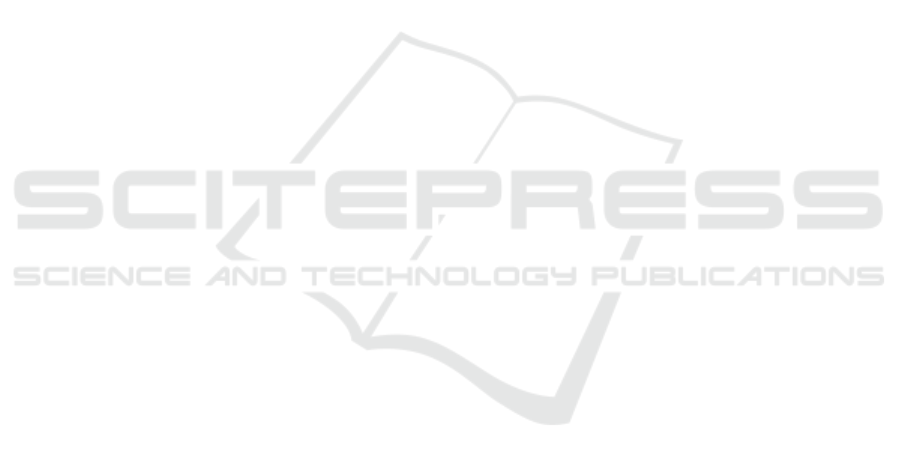
successfully in order that it can accurately match a
given signal with the corresponding physical
activity. This study constructed a general
predictable model that takes advantage of the
signals generated by the whole dataset population
(60 participants).
6 CONCLUSIONS
The findings of this study provide evidence that it is
possible to identify an individual’s physical activity
with a high degree of accuracy, reaching nearly 98%,
based on smartphone-embedded gyroscope and
accelerometer sensor signals gathered over two days.
This was achieved by leveraging the capabilities of
machine learning algorithms in two stages: feature
ranking, in which the feature space is ranked based on
the multiclass classification approach, followed by
activity identification, in which only top-ranked
features are included within the classification phase.
The soft majority voting approach provides the
highest accuracy in comparison with other models,
such as single classifier or hard majority voting.
REFERENCES
Al-Naffakh, N. et al. (2016) ‘Activity Recognition using
wearable computing’, in 2016 11th International
Conference for Internet Technology and Secured
Transactions (ICITST). IEEE, pp. 189–195. doi:
10.1109/ICITST.2016.7856695.
Altun, K., Barshan, B. and Tunçel, O. (2010) ‘Comparative
study on classifying human activities with miniature
inertial and magnetic sensors’, Pattern Recognition,
43(10), pp. 3605–3620. doi: 10.1016/j.patcog.2010.
04.019.
Anguita, D. et al. (2012) ‘Human Activity Recognition on
Smartphones Using a Multiclass Hardware-Friendly
Support Vector Machine’, in Lecture Notes in Computer
Science (including subseries Lecture Notes in Artificial
Intelligence and Lecture Notes in Bioinformatics), pp.
216–223. doi: 10.1007/978-3-642-35395-6_30.
Antos, S. A., Albert, M. V. and Kording, K. P. (2014) ‘Hand,
belt, pocket or bag: Practical activity tracking with
mobile phones’, Journal of Neuroscience Methods, 231,
pp. 22–30. doi: 10.1016/j.jneumeth.2013.09.015.
Bahle, G. et al. (2014) ‘Recognizing Hospital Care
Activities with a Coat Pocket Worn Smartphone’, in
Proceedings of the 6th International Conference on
Mobile Computing, Applications and Services. ICST.
doi: 10.4108/icst.mobicase.2014.257777.
Bayat, A., Pomplun, M. and Tran, D. A. (2014) ‘A study on
human activity recognition using accelerometer data
from smartphones’, Procedia Computer Science.
Elsevier Masson SAS, 34(C), pp. 450–457. doi:
10.1016/j.procs.2014.07.009.
Bhanu Jyothi, K. and Hima Bindu, K. (2018) ‘A Case Study
in R to Recognize Human Activity Using Smartphones’,
in, pp. 191–200. doi: 10.1007/978-981-10-6319-0_17.
Bieber, G. et al. (2011) ‘The hearing trousers pocket’, in
Proceedings of the 4th International Conference on
PErvasive Technologies Related to Assistive
Environments - PETRA ’11. New York, New York,
USA: ACM Press, p. 1. doi: 10.1145/2141622.2141674.
Bro, R. and Smilde, A. K. (2014) ‘Principal component
analysis’, Analytical Methods, 6(9), pp. 2812–2831. doi:
10.1039/c3ay41907j.
Capela, N. A. et al. (2016) ‘Evaluation of a smartphone
human activity recognition application with able-bodied
and stroke participants’, Journal of NeuroEngineering
and Rehabilitation, 13(1), p. 5. doi: 10.1186/s12984-
016-0114-0.
Derawi, M. and Bours, P. (2013) ‘Gait and activity
recognition using commercial phones’, Computers &
Security, 39, pp. 137–144. doi: 10.1016/j.cose.2013.07.
004.
F, A. (no date) AndroSensor. Available at: https://
play.google.com/store/apps/details?id=com.fivasim.an
drosensor&hl=en_GB (Accessed: 14 August 2018).
Ganti, R. K., Srinivasan, S. and Gacic, A. (2010)
‘Multisensor Fusion in Smartphones for Lifestyle
Monitoring’, in 2010 International Conference on Body
Sensor Networks. IEEE, pp. 36–43. doi: 10.1109/
BSN.2010.10.
Goodall, C. and Jolliffe, I. T. (2002) Principal Component
Analysis. John Wiley & Sons, Ltd. Available at:
http://onlinelibrary.wiley.com/doi/10.1002/047001319
2.bsa501/full.
Ha, S. and Choi, S. (2016) ‘Convolutional neural networks
for human activity recognition using multiple
accelerometer and gyroscope sensors’, in 2016
International Joint Conference on Neural Networks
(IJCNN). IEEE, pp. 381–388. doi: 10.1109/
IJCNN.2016.7727224.
Hamm, J. et al. (2013) ‘Automatic Annotation of Daily
Activity from Smartphone-Based Multisensory
Streams’, in, pp. 328–342. doi: 10.1007/978-3-642-
36632-1_19.
He, Y. and Li, Y. (2013) ‘Physical Activity Recognition
Utilizing the Built-In Kinematic Sensors of a
Smartphone’, International Journal of Distributed
Sensor Networks, 9(4), p. 481580. doi: 10.1155/
2013/481580.
Heng, X., Wang, Z. and Wang, J. (2016) ‘Human activity
recognition based on transformed accelerometer data
from a mobile phone’, International Journal of
Communication Systems, 29(13), pp. 1981–1991. doi:
10.1002/dac.2888.
Jiang, W. and Yin, Z. (2015) ‘Human Activity Recognition
Using Wearable Sensors by Deep Convolutional Neural
Networks’, in Proceedings of the 23rd ACM
international conference on Multimedia - MM ’15. New
York, New York, USA: ACM Press, pp. 1307–1310.
doi: 10.1145/2733373.2806333.
ICPRAM 2019 - 8th International Conference on Pattern Recognition Applications and Methods
350