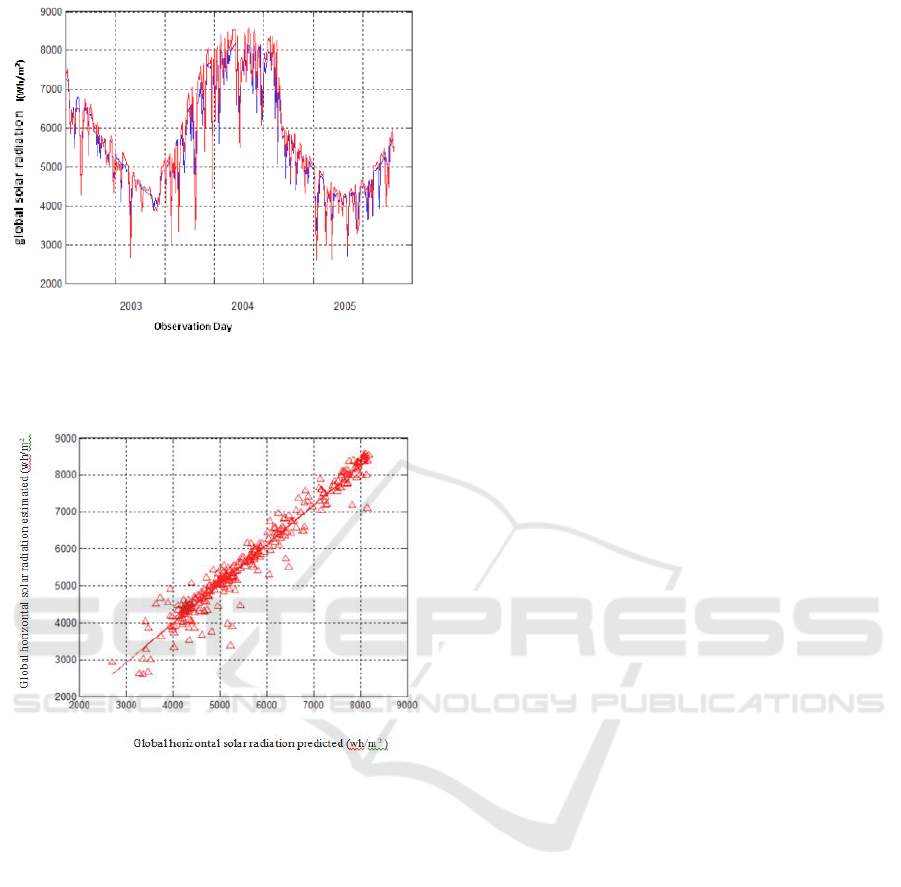
Figure 7: Global horizontal solar radiation estimated from
a sunshine duration of the 2003-2005 period, in red the
desired outputs, in blue the predicted outputs (simulated).
Figure 8: The correlation between the desired outputs and
predicted outputs of global horizontal solar radiation.
The function represents an approximation of the
correlation between predicted and desired outputs;
according to the data used the coefficient is
approximately 0.78 so make improvements on the
model to get better results.
6 CONCLUSIONS
In our study we were interested in the neural
network prediction method, in particular the multi-
layer perceptron method.
For learning has used the Levenberg-Marquardt
algorithm to calculate the approximation weights.
For this network the inputs propagate to the output
without return.
For the learning used the database 2000-2003,
for the test used the data of 2003-2005, the
simulation with these databases gives results of
correlation coefficient equal 0.81651for learning;
and 0.76259 for validation. According to the
correlation graphs between the desired and predicted
outputs on the one hand, and the mean square error
on the other, we can use this neural model to
estimate daily global solar irradiations.
Improving the model with the use of data from the
Adrar URERMS research unit station remains a
work of the future.
REFERENCES
Angstrom, A., 1924. Solar and terrestrial radiation, Quart
J Roy Met Soc, 50:121–5.
Azadeh, A., Maghsoudi, A., Sohrabkhani, S., 2009 An
integrated artificial neural networks approach for
predicting global radiation, Energy Conversion and
Management 50.
CYRIL v., 2011. Prédiction de séries temporelles de
rayonnement solaire global et de production d’énergie
photovoltaïque à partir de réseaux de neurones
artificiels, Université de corse-pascal paoli. Thèse
doctorat
Duffie JA, Beckman WA. Solar engineering of thermal
process. New York: Wiley; 1991.
Mellit, A., 2008. Artificial Intelligence technique for
modelling and forecasting of solar radiation data, Int.
J. Art. Int and Soft Computing, Vol. 1, No. 1.
Mellit, A., Benghanem, M., Hadj Arab, c, A., Guessoum,
d. 2005 A simplified model for generating sequences
of global solar radiation data for isolated sites :Using
artificial neural network and a library of Markov
transition matrices approach, Solar Energy 79 469–
482.
Mohandes, M., Rehman, S., and Halawani, T. O., 1998.
Estimation of global solar radiation using artificial
neural networks, Renewable Energy, Vol. 14.
Mubiru, J., Banda, E. J. K. B., 2008. Estimation of
monthly average daily global solar irradiation using
artificial neural networks, Solar Energy, 82.
Prescott, JA., 1940. Evaporation from water surface in
relation to solar radiation. Trans Roy Soc Austrn
46:114–8.
Page, JK., 1961.The estimation of monthly mean values of
daily total short wave radiation on vertical and
inclined surfaces from sunshine records for latitudes
40N–40S. In: Proceedings of UN conference on new
sources of energy, 78–90.
Rahimikhoob, A., 2010. Estimating global solar radiation
using artificial neural network and air temperature data
in a semi-arid environment, Renewable Energy 35.
ICCSRE 2018 - International Conference of Computer Science and Renewable Energies
408