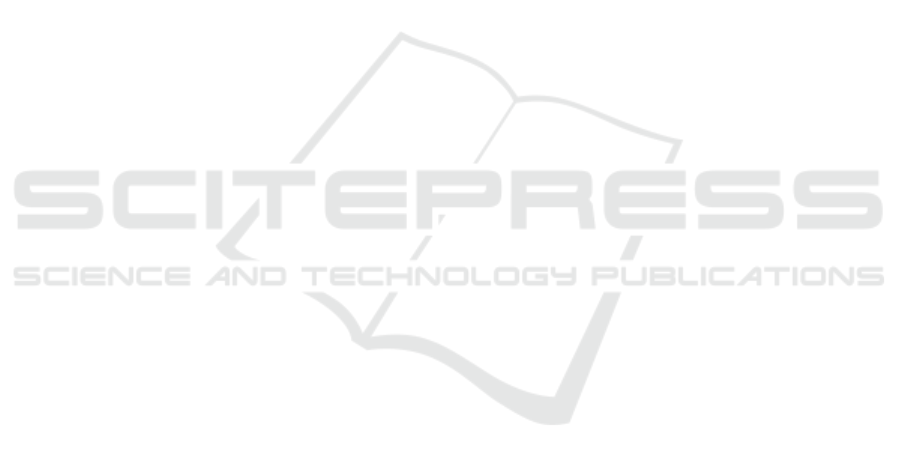
6 CONCLUSION
Previous studies addressed precast production
planning by using either mathematical programming
methods or simulation models. However, the
uncertainty of processing times when determining
optimum PCs schedules to achieve on-time delivery
of PCs was seldom addressed. To fill this gap, a
simulation-based optimization approach is developed
in which a discrete event simulation model was
developed by using Arena
®
software based on precast
flow shop sequencing formulation. Then, the
developed model is linked with OptQuest
®
(an
optimization package) to search for optimum PCs
sequences that minimize deviation from the
contracted due dates of PCs. Thereafter, the proposed
approach was validated by comparing its results with
a published approach from literature. To test its
practicality, the developed approach was applied on a
case study with the objective of minimizing the
tardiness and earliness penalty costs. The obtained
results indicated that the optimum sequence can save
about 15% of penalty costs in comparison with the
results of a heuristic rule.
In future work, multi-objective function to
minimize both the penalty cost and production costs
can be applied while considering other realistic
features of the precast production such as buffer space
between production stages and multiple production
lines. However, the computation time will be longer
due to the complexity of the simulation model. This
might call for using other simulation optimization
methods such as the response surface methodology to
reduce the time needed to make urgent operational
decisions in precast plants such as PCs sequencing.
REFERENCES
Al-Bazi, A., and Dawood, N., 2018. Simulation-based
optimisation using simulated annealing for crew
allocation in the precast industry. Architectural
Engineering and Design Management, 14(1–2), 109–
126. https://doi.org/10.1080/17452007.2017.1313721
Arashpour, M., Wakefield, R., Abbasi, B., Lee, E. W. M.,
and Minas, J., 2016. Off-site construction optimization:
Sequencing multiple job classes with time constraints.
Automation in Construction, 71(Part 2), 262–270.
https://doi.org/10.1016/j.autcon.2016.08.001
Benjaoran, V., Dawood, N., and Hobbs, B., 2005. Flowshop
scheduling model for bespoke precast concrete
production planning. Construction Management and
Economics, 23(1), 93–105.
https://doi.org/10.1080/0144619042000287732
Bradley, A., 2007. OptQuest for Arena User’s Guide. RS
Inc., Ed., Ed.
Chan, W.-T., and Hu, H., 2001. An application of genetic
algorithms to precast production scheduling.
Computers and Structures, 79(17), 1605–1616.
Chan, W. T., and Hu, H., 2002. Constraint programming
approach to precast production scheduling. Journal of
Construction Engineering and Management, 128(6),
513–521.
Chen, J. H., Yang, L. R., and Tai, H. W., 2016. Process
reengineering and improvement for building precast
production. Automation in Construction, 68, 249–258.
https://doi.org/10.1016/j.autcon.2016.05.015
Cheng, T. M., and Yan, R. Z., 2009. Integrating messy
genetic algorithms and simulation to optimize resource
utilization. Computer-Aided Civil and Infrastructure
Engineering, 24(6), 401–415.
https://doi.org/10.1111/j.1467-8667.2008.00588.x
Hu, H., 2007. A study of resource planning for precast
production. Architectural Science Review, 50(2), 106–
114. https://doi.org/10.3763/asre.2007.5016
Ko, C. H., and Wang, S. F., 2011. Precast production
scheduling using multi-objective genetic algorithms.
Expert Systems with Applications, 38(7), 8293–8302.
https://doi.org/10.1016/j.eswa.2011.01.013
Laguna, M., and Marti, R., 2003. The OptQuest callable
library. In Optimization Software Class Libraries (pp.
193–218). Springer.
Law, A. M., 2007. Simulation modeling and analysis,
McGraw-Hill New York, 4
th
edition.
Sacks, R., Eastman, C. M., and Lee, G., 2004. Process
model perspectives on management and engineering
procedures in the precast/prestressed concrete industry.
Journal of Construction Engineering and Management,
130(2), 206–215.
Wang, Z., and Hu, H., 2017. Improved Precast Production
– Scheduling Model Considering the Whole Supply
Chain. Journal of Computing in Civil Engineering,
31(4), 1–12. https://doi.org/10.1061/(ASCE)CP.1943-
5487.0000667.
Wang, Z., Hu, H., and Gong, J., 2018a. Framework for
modeling operational uncertainty to optimize offsite
production scheduling of precast components.
Automation in Construction, 86(April 2017), 69–80.
https://doi.org/10.1016/j.autcon.2017.10.026
Wang, Z., Hu, H., and Gong, J., 2018b. Simulation based
multiple disturbances evaluation in the precast supply
chain for improved disturbance prevention. Journal of
Cleaner Production, 177(March), 232–244.
https://doi.org/10.1016/j.jclepro.2017.12.188
Yang, Z., Ma, Z., and Wu, S., 2016. Optimized flowshop
scheduling of multiple production lines for precast
production. Automation in Construction, 72, 321–329.
https://doi.org/10.1016/j.autcon.2016.08.021
Yenisey, M. M., and Yagmahan, B., 2014. Multi-objective
permutation flow shop scheduling problem: Literature
review, classification and current trends. Omega, 45,
119–135.
ICORES 2019 - 8th International Conference on Operations Research and Enterprise Systems
252