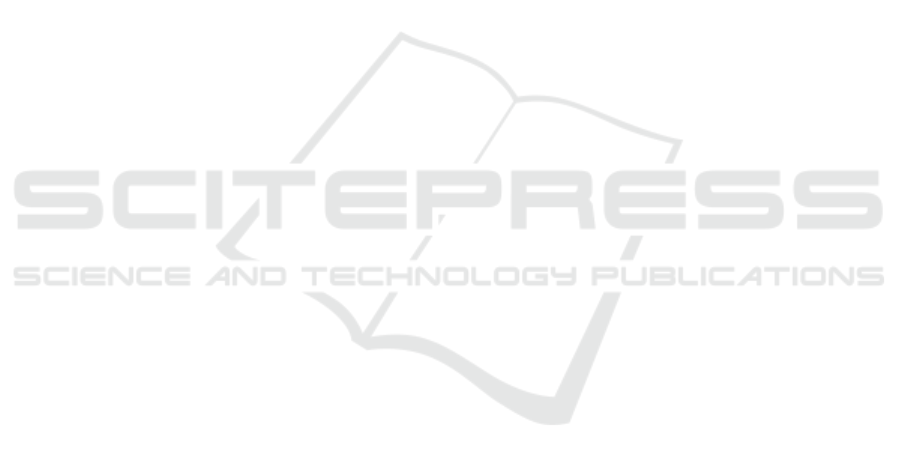
error, but constraint by the limited amount of data the
”time delay” phenomenon is not completely elimina-
ted.
REFERENCES
Bi, H., Liang, H., and Wang, W. (2009). Resampling
method and machine learning. Journal of Computer,
32(5):862–877.
Bontempi, G., Taieb, S. B., and Le Borgne, Y.-A. (2012).
Machine learning strategies for time series forecas-
ting. In European Business Intelligence Summer
School, pages 62–77. Springer.
Clark, P. A., Harcourt, S. A., Macpherson, B., Mathison,
C. T., Cusack, S., and Naylor, M. (2008). Prediction of
visibility and aerosol within the operational met office
unified model. i: Model formulation and variational
assimilation. Quarterly Journal of the Royal Meteo-
rological Society, 134(636):1801–1816.
Cortez, P., Rio, M., Rocha, M., and Sousa, P. (2006). Inter-
net traffic forecasting using neural networks. In Neu-
ral Networks, 2006. IJCNN’06. International Joint
Conference on, pages 2635–2642. IEEE.
Dietterich, T. G. (2002). Machine learning for sequential
data: A review. In Joint IAPR International Works-
hops on Statistical Techniques in Pattern Recognition
(SPR) and Structural and Syntactic Pattern Recogni-
tion (SSPR), pages 15–30. Springer.
Dietz, S. J., Kneringer, P., Mayr, G. J., and Zeileis, A.
(2017). Forecasting low-visibility procedure states
with tree-based statistical methods. Pure and Applied
Geophysics, pages 1–14.
Fan, G., Ma, H., Zhang, X., and Liu, Y. (2016). Effects
of relative humidity and pm2.5 concentration on at-
mospheric visibility: a multi station comparative ana-
lysis based on hourly data. Journal of Meteorology,
74(6):959–973.
Fan, J., Li, Q., Hou, J., Feng, X., Karimian, H., and Lin,
S. (2017). A spatiotemporal prediction framework for
air pollution based on deep rnn. ISPRS Annals of the
Photogrammetry, Remote Sensing and Spatial Infor-
mation Sciences, 4:15.
Friedman, J., Hastie, T., and Tibshirani, R. (2001). The
elements of statistical learning. Springer series in sta-
tistics New York, NY, USA:.
Greff, K., Srivastava, R. K., Koutnik, J., Steunebrink, B. R.,
and Schmidhuber, J. (2016). Lstm: A search space
odyssey. IEEE Transactions on Neural Networks &
Learning Systems, 28(10):2222–2232.
Hochreiter, S. and Schmidhuber, J. (1997). Long short-term
memory. Neural Computation, 9(8):1735–1780.
James, G., Witten, D., Hastie, T., and Tibshirani, R. (2013).
An introduction to statistical learning, volume 112.
Springer.
Kurt, A. and Oktay, A. B. (2010). Forecasting air pollu-
tant indicator levels with geographic models 3 days in
advance using neural networks. Expert Systems with
Applications, 37(12):7986–7992.
Li, X., Peng, L., Yao, X., Cui, S., Hu, Y., You, C., and
Chi, T. (2017). Long short-term memory neural net-
work for air pollutant concentration predictions: Met-
hod development and evaluation. Environmental Pol-
lution, 231:997–1004.
Long, K., Li, C., Mao, X., and Hu, Y. (2017). Highway
foggy visibility prediction method. Journal of Xuzhou
Institute of Technology: Natural Sciences Edition, pa-
ges 31–37.
Minami, S. (2018). Predicting equity price with corporate
action events using lstm-rnn. Journal of Mathematical
Finance, 8(01):58.
Salman, A. G., Heryadi, Y., Abdurahman, E., and Suparta,
W. (2018). Weather forecasting using merged long
short-term memory model (lstm) and autoregressive
integrated moving average (arima) model. Journal of
Computer Science.
Simon, J. L. (1992). Resampling: the new statistics. arling-
ton, va: Resampling stats.
Song, M., Han, S., Zhang, M., Yao, Q., and Zhu, B. (2013).
Relationship between visibility and relative humidity
and pm10 and pm2.5 in tianjin. Journal of Meteoro-
logy and Environment, 29(2):34–41.
Wang, Q., Guo, Y., Yu, L., and Li, P. (2017). Eart-
hquake prediction based on spatio-temporal data mi-
ning: An LSTM network approach. IEEE Transacti-
ons on Emerging Topics in Computing, PP(99):1–1.
Wang, X., Han, J., Chen, J., Yan, W., and Yue, Y. (2016).
Variation characteristics of atmospheric visibility and
their relationship with relative humidity and particle
concentration in shijiazhuang of hebei. Journal of
Arid Meteorology, 34(4):648–655.
Yadav, A. P., Kumar, A., and Behera, L. (2013). Rnn ba-
sed solar radiation forecasting using adaptive learning
rate. In International Conference on Swarm, Evoluti-
onary, and Memetic Computing, pages 442–452.
Zhu, L., Zhu, G., Han, L., and Wang, N. (2017). The ap-
plication of deep learning in airport visibility forecast.
Atmospheric and Climate Sciences, 7(03):314.
Visibility Forecast for Airport Operations by LSTM Neural Network
473