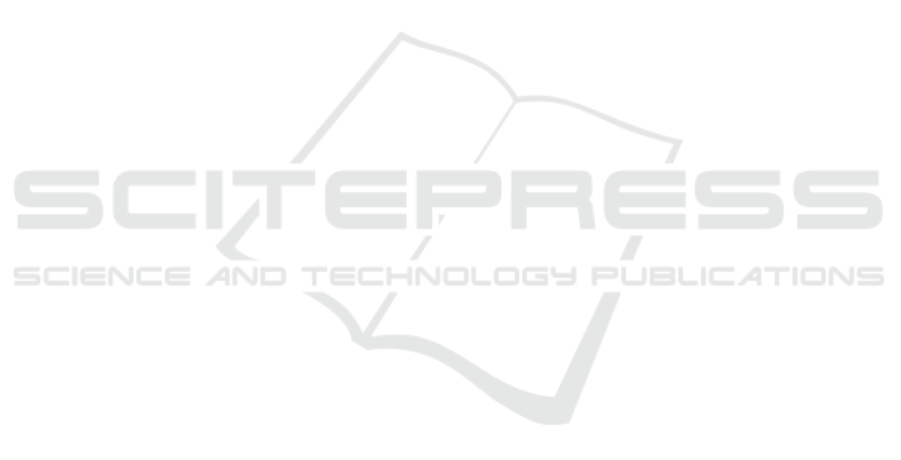
rithms have been evaluated.
We obtained a precision equal to 0.857 and a re-
call equal to 0.750 in the won match prediction, while
an average precision equal to 0.862 is obtained in the
number of goal prediction.
As future work, we plan to investigate whether the
proposed method is applicable to other sports like, for
instance, basketball or tennis. Furthermore, with the
aim to increase the obtained performances, we plan to
evaluate whether emerging deep learning algorithms
can be useful in order to detect match results with bet-
ter performances. Moreover, we plan to apply formal
methods (De Francesco et al., 2016; Santone et al.,
2013; Avvenuti et al., 2012) which have been already
been demonstrated to be effective in other domains,
like for example in biology (Ruvo et al., 2015).
REFERENCES
Arlot, S., Celisse, A., et al. (2010). A survey of cross-
validation procedures for model selection. Statistics
surveys, 4:40–79.
Avvenuti, M., Bernardeschi, C., De Francesco, N., and
Masci, P. (2012). JCSI: A tool for checking secure
information flow in java card applications. Journal of
Systems and Software, 85(11):2479–2493.
Baboota, R. and Kaur, H. (2018). Predictive analysis and
modelling football results using machine learning ap-
proach for english premier league. International Jour-
nal of Forecasting.
Carbonell, J. G., Michalski, R. S., and Mitchell, T. M.
(1983). An overview of machine learning. In Machine
Learning, Volume I, pages 3–23. Elsevier.
De Francesco, N., Lettieri, G., Santone, A., and Vaglini,
G. (2016). Heuristic search for equivalence checking.
Software and Systems Modeling, 15(2):513–530.
Dijksterhuis, A., Bos, M. W., Van der Leij, A., and
Van Baaren, R. B. (2009). Predicting soccer matches
after unconscious and conscious thought as a function
of expertise. Psychological Science, 20(11):1381–
1387.
Gomes, J., Portela, F., and Santos, M. F. (2015). Deci-
sion support system for predicting football game re-
sult. In Computers-19th International Conference on
Circuits, Systems, Communications and Computers-
Intelligent Systems and Applications Special Sessions.
Series, volume 32, pages 348–353.
Jordan, M. I. and Mitchell, T. M. (2015). Machine learn-
ing: Trends, perspectives, and prospects. Science,
349(6245):255–260.
Joseph, A., Fenton, N. E., and Neil, M. (2006). Predict-
ing football results using bayesian nets and other ma-
chine learning techniques. Knowledge-Based Systems,
19(7):544–553.
Kumar, G. (2013). Machine learning for soccer analytics.
Liti, C., Piccialli, V., and Sciandrone, M. (2017). Predict-
ing soccer match outcome using machine learning al-
gorithms. In Proceedings of MathSport International
2017 Conference, page 229.
Lohr, S. (2012). The age of big data. New York Times,
11(2012).
Lucey, P., Bialkowski, A., Monfort, M., Carr, P., and
Matthews, I. (2014). quality vs quantity: Improved
shot prediction in soccer using strategic features from
spatiotemporal data. In Proc. 8th annual mit sloan
sports analytics conference, pages 1–9.
Michalski, R. S., Carbonell, J. G., and Mitchell, T. M.
(2013). Machine learning: An artificial intelligence
approach. Springer Science & Business Media.
Nasrabadi, N. M. (2007). Pattern recognition and ma-
chine learning. Journal of electronic imaging,
16(4):049901.
Razali, M., Yatim, and Aziz, A. (2017). Predicting football
matches results using bayesian networks for english
premier league (epl). International Research and In-
novation Summit.
Rein, R. and Memmert, D. (2016). Big data and tactical
analysis in elite soccer: future challenges and oppor-
tunities for sports science. SpringerPlus, 5(1):1410.
Ruvo, G., Nardone, V., Santone, A., Ceccarelli, M., and
Cerulo, L. (2015). Infer gene regulatory networks
from time series data with probabilistic model check-
ing. pages 26–32.
Santone, A., Vaglini, G., and Villani, M. (2013). Incremen-
tal construction of systems: An efficient characteriza-
tion of the lacking sub-system. Science of Computer
Programming, 78(9):1346–1367.
Sathe, Kasat, K. and Satao (2017). Predictive analysis
of premier league using machine learning. Interna-
tional Journal of Innovative Research in Computer
and Communication Engineering.
Shaikh, Z. A., Khan, U. A., Rajput, M. A., and Memon,
A. W. (2016). Machine learning based number
plate detection and recognition. In Proceedings of
the 5th International Conference on Pattern Recog-
nition Applications and Methods, pages 327–333.
SCITEPRESS-Science and Technology Publications,
Lda.
Silva, D., Aidos, H., and Fred, A. L. (2016). Efficient ev-
idence accumulation clustering for large datasets. In
ICPRAM, pages 367–374.
Wu, X., Kumar, V., Quinlan, J. R., Ghosh, J., Yang, Q.,
Motoda, H., McLachlan, G. J., Ng, A., Liu, B., Philip,
S. Y., et al. (2008). Top 10 algorithms in data mining.
Knowledge and information systems, 14(1):1–37.
Can Machine Learning Predict Soccer Match Results?
465