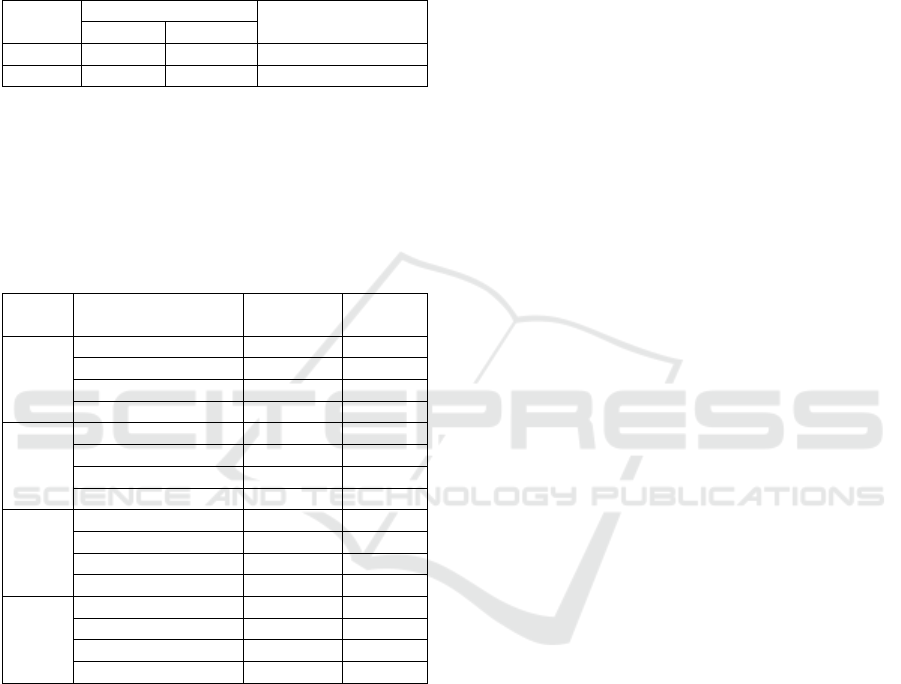
The testing of osteoporosis diagnose uses Table
3, with TP is the patient has the disease and diagnose
is exact, TN is the patient hasn’t diseased and
diagnose is exact, FP is the patient hasn’t diseased
and diagnose is wrong, and FN is the patient has the
disease and diagnose is wrong.
Table 3: Result of Diagnose
Experiment results with four orientation angles
using the kernel polynomials are expressed in Table
4. Then, implementation of SVM Multiclass method
with analysis dental panoramic radiograph images
on the ROI ramus mandibular used as basic on
CADS has been ably used as osteoporosis detection.
Table 4: Value of Confusion Matrix
Working Estimator
Classification
Based on Table 4, extraction features GLCM
with four features statistics shown the best
orientation angle is
and distance pixels.
Results show the accuracy of test data generated of
81.25%, sensitivity of 75%, specificity of 90%, and
precision of 88.89%.
5 CONCLUSIONS
Based on the result of SVM method implementation
Multiclass with the polynomial kernel for
osteoporosis detection, it can be concluded that
SVM Multiclass method with kernel polynomials of
two degrees can be used as the basis of CADS for
osteoporosis detection. Extraction features GLCM
based on a combination of four feature statistics to
identify the value bone mineral density from the one
analysis the mandibular ramus bone has pointed at
the best orientation angle is
with distance
pixels. The results show the best test data accuracy
is 81.25%, sensitivity is 75%, specificity is 90%, and
precision is 88.89%.
REFERENCES
Anand, S., Gayathri, S., 2015. Mammogram image
enhancement by two-stage adaptive histogram
equalization. Elsevier, pp. 3150-3152.
Azhari, et al., 2014. Analisis Citra Radiografi Panoramik
pada Tulang Mandibula untuk Deteksi Dini
Osteoporosis dengan Metode Gray Level Cooccurence
Matrix (GLCM). MKB, pp. 203 - 208.
Fanani, A., Ulinnuha, N., 2016. Watermarking Citra
Digital Menggunakan Metode Discrete Cosine
Transform. Jurnal Matematika "Mantik", pp. 1-7.
Feng, C., Liao, S., 2017. Scalable Gaussian Kernel
Support Vector Machines with Sublinear Training
Time Complexity. Information Sciences Journal, pp.
1-33.
Gonzalez, R. C., Woods, R. E., 1997. Digital Image
Processing. New Jersey: Prentice Hall.
Harrar, K., Hamami, L., 2012. An Interconnectivity Index
for Osteoporosis Assessment Using X-Ray Images.
Journal of Medical and Biological Engineering, 33(6),
pp. 569-575.
Hazra, T. K., Dutta, S., 2015. A New Approach to Identify
the Fracture Zone and Detection of Bone Diseases of
X-Ray Image. International Journal of Science and
Research (IJSR), ISSN (Online): 2319-7064, Paper
ID: ART20161989, pp. 1640-1646.
Kavitha, M. S., An, S.-Y. and An, C.-H., 2014. Texture
Analysis of Mandibular Cortical Bone on Digital
Dental Panoramic Radiographs for the Diagnosis of
Osteoporosis in Korean Women. Journal of Oral
Surgery, Oral Medicine, Oral Pathology and Oral
Radiology, pp. 1-22.
Kikuchi, T., Abe, S., 2005. Comparison between error
correcting output codes and fuzzy support vector
machines. Elsevier, pp. 1937-1945.
Prasetyo, E., 2014. Data Mining, Mengelola Data Menjadi
Informasi Menggunakan Matlab. Yogyakarta: ANDI
Yogyakarta.
Ramkumar, S., Malathi, R., 2016. Detection of
Osteoporosis and Osteopenia using Bone
Densitometer - Simulation Study. Elsevier, pp. 1024-
1036.
Shastry, K. A., Sanjay, H. and Deexith, G., 2017.
Quadratic-Radial-Basis-Function-Kernel for
classifying multi-class agricultural datasets with
continuous attributes. Applied Soft Computing
Journal, pp. 1-30.
Support Vector Machine Multiclass using Polynomial Kernel for Osteoporosis Detection
389