 for the Computer-Based National
Exams scores namely Mathematics CBNE and
Competency Skill CBNE. 5) Likewise, the education
variables of parents experienced fluctuations in knots
5, 6, and 7 for both UNBK scores namely
Mathematics CBNE and Competency Skill CBNE. 6)
Furthermore, for the mathematics school examination
variable values fluctuated at 66, 67, and68 knots for
both CBNE values namely Mathematics and
Competency skill. 7) Likewise, the variable scores on
school competency skills scores also fluctuated at
knots 83, 84, and 87 for both CBNE scores namely
Mathematics and Competency skill.
4 CONCLUSIONS
Based on the two best models, each increase in one
unit of mathematical report value and competency
skills, resulting in an increase in each CBNE value.
Based on gender, male students are higher in value
than female students. Whereas based on the school
accreditation value, the accreditation value of A is
higher than B and C. In school distance variables,
parent education, and school exam scores on
mathematics subjects and competency skill fluctuate
on certain knots in each CBNE value. The result of
the estimation Bi-response semiparametric regression
model, we get the R
2
tends to one (i.e., 90%) and MSE
tend to zero that it satisfies goodness of fit criterions.
ACKNOWLEDGEMENTS
Acknowledgment to the Excellence Scholarship of
the Bureau of Planning and Foreign Cooperation of
the Secretariat General of the Ministry of Education
and Culture which has supported the funding of
tuition fees for the doctoral study.
ICMIs 2018 - International Conference on Mathematics and Islam
360