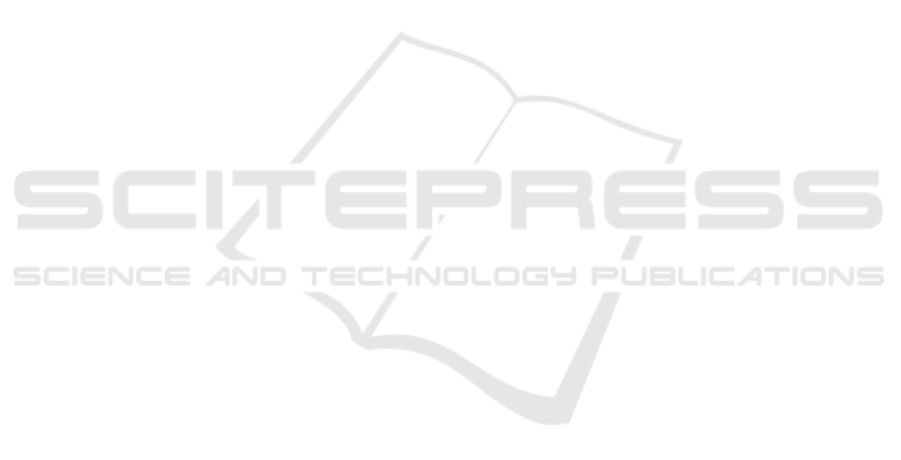
for the Target Bank Skill in a very small-scale study,
as that described above, suggests that it may also be
possible to generate adversarial utterances which are
effective against a more robust natural language un-
derstanding functionality, albeit that this is likely to
require larger scale experimental work.
4 CONCLUSIONS
Based on the small-scale experiment presented here,
we conclude that voice-controlled digital assistants
are potentially vulnerable to malicious input consist-
ing of nonsense syllables which humans perceive as
meaningless. A focus of future work might be to con-
duct a larger scale study and to conduct a more fine-
tuned analysis of successful and unsuccessful non-
sense attacks, to determine which nonsense syllables
are most likely to be confused with target commands
by machines, whilst still being perceived as nonsen-
sical by humans. This would enable investigation of
more targeted attacks. Ultimately the focus of future
work should be to consider how voice-controlled sys-
tems might be better trained to distinguish between
meaningful and meaningless sound with respect to the
language to which they are intended to respond.
Based on the proof-of-concept study presented
here, we further conclude that the natural language
understanding functionality in voice-controlled dig-
ital assistants is vulnerable to being misled by ad-
versarial utterances which trigger a target action by
the assistant, despite being unrelated to the action in
terms of the meaning of the utterance as understood
by humans. Future work should investigate the poten-
tial for attacks which are effective against a more ro-
bust natural language understanding functionality in
larger scale experimental work.
ACKNOWLEDGEMENTS
This work was funded by a doctoral training grant
from the UK Engineering and Physical Sciences Re-
search Council (EPSRC).
REFERENCES
Bailey, T. M. and Hahn, U. (2005). Phoneme similarity
and confusability. Journal of Memory and Language,
52(3):339–362.
Bispham, M. K., Agrafiotis, I., and Goldsmith, M. (2018).
A taxonomy of attacks via the speech interface. ac-
cepted for publication by the The Third Interna-
tional Conference on Cyber-Technologies and Cyber-
Systems.
Carlini, N., Mishra, P., Vaidya, T., Zhang, Y., Sherr, M.,
Shields, C., Wagner, D., and Zhou, W. (2016). Hid-
den voice commands. In 25th USENIX Security Sym-
posium (USENIX Security 16), Austin, TX.
Carlini, N. and Wagner, D. (2018). Audio adversarial ex-
amples: Targeted attacks on speech-to-text. arXiv
preprint arXiv:1801.01944.
Kollar, T., Berry, D., Stuart, L., Owczarzak, K., Chung, T.,
Mathias, L., Kayser, M., Snow, B., and Matsoukas,
S. (2018). The Alexa Meaning Representation Lan-
guage. In Proceedings of the 2018 Conference of the
North American Chapter of the Association for Com-
putational Linguistics: Human Language Technolo-
gies, Volume 3 (Industry Papers), volume 3, pages
177–184.
Kumar, A., Gupta, A., Chan, J., Tucker, S., Hoffmeister,
B., and Dreyer, M. (2017). Just ASK: Building an ar-
chitecture for extensible self-service spoken language
understanding. arXiv preprint arXiv:1711.00549.
Kumar, D., Paccagnella, R., Murley, P., Hennenfent, E.,
Mason, J., Bates, A., and Bailey, M. (2018). Skill
squatting attacks on Amazon Alexa. In 27th USENIX
Security Symposium (USENIX Security 18), pages 33–
47, Baltimore, MD. USENIX Association.
Lippmann, R. P. et al. (1997). Speech recognition by ma-
chines and humans. Speech communication, 22(1):1–
15.
McCurdy, N., Srikumar, V., and Meyer, M. (2015).
Rhymedesign: A tool for analyzing sonic devices in
poetry. In Proceedings of the Fourth Workshop on
Computational Linguistics for Literature, pages 12–
22.
McTear, M., Callejas, Z., and Griol, D. (2016). The conver-
sational interface. Springer.
Meutzner, H., Gupta, S., and Kolossa, D. (2015). Con-
structing secure audio captchas by exploiting differ-
ences between humans and machines. In Proceedings
of the 33rd annual ACM conference on human factors
in computing systems, pages 2335–2338. ACM.
Miller, G. A. (1995). WordNet: a lexical database for En-
glish. Communications of the ACM, 38(11):39–41.
Nowak, M. A. and Krakauer, D. C. (1999). The evolution
of language. Proceedings of the National Academy of
Sciences, 96(14):8028–8033.
Papernot, N., McDaniel, P., Swami, A., and Harang, R.
(2016). Crafting adversarial input sequences for re-
current neural networks. In Military Communications
Conference, MILCOM 2016-2016 IEEE, pages 49–
54. IEEE.
Roberts, A. C., Wetterlin, A., and Lahiri, A. (2013). Align-
ing mispronounced words to meaning: Evidence from
ERP and reaction time studies. The Mental Lexicon,
8(2):140–163.
Scharenborg, O. and Cooke, M. (2008). Comparing hu-
man and machine recognition performance on a VCV
corpus. Proceedings of the ISCA Tutorial and Re-
search Workshop on Speech Analysis and Processing
for Knowledge Discovery.
ICISSP 2019 - 5th International Conference on Information Systems Security and Privacy
86