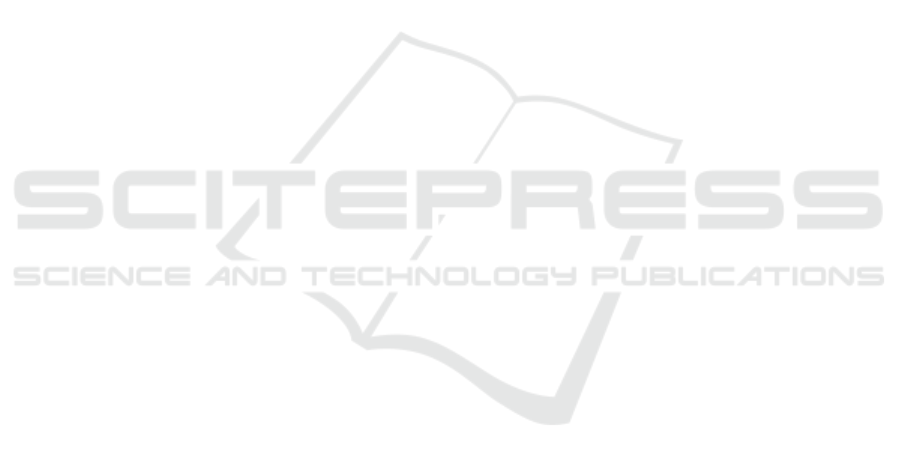
good estimate justified by the mean squared error not
by the graph. We can note that the choice of the num-
ber of clusters in the algorithm depends on how much
data appears to us. It is important to find a number of
clusters that best estimate the input. In statistics, ge-
neralized least squares are techniques for estimating
parameters unknows in a linear regression model. In-
deed, GLS is used to perform a linear regression when
there is some degree of correlation between values
in a model. In this case, the classical weighted least
square method can be statistically ineffective or even
give misleading inferences.
In accordance with previous results (in Tables 5
and 5), the results show that backpropagation asso-
ciated with GLS, under conditions of poor specifica-
tion, provides realistic indices of model implementa-
tion and less biased parameter values for paths that
overlap with the real model. However, despite the re-
commendations of the literature that WLS should be
used when data is not distributed normally, we find
that under no circumstances is the WLS method better
than the other two methods of estimating parameters
in terms of bias and implementation. In fact, only for
large sample and for implementation indices close to
those obtained for backpropagation and the GLS me-
thod. In addition for wrongly specified models, WLS
gives low estimates reliable and overly optimistic va-
lues of fit.
With simulations performed with WLS / GLS
methods associated with backpropagation simulta-
neously, if we increase the number of iterations there
is noise added because it takes more time during the
simulation. It will be the same if we increase the num-
ber of hidden layers where a certain amount of in-
formation will be lost. It is advisable to consider few
layers hidden to avoid noise and a number of reaso-
nable iterations that best justifies the convergence of
the error towards zero. Then the class CL1 is the best
estimated by the GLS associated with backpropaga-
tion method which is more confident and is likely to
help us make a decision.
6 CONCLUSION
In this work the fuzzy model TS has been identi-
fied for our example and for a signal of the road traffic
studied based on data in an autoregressive way. This
method is simple and allowed us to generate data wi-
thout the need to use other variables in addition. Mo-
reover, once the optimization phase is over, the model
is capable to predict other data on the process being
studied. We used design methods based on a learning
that allows to iteratively define the best set of parame-
ters : the optimization of fuzzy rules (WLS, GLS) and
the optimization of membership functions. We also
have proposed one of the WLS or GLS optimization
models with backpropagation to test the convergence
of the error. The results obtained show that even with
a non linear we can hope to obtain quite satisfactory
performance.
REFERENCES
BABU, C. and REDDY, B. (2015). Performance compari-
son of four new arima-ann prediction models on inter-
net traffic data. Journal of Telecommunications and
Information Technology, 1 :67–75.
BABUSKA and al. (2002). Improved covariance estima-
tion for gustafson-kessel clustering. IEEE Internatio-
nal Conference on Fuzzy Systems, pages 1081–1085.
BOUZID, H. and S.BENMERIEM (2013). Application de
la technique de la logique floue pour la prédiction de
l’amorçage des intervalles d’air pointes-plans. Uni-
versité Kasdi Merbah -Ouargla, Algérie.
BOVEIRI, H. and ELHOSENY, M. (2018). A-coa : an
adaptive cuckoo optimization algorithm for conti-
nuous and combinatorial optimization. In Neural
Computing and Applications, pages 1–25.
CHEN and al. (2018). Emotion recognition using empiri-
cal mode decomposition and approximation entropy.
Computers and Electrical Engineering, 72 :383–392.
CHEN, Y. and XIAO, D. (1999). Fuzzy identification and
control algorithms based on an etsk model. 14th Trien-
nial World Congress, 32 :5456–5461.
DASS, A. and SRIVASTAVA, S. (2018). Identification and
control of dynamical systems using different architec-
tures of recurrent fuzzy system. ISA Transanctions.
ELMZABI, A. (2005). Une approche adaptative basée sur
le clustering flou : Outil d’aide à la gestion intelli-
gente du réseau. PhD thesis, Faculté des Sciences et
Techniques Mohammedia, Maroc.
HAIDI and al. (2018). Feature selection based on artificial
bee colony and gradient boosting decision tree. In Ap-
plied Soft Computing, volume 74, pages 634–642.
HASSABIS and al. (2017). Neuroscience-inspired artificial
intelligence. Neuron 95, pages 245–258.
HURRAH and al. (2019). Dual watermarking framework
for privacy protection and content authentication of
multimedia. Future Generation Computer Systems,
94 :654–667.
IHUEZE, C. and ONWURAH, U. (2018). Road traffic ac-
cidents prediction modelling : An analysis of anam-
bra state, nigeria. Accident Analysis and Prevention,
pages 21–29.
IQDOUR, R. (2006). Modélisation des séries temporelles
par les systèmes flous et les réseaux de neurones :
application à la prédiction des processus météorolo-
giques. PhD thesis, Faculté des Sciences Semlalia,
Maroc.
ISHIBUSHI and al. (1995). Selecting fuzzy if-then rules
for classification problems using genetic algorithms.
IEEE Transactions on Fuzzy Systems, 3 :260–270.
Application of Artificial Intelligence Approach for Optimizing Management of Road Traffic
9