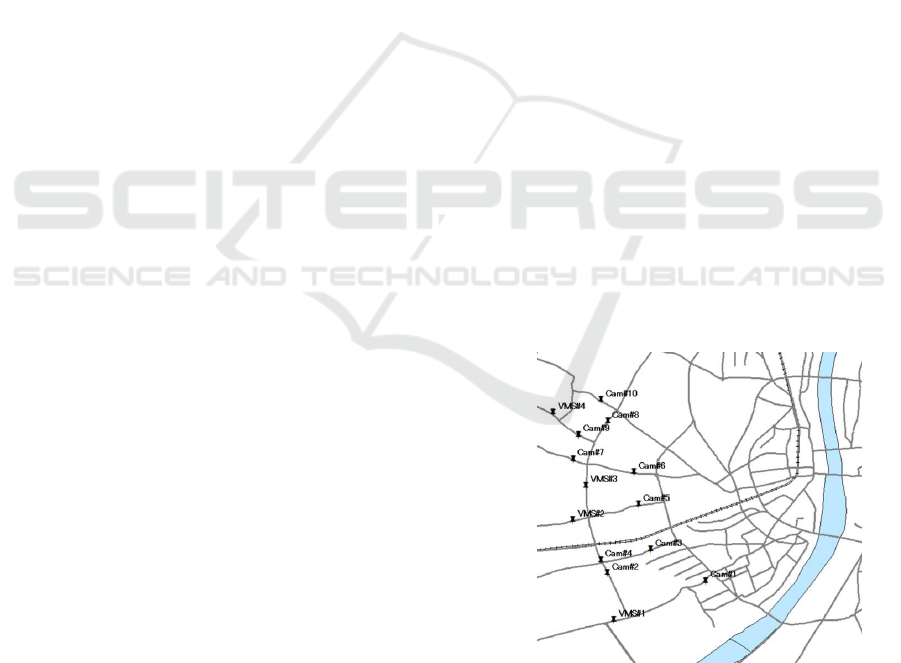
theory. Therefore the traffic condition under less than
0.5 V.R. area means congested condition. The V.R.
becomes important parameter for congestion
condition of the roads.
As the third step, we investigate lane effect
against each traffic condition and this analysis is main
study in this paper. In order to analyze the lane effect
to traffic congestion, we compare the variance of
traffic congestion between driving lane and passing
lane. In this study, we have three measured data of the
roads—speed (v), traffic density (k), and traffic
volume (q). For the traffic condition variance, two
value of them are enough for the variance comparison
because there is the relationship among them—
q=k×v. So we chose (v, k) parameter set as
representative traffic characteristics for each lane.
Since the V.R. is congested condition parameter, the
correlation between V.R. and the (v, k) parameter set
of each lane provides lane effectiveness for its traffic
condition.
And finally we show a certain lane effect between
driving lane and passing lane by K-Q curve of each
lane.
This is the first traffic flow analysis in emerging
country in terms of the following features.
Use one month traffic flow big data among 10
locations
Introduce traffic flow equation by EO method
Show lane effectiveness by K-Q curve
characteristics
1.2 Related Study
In terms of traffic analysis, there are new challenges
by using advanced sensing technology such as the
probing data with Global Positioning System (GPS)
in side vehicles. This study is estimation by using
probing vehicle behaviour but this case study is
limited number of probe data and a study in the
advanced country i.e. Italy. For probing technology
based traffic analysis, there are many case studies in
the vehicular ad hoc network (VANET) environment.
These research are useful to estimation traffic safety
application especially in the congested traffic
condition. In VANET environment, the advanced
communication technology is sued such as Dedicated
Short Radio Communication (DSRC), Cellular phone
network like Long term Evolution (LTE), 3G, 4G, 5G
etc. Most of the advanced network communication
technology has just been released in the advanced
countries and will be installed in new manufacturing
vehicles in future.
Therefore the classical traffic monitoring technology
with monitoring cameras is still valid especially for
study of traffic flow analysis in the emerging
countries. In case of traffic analysis in emerging
countries such as India, there are several studies these
days. Goutham.M has proving data analysis at
National Highway in Hyderabad. It shows trend of
traffic condition and comparison with Indian Road
standard IRC-106-1990 but measurement points are
only two Highways and volume is two days with five
CCTVs. In Salim.A et al study, it describes traffic
congestion condition by headway measurement in
Chennai. But measurement point is only one city road
and four days data with one hour for each. It is also
limited measurement data.
2 ENVIRONMENT OF TRAFFIC
DATA MEASUREMENT
2.1 Location
As mentioned in Introduction, the Indian ITS project
has been started since October 2014. The ITS
business operates in Ahmedabad city of Gujarat states
in India, where it is located west region of India and
it has about 8 million population. There are fourteen
traffic monitoring cameras which collect traffic
parameters such as traffic density, traffic volume, and
speed.
The Figure.1 shows total ITS cameras installation
location in the city. The “Cam” in Figure.1 means
Traffic Monitoring Camera and “VMS” means
Various Message Sign board. The main purpose of
VMS is display of traffic condition to drivers after
traffic condition analysis.
Figure 1: Indian ITS system installation location.
2.2 System Configuration
The Indian ITS system has three components—traffic
monitoring camera, traffic information board, traffic
Lane Effect of Traffic Flow Analysis in India
269