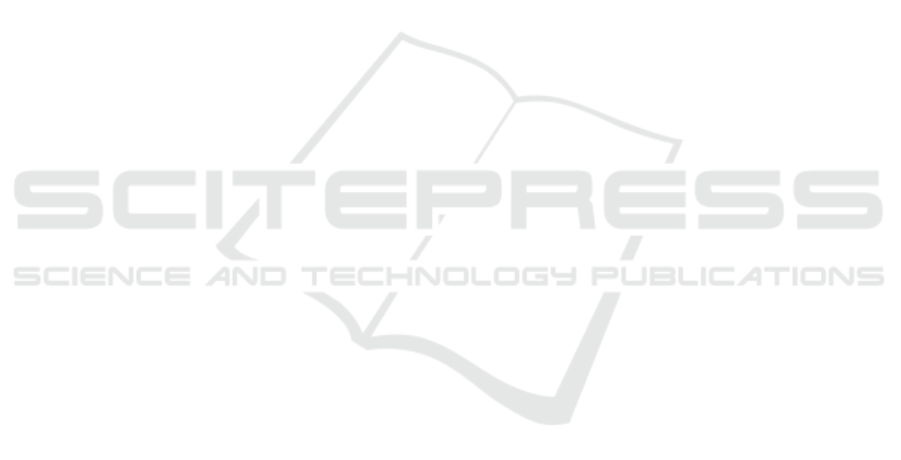
Glodek, M., Tschechne, S., Layher, G., Schels, M., Brosch,
T., Scherer, S., K
¨
achele, M., Schmidt, M., Neumann,
H., Palm, G., et al. (2011). Multiple classifier sys-
tems for the classification of audio-visual emotional
states. In Affective Computing and Intelligent Interac-
tion, pages 359–368. Springer.
Hori, C., Hori, T., Lee, T.-Y., Zhang, Z., Harsham, B.,
Hershey, J. R., Marks, T. K., and Sumi, K. (2017).
Attention-based multimodal fusion for video descrip-
tion. In Computer Vision (ICCV), 2017 IEEE Interna-
tional Conference on, pages 4203–4212. IEEE.
Huang, G., Liu, Z., Weinberger, K. Q., and van der Maaten,
L. (2017). Densely connected convolutional networks.
In Proceedings of the IEEE conference on computer
vision and pattern recognition, volume 1, page 3.
Kim, Y. (2014). Convolutional neural networks for sentence
classification. arXiv preprint arXiv:1408.5882.
Krizhevsky, A., Sutskever, I., and Hinton, G. E. (2012). Im-
agenet classification with deep convolutional neural
networks. In Advances in neural information process-
ing systems, pages 1097–1105.
Machajdik, J. and Hanbury, A. (2010). Affective image
classification using features inspired by psychology
and art theory. In Proceedings of the 18th ACM in-
ternational conference on Multimedia, pages 83–92.
ACM.
Mikolov, T., Sutskever, I., Chen, K., Corrado, G. S., and
Dean, J. (2013). Distributed representations of words
and phrases and their compositionality. In Advances in
neural information processing systems, pages 3111–
3119.
Ngiam, J., Khosla, A., Kim, M., Nam, J., Lee, H., and Ng,
A. Y. (2011). Multimodal deep learning. In Proceed-
ings of the 28th international conference on machine
learning (ICML-11), pages 689–696.
Ruder, S. (2017). An overview of multi-task learn-
ing in deep neural networks. arXiv preprint
arXiv:1706.05098.
Simonyan, K. and Zisserman, A. (2014). Very deep con-
volutional networks for large-scale image recognition.
arXiv preprint arXiv:1409.1556.
Sohn, K., Shang, W., and Lee, H. (2014). Improved multi-
modal deep learning with variation of information. In
Advances in Neural Information Processing Systems,
pages 2141–2149.
Soleymani, M., Garcia, D., Jou, B., Schuller, B., Chang, S.-
F., and Pantic, M. (2017). A survey of multimodal sen-
timent analysis. Image and Vision Computing, 65:3–
14.
Vielzeuf, V., Lechervy, A., Pateux, S., and Jurie, F. (2018).
Centralnet: a multilayer approach for multimodal fu-
sion. arXiv preprint arXiv:1808.07275.
Wang, J., Fu, J., Xu, Y., and Mei, T. (2016). Beyond object
recognition: Visual sentiment analysis with deep cou-
pled adjective and noun neural networks. In IJCAI,
pages 3484–3490.
Xu, N. and Mao, W. (2017). Multisentinet: A deep seman-
tic network for multimodal sentiment analysis. In Pro-
ceedings of the 2017 ACM on Conference on Informa-
tion and Knowledge Management, pages 2399–2402.
ACM.
You, Q., Jin, H., and Luo, J. (2017). Visual sentiment anal-
ysis by attending on local image regions. In AAAI,
pages 231–237.
You, Q., Luo, J., Jin, H., and Yang, J. (2015). Robust image
sentiment analysis using progressively trained and do-
main transferred deep networks. In AAAI, pages 381–
388.
You, Q., Luo, J., Jin, H., and Yang, J. (2016). Building a
large scale dataset for image emotion recognition: The
fine print and the benchmark. In AAAI, pages 308–
314.
Zadeh, A., Chen, M., Poria, S., Cambria, E., and Morency,
L.-P. (2017). Tensor fusion network for multimodal
sentiment analysis. arXiv preprint arXiv:1707.07250.
ICPRAM 2019 - 8th International Conference on Pattern Recognition Applications and Methods
376