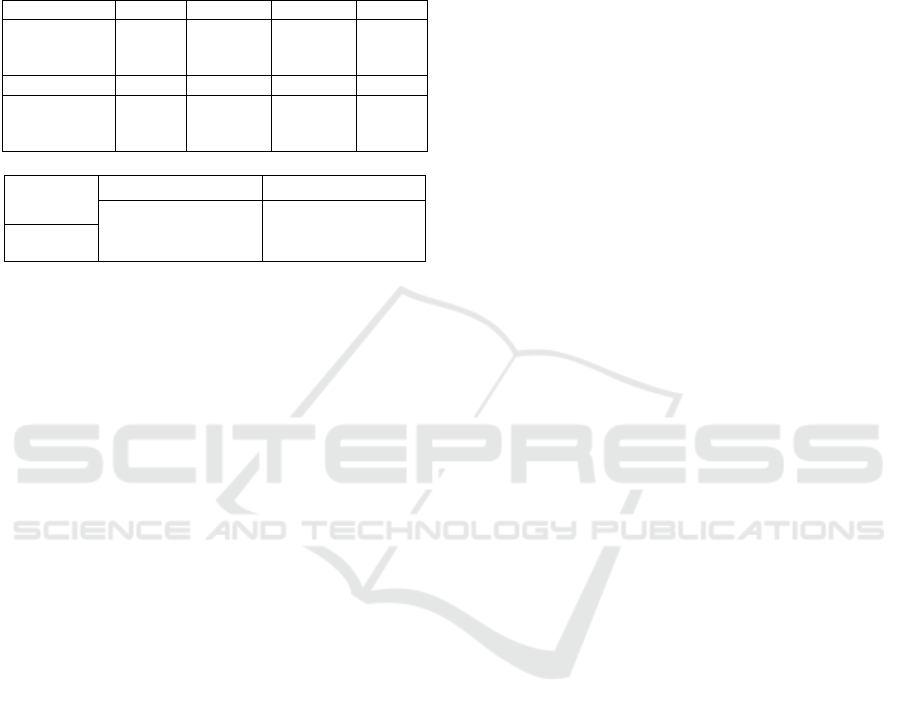
with over 98,3% accuracy. The results were obtained
by Intel® Optimization for TensorFlow. The LSTM
network was run on a gold Xeon processor showing
faster speed than on a 1080 nvidea graphics board in
30% under the same conditions.
Table 4: Classification of Voice Pathologies.
Parameters of
the glottal
signal
Glottal signal
parameters
and MFCCs
5 CONCLUSIONS
The aim of this work was the classification of two
voice diseases: nodule and unilateral paralysis and the
evaluation of the impact of parameters from the
glottal signal on this identification. Three different
classifiers have been used, to compare their
performance: an Artificial Neural Network, a Support
Vector Machine, LSTM and a Hidden Markov
Model.
From the results obtained, it can be verified that
glottal signal parameters are more relevant to
discriminate pathologies of the vocal folds than
MFCC’s, when they are evaluated individually. This
is the case even when the database is composed of
individuals with different genders and ages, providing
an average accuracy over 99%.
ACKNOWLEDGMENTS
This work was supported by Intel Corporation.
REFERENCES
Roy, N., Holt, K. I., Redmond, S., Muntz, H., 2007,
Behavioral characteristics of children with vocal fold
nodules. J Voice. 21(2):157-68.
Francis, D. O.; McKiever, M. E.; Garrett, G., Jacobson, B.;
Penson, D. F., 2014, Assessment of Patient Experience
with Unilateral Vocal Fold Immobility: A Preliminary
Study, Journal of voice 28 (5), 636-643.
Steffen, N., Pedrosa, V. V., Kazuo, R., Pontes, P., 2009,
Modifications of Vestibular Fold Shape from
Respiration to Phonation in Unilateral Vocal
FoldParalysis, Journal of Voice, Vol. 25, No. 1, pp.
111-113.
Behlau, M., Pontes, P. P., 1995, Avaliação e tratamento das
disfonias. São Paulo: Lovise, in unilateral vocal fold
paralysis, Journal of Voice 13(1):36-42.
Henrich, N., 2001, Étude de la source glottique en voix
parlée et chantée modelisation et estimation, mesures
acoustiques et electroglottographiques, perception,
Thèse de doctorat de l'Université Paris 6 (PhD Thesis).
Henrich, N., d'Alessandro, C., Doval, B., Castellengo, M.
2005, Glottal Open quotient in singing: Measurements
and correlation with laryngeal mechanisms, vocal
intensity, and fundamental frequency, Journal of the
Acoustical Society of America 117(3), pp 1417-1430.
Mendonza, L., Vellasco, M., Cataldo, E., 2014,
Classification of Vocal Aging Using Parameters
Extracted From the Glottal Signal J Voice. 21(2):157-
68.
Software Aparat, http://aparat.sourceforge.net/index.php/
Main_Page, Helsinki University of Technology
Laboratory of Acoustics and Audio Signal Processing.).
Rosa, I. S., 2005, Análise acústica da voz de indivíduos na
terceira idade, Tese de mestrado Universidade de São
Paulo, São Carlos (in portuguese).
Londoño, J., Llorente, J., 2010, An improved method for
voice pathology detection by means of a HMM-based
feature space transformation Pattern Recognition,
Volume 43, Issue 9, September 2010.
Wang, X., Zhang, J., Yan, Y., 2009, Glottal Source
biometrical signature for voice pathology detection,
Speech Communication, 51 759-781.
Hariharan, M., Paulraj, M. P., Yaacob, S., 2009,
Identification of vocal fold pathology based on Mel
Frequency Band Energy Coefficients and singular
value decomposition Signal and Image Processing
Applications (ICSIPA), volume 514 – 517.
Rosa, M. D. O., Pereira, J. C., Grellet, M., 2000, Adaptive
Estimation of Residue Signal for Voice Pathology
Diagnosis, IEEE Trans. Biomedical Eng., Vol. 47, No.
1, Jan. 2000.
Pulakka H., 2005, Analysis of Human Voice Production
Using Inverse Filtering, High-Speed Imaging, and
Electroglottograph,. University of Technology
Helsinki.
Software Praat, http://www.fon.hum.uva.nl/praat/,
University of Amsterdam.
ICAART 2019 - 11th International Conference on Agents and Artificial Intelligence
28