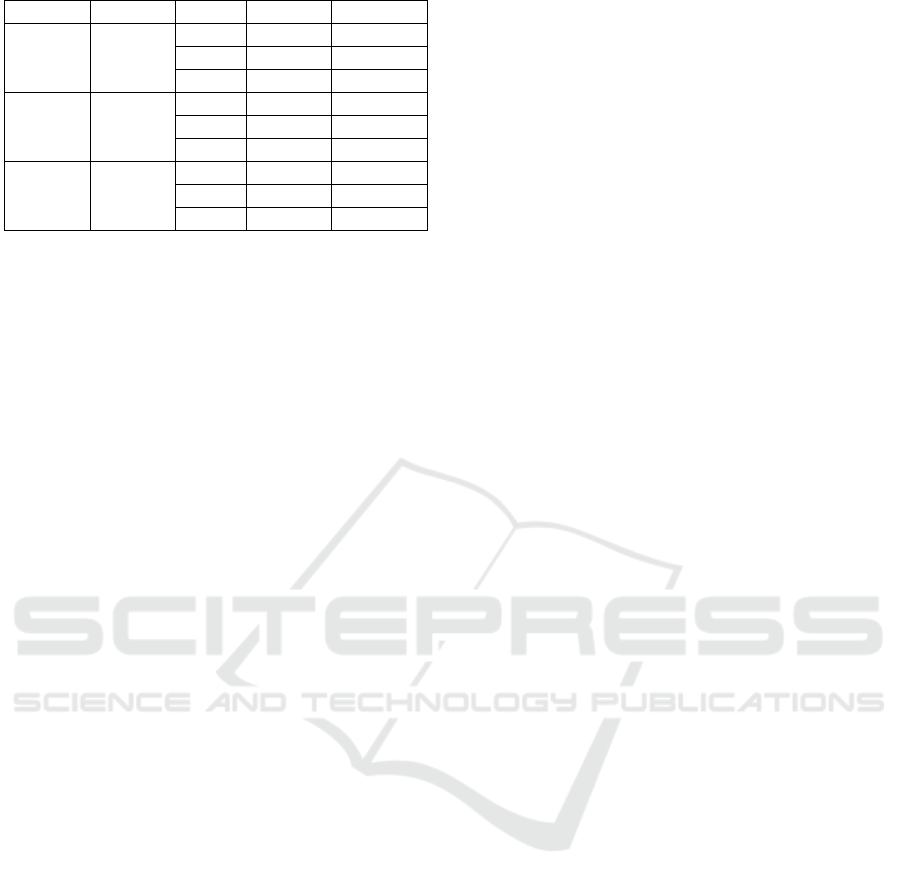
The results in Table 2 show the wavelet analysis
texture has the highest accuracy of 82.14%, while
wavelet decomposition has the highest accuracy
with 75% value. From the results show that the
analysis texture has better accuracy when compared
with the accuracy value generated by wavelet
decomposition. The highest accuracy of Analysis
Texture is shown when using wavelet level 3, with
60% data distribution as training data, and 40% data
testing.
5 CONCLUSIONS
The results show that wavelet texture analysis is
better than wavelet decomposition as feature
extraction method. The statement was supported by
the best accuracy results obtained wavelet texture
analysis of 82.14%, while the best accuracy
possessed by the wavelet decomposition method was
75%. Seeing some of these statements, it can be
concluded that the best feature extraction method
using the brain image is wavelet analysis texture
method.
REFERENCES
Birgale, L V, and M Kokare. 2009. “Iris Recognition
Using Discrete Wavelet Transform.” Digital Image
Processing, 2009 International Conference on
(April): 147–51.
Breckon, C. Solomon; T. 2011. “Fundamentals of Digital
Image Processing : A Practical Approach with
Examples in Matlab. John Wiley & Sons, Inc.,
2011.” John Wiley & Sons Inc.
Chen, Dar-Ren et al. 2002. “Diagnosis of Breast Tumors
with Sonographic Texture Analysis Using Wavelet
Transform and Neural Networks.” Ultrasound in
Medicine & Biology 28(10): 1301–10.
http://www.sciencedirect.com/science/article/pii/S0
301562902006208.
El-dahshan, El-sayed A, Heba M Mohsen, Kenneth
Revett, and Abdel-badeeh M Salem. 2014.
“Computer-Aided Diagnosis of Human Brain
Tumor through MRI : A Survey and a New
Algorithm.” Expert Systems with Applications
41(11): 5526–45.
http://dx.doi.org/10.1016/j.eswa.2014.01.021.
Ferreira, Cristiane Bastos Rocha, and Díbio Leandro
Borges. 2003. “Analysis of Mammogram
Classification Using a Wavelet Transform
Decomposition.” Pattern Recognition Letters 24(7):
973–82.
Jiawei Han and Micheline Kamber. 2006. Data Mining.
Second. San Francisco: Morgan Kaufmann
Publisher.
Jong, Wim de. 2005. “Kanker, Apakah Itu? Pengobatan,
Harpaan Hidup, Dan Dukungan Keluarga.”
Lo, Chien Shun, and Chuin Mu Wang. 2012. “Support
Vector Machine for Breast MR Image
Classification.” Computers and Mathematics with
Applications 64(5): 1153–62.
http://dx.doi.org/10.1016/j.camwa.2012.03.033.
Marinus T. Vlaardingerbroek, Jasques A. Boer. 2013.
Magnetic Resonance Imaging Theory and
Practice.Pdf. New York: Springer Science &
Business Media.
Nadesul, Handrawan. 2011. Menyayangi Otak.Pdf.
Jakarta: Buku Kompas.
Pokorny, Morgan R. et al. 2014. “Prospective Study of
Diagnostic Accuracy Comparing Prostate Cancer
Detection by Transrectal Ultrasound-Guided Biopsy
versus Magnetic Resonance (MR) Imaging with
Subsequent Mr-Guided Biopsy in Men without
Previous Prostate Biopsies.” European Urology
66(1): 22–29.
Riad, Rabia et al. 2018. “Texture Analysis Using Complex
Wavelet Decomposition for Knee Osteoarthritis
Detection: Data from the Osteoarthritis Initiative.”
Computers and Electrical Engineering 68(October
2017): 181–91.
de Rooij, Maarten et al. 2016. “Accuracy of Magnetic
Resonance Imaging for Local Staging of Prostate
Cancer: A Diagnostic Meta-Analysis.” European
Urology 70(2): 233–45.
http://dx.doi.org/10.1016/j.eururo.2015.07.029.
S Jayaraman, S Esakkirajan, T Veerakumar. 2009. Digital
Image Processing.Pdf. New Delhi: Tata McGraw
Hill Education Private Limited.
Seymour, Lynne. 1999. “Image Processing and Data
Analysis, The Multiscale Approach.” 94(448):
1389.
Shih, Fy. 2010. “Image Processing and Mathematical
Morphology: Fundamentals and Applications.”
Wang, Shao Peng, and Yu Dong Cai. 2018. “Identification
of the Functional Alteration Signatures across
Different Cancer Types with Support Vector
Machine and Feature Analysis.” Biochimica et
Biophysica Acta - Molecular Basis of Disease
1864(6): 2218–27.
http://dx.doi.org/10.1016/j.bbadis.2017.12.026.
Brain Disease Classification using Different Wavelet Analysis for Support Vector Machine (SVM)
465