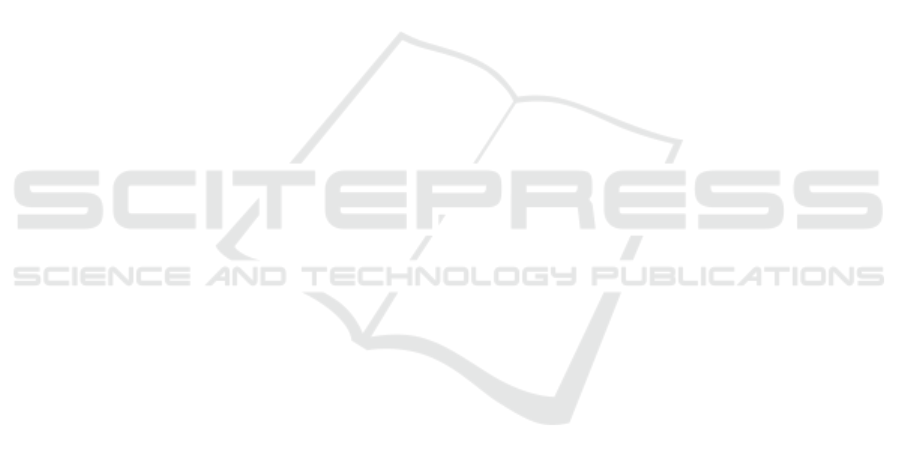
formal concept analysis to the analysis of a satisfac-
tion questionnaire for a course of bachelor. To do
this, we have performed a natural language process-
ing for open questions before extracting formal con-
cepts. The goal was to extract the lattice concepts by
taking into account uncertainty generated by the pro-
cessing of open questions. We have proposed to use
queries to extract interesting formal concepts. In or-
der to improve the presentation of the results of the
queries, we have proposed a visualization which high-
lights the uncertainty of the formal concept by using
a colour gradation and a circle with a radius propor-
tional to the number of answers. In future, we would
like to improve our experimentation in order to ob-
tain more experimental results and comparative eval-
uations. Particularly, we have to evaluate better the
query on the lattice concept and the use of the score
of relevance. In this study, we have limited our ap-
proach to a simple case of the certainty computation
of a formal concept but we wish to propose a general
frame for certainty computation. On the other hand,
we also need to improve the performance of the lattice
concept computation. We have explored only the use
of the guaranteed possibility operator, so we would
like to explore the interest of the use of the other op-
erators.
REFERENCES
Bedek, M. A., Kopeinik, S., Pr
¨
unster, B., and Albert, D.
(2015). Applying the formal concept analysis to in-
troduce guidance in an inquiry-based learning envi-
ronment. In IEEE 15th International Conference on
Advanced Learning Technologies, pages 285–289.
Belohlavek, R. (2004). Concept lattices and order in fuzzy
logic. In Annals of Pure and Applied Logic, volume
128, pages 277–298.
Belohlavek, R., Sklen
´
a
ˇ
r, V., Zacpal, J., and Sigmund, E.
(2007). Evaluation of questionnaires by means of for-
mal concept analysis. In In J. Diatta, P. Eklund, M.
Liquiere (Eds.): CLA 2007, Int. Conference on Con-
cept Lattices and Their Applications, pages 100–111.
Christen, P. (2006). A comparison of personal name match-
ing: Techniques and practical issues. In Proceedings
of the Sixth IEEE International Conference on Data
Mining - Workshops, ICDMW ’06, pages 290–294,
Washington, DC, USA. IEEE Computer Society.
Cross, V., Kandasamy, M., and Yi, W. (2010). Fuzzy con-
cept lattices: Examples using the gene ontology. In
2010 Annual Meeting of the North American Fuzzy
Information Processing Society, pages 1–6.
Dubois, D., de Saint-Cyr, F. D., and Prade, H. (2007). A
possibility-theoretic view of formal concept analysis.
volume 75, pages 195–213, Amsterdam, The Nether-
lands. IOS Press.
Dubois, D. and Prade, H. (1988). Possibility theory: An
Approach to Computerized Processing of Uncertainty.
Plenum Press, New York.
Dubois, D. and Prade, H. (2009). Possibility theory and
formal concept analysis in information systems. pages
1021–1026.
Dubois, D. and Prade, H. (2012). Possibility theory and
formal concept analysis: Characterizing independent
sub-contexts. volume 196, pages 4–16. On Advances
in Soft Computing Applied to Databases and Informa-
tion Systems.
Dubois, D. and Prade, H. (2015). Formal concept analysis
from the standpoint of possibility theory. In Baixeries,
J., Sacarea, C., and Ojeda-Aciego, M., editors, Formal
Concept Analysis, pages 21–38, Cham. Springer Inter-
national Publishing.
Fernandez-Manjon, B. and Fernandez-Valmayor, A. (1998).
Building educational tools based on formal concept
analysis. volume 3, pages 187–201.
Ganter, B. (1987). Algorithmen zur formalen begriffsanal-
yse. pages 241–255.
Jaro, M. A. (1989). Advances in record linking method-
ology as applied to the 1985 census of tampa florida.
volume 84, pages 414–420.
Kickmeier-Rust, M. D., Bedek, M., and Albert, D. (2016).
Theory-based learning analytics: Using formal con-
cept analysis for intelligent student modelling. pages
97–100, Las Vegas, Nevada, USA.
Kuznetsov, S. O. and Obiedkov, S. A. (2003). Comparing
performance of algorithms for generating concept lat-
tices. volume 14, pages 189–216.
Miclet, L., Prade, H., and Guennec, D. (2011). Looking
for analogical proportions in a formal concept analysis
setting. pages 295–307, Nancy, France.
Navarro, E., Prade, H., and Gaume, B. (2012). Clustering
sets of objects using concepts-objects bipartite graphs.
In H
¨
ullermeier, E., Link, S., Fober, T., and Seeger,
B., editors, Scalable Uncertainty Management, pages
420–432, Berlin, Heidelberg. Springer Berlin Heidel-
berg.
Sn
´
a
ˇ
sel, V., Hor
´
ak, Z., and Abraham, A. (2008). Under-
standing social networks using formal concept anal-
ysis. In 2008 IEEE/WIC/ACM International Confer-
ence on Web Intelligence and Intelligent Agent Tech-
nology, volume 3, pages 390–393.
Wille, R. (1982). Restructuring lattice theory: An approach
based on hierarchies of concepts. In Rival, I., edi-
tor, Ordered Sets, pages 445–470, Dordrecht. Springer
Netherlands.
Winkler, W. E. (1999). The state of record linkage and cur-
rent research problems. Technical report, Statistical
Research Division, U.S. Bureau of the Census.
Zadeh, L. A. (1978). Fuzzy sets as a basis for a theory of
possibility. volume 1, pages 3–28.
Zou, C. and Deng, H. (2017). Using fuzzy concept lattice
for intelligent disease diagnosis. In IEEE Access, vol-
ume 5, pages 236–242.
Uncertain Formal Concept Analysis for the Analyze of a Course Satisfaction Questionnaire
495