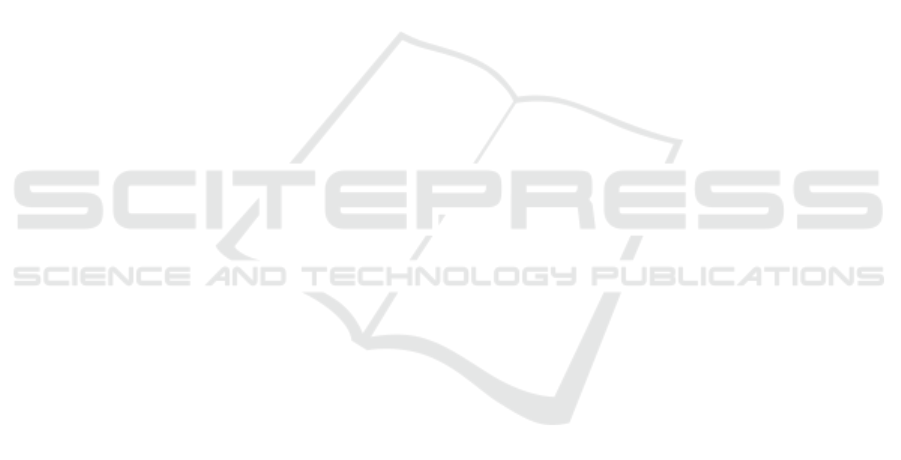
probability that the public signal suggests the
“correct” course for the decision becomes higher.
The main premise of the model, therefore, is that
the public signal will almost always prove stronger
and influence the decision even when the private
signal initially informed a contrasting course.
Information cascades rely on four prerequisites
for the model to be applied to a situation (Easley and
Kleinberg, 2010):
1) There is a (binary/contrasting) decision to be
made;
2) Each person has a private signal that originally
informs their initial proclivity;
3) The decisions that were made by others can be
observed and the decisions happen sequentially;
and
4) The private information of others is not known to
the individual.
However, inferences can be made about
another’s private information based on their publicly
noticeable decision from number 3 above.
Take, for instance, an example of adopting new
technology. An individual, let us call him John, is
looking for a new digital device and has an
expectation of the utility (gain) that the new
technology will have to him. He reads an online
review of the device which indicates that the
technology is in its infancy and it might be better to
wait for revisions, rather than adopt the technology
in its current form. John then forms a negative,
personal signal because of the perceived lack of
utility. The information that the signal relays
prevents him from adopting the technology.
As stated before, John is not in isolation. He
moves around in public spaces and notices that
another person has one of the devices in their
possession. He has, however, no sense of whether
the other person is happy with the purchase (i.e. the
decision to adopt was the correct decision to make),
and he does not know what the private signal of the
other person was that convinced him/her to adopt the
technology. Instead, John receives a positive public
signal that indicates that adoption of the technology
has happened. As he encounters and sees more
people that have adopted the technology, the higher
the probability becomes that adoption, rather than
rejection, is preferable and the signal becomes
stronger.
As soon as the public signal becomes stronger
than the private and overcomes the associated risk
with adoption, John will make the decision to follow
the group of adopters and adopt the technology. The
prerequisites for an information cascade, mentioned
above, have been met and an information cascade has
taken place.
An example of how information cascades can be
evaluated and, possibly, predicted is the application
of behavioural threshold analysis. This is discussed
in the following sub-section.
2.2 Behavioural Threshold Analysis
From the brief description in Section 2.1, it is clear
that information cascades and sequential decision-
making may play a significant role in information
security behaviour. However, to do a meaningful
evaluation thereof, in a way that provides new
insights into information security behaviour and
specifically paradoxical information security
behaviour, an acceptable method for analysing
information cascades should be found. One possible
approach is to use behavioural threshold analysis
which is also based on sequential decision-making.
In this section, behavioural threshold analysis is
introduced briefly as a possible instrument to
evaluate information cascades. Parallels are also
drawn between information cascades and
behavioural threshold analysis to support the idea of
behavioural threshold analysis as an evaluation
instrument for information cascades and,
specifically, sequential decision-making.
The notion of behavioural threshold analysis pre-
dates the conceptualisation of information cascades
by some time. Behavioural threshold analysis was
first developed by Granovetter (1978) and was
coined “threshold models of collective behaviour”.
The premise supporting the model of threshold
analysis, is that the behaviour of an individual
(Sarah) in a group setting will be influenced by the
group’s behaviour if a large enough number of other
members of the group exhibit a specific behaviour.
The number of others that must perform a behaviour
before Sarah joins in, is determined by her inherent
threshold for participation. If the number of others in
the group that perform a certain action, exceeds
Sarah’s threshold for participation, she will follow
the group and also perform the action. To
demonstrate the similarities between information
cascades and behavioural threshold analysis, an
example of behavioural threshold analysis (in terms
of information security behaviour) is presented.
Information cascades, in information security
behaviour, can manifest itself when employees in a
group setting, follow the security behaviour of
others rather than relying on their own knowledge.
This is similar for behavioural thresholds:
Information security awareness training is often
conducted in organisations to educate employees on
Theorising on Information Cascades and Sequential Decision-making for Analysing Security Behaviour
207