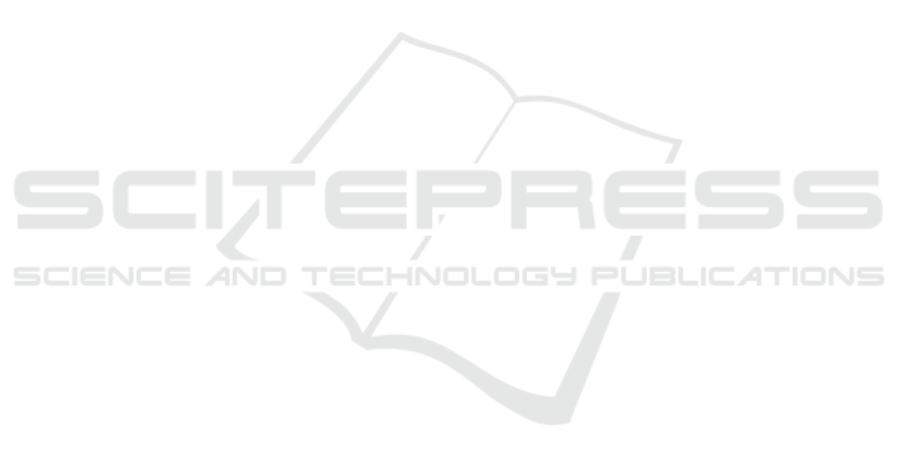
expenditure, student–teacher ratio, politics,
household demography, and socioeconomic status.
Poverty significantly impacts on people’s lives,
not only for those living in poverty but also for people
living in prosperity. Poverty is both individual and
social problem which means every nation should
work together defeating poverty. The best way to
escape poverty is through education (Maipita, 2014,
2016). Empirical evidence shows that better access to
education for lower socioeconomic status is pivotal in
saving a nation from poverty. Poverty can be caused
by: (a) low quality of human resource caused by low
level of education, (b) difficult and limited access of
capital ownership, (c) low technological competence,
(d) inefficient use of resources, and (e) high
population growth (Sharp et al., 2000). Many
research results imply that economic growth can
improve per capita income that will finally lead to the
decrease of poverty ratio (Dollar and Kraay, 2001;
Field, 1989).
3 RESEARCH METHOD
This study followed a model built according to the
main literature from Rajkumar and Swaroop (2008)
and some complementary articles including from
Anyanwu (2007); Checchi (1999); Flug et al. (1998);
Pritchett and Filmer (1999); and Psacharopoulos
(1994). A model of educational data results is
developed into two categories, enrollment ratio and
years of schooling. Both were assumed to be the main
indicators of education besides many other variables.
The categories used for enrollment ratio were
Elementary School (ES), Junior High School (JHS),
and Senior High School (SHS), as the score results of
education. The first model was the determinant of
enrollment which was divided into three levels of
education: ES, JHS, and SHS in net enrollment ratio
(NER). The second model was the determinant of
mean years of schooling (MYS). The independent
variables taken from the result of literature studies
were per capita income; government expenditure on
education; GINI coefficient; and age from people
aged 7-12 years old (Elementary School age), 3-15
year old (Junior High School age), and 16-18 years
old (Senior High School age).
The data used in this study was from the Susenas
2011-2015. The macro economic and fiscal data were
collected from the Central Bureau of Statistics and the
Ministry of Finance, Directorate General for Fiscal
Affairs of the Republic of Indonesia. Unit analysis
was done at the provincial level annually.
Additionally, the econometric model was formed
from panel and time series data during 2011-2015,
and cross section data for provinces in Indonesia.
To analyze the significant relationship between
independent and dependent variables from regression
econometric model, hypothesis testing on the
parameters of population regression function was
conducted. This hypothesis testing covered single
parameter significance test and overall test on the
population regression function. T-test was used for
hypothesis testing in parameters of a single
population while the significance of overall
regression was tested using f-test (Wooldridge,
2009). To identify whether there was
multicollinearity, Variance Inflation Factor (VIF)
was utilized. Then the white test was employed for
analyzing Heterocedasticity.
4 RESULT AND DISCUSSIONS
In this section, the estimation results for each
equation are discussed. The model is estimated by
using the Fixed Effect for 29 provincial data and 5
years of observation from 2011 to 2015. There are 5
provinces that are not included in the estimation
because the data is not available. The five provinces
are: Riau Islands Province, Jakarta Special Capital
Region Province, Gorontalo Province, West Sulawesi
Province and West Papua Province. The 29 provinces
are considered to be sufficient to represent Indonesia.
Hence, the analysis can still be done.
The Fixed Effect model was chosen because it has
the ability to make model specifications for each
variable from the data cross-section. This is to
provide an in-depth analysis of each province in the
model. This makes it easy to determine which
provinces have a greater impact, having different
roles based on coefficient signs.
One of the key indicators of educational
performance is school enrollment and mean years of
schooling. Table 1 is the result of estimation for
elementary school, junior high school (NER_JHS)
and senior high school (NER_SHS) school
enrollment and the estimation results for mean years
of schooling (MYS) as the dependent variable and all
in percent units. Independent variables consisting of
Per Capita Income (PCI) in natural logarithms,
government expenditure on education (GOV_Ed) in
percent units against the GRDP of each province.
GINI variables remain in index units and age
variables. The elementary school age (AGE712),
junior high school age (AGE1315) and senior high
school age (AGE1618) are all in percent units of the
population.
UNICEES 2018 - Unimed International Conference on Economics Education and Social Science
94