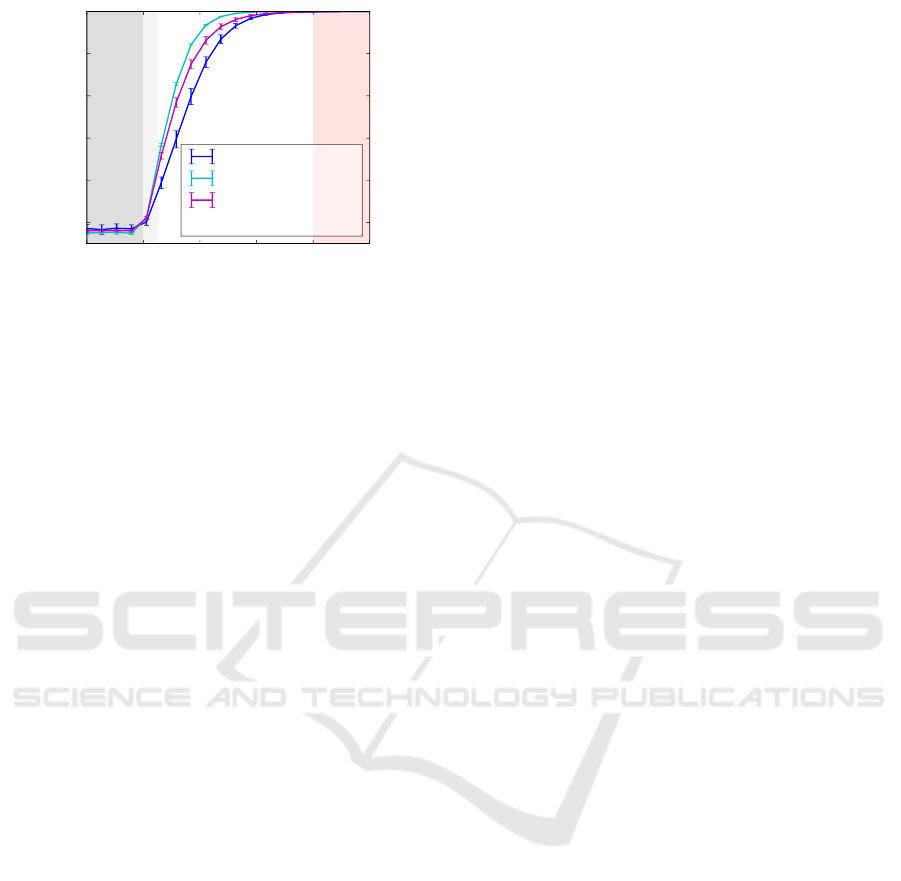
0.0 0.2 0.4 0.6 0.8 1.0
Side parameter t
0.5
0.6
0.7
0.8
0.9
1.0
AUC
Baseline
Proposed
JointOptimization
(Tanaka+, 2018)
Figure 9: AUCs on test images compared with (Tanaka
et al., 2018). The treatment as simple label noise failed to
correct labels in the preferable way for our settings.
labels along such series. Experiments on synthesized
datasets showed that our method achieved the same
performance as supervision by oracle labels, which
is the most sensitive to positive data, not limited by
given annotation ability.
Our method enables us to get models that can find
earlier anomalies than annotators by searching for dis-
criminative cues back to the earlier phase. In addition,
this can utilize poor labels made by simple processing
such as thresholding or by other classifiers.
REFERENCES
Arpit, D., Jastrzebski, S., Ballas, N., Krueger, D., Bengio,
E., Kanwal, M. S., Maharaj, T., Fischer, A., Courville,
A., Bengio, Y., and Lacoste-Julien, S. (2017). A closer
look at memorization in deep networks. In 34th Inter-
national Conference on Machine Learning (ICML).
Breiman, L. (2001). Random forests. In Machine Learning.
Goldberger, J. and Ben-Reuven, E. (2017). Training deep
neural-networks using a noise adaptation layer. In
ICLR, 5th International Conference on Learning Rep-
resentations.
Jindal, I., Nokleby, M., and Chen, X. (2016). Learning
deep networks from noisy labels with dropout regu-
larization. In 16th International Conference on Data
Mining (ICDM). IEEE.
Krizhevsky, A., Sutskever, I., and Hinton, G. E. (2012). Im-
agenet classification with deep convolutional neural
networks. In 26th Annual Conference on Neural In-
formation Processing Systems (NIPS).
Li, Y., Yang, J., Song, Y., Cao, L., Luo, J., and Li, L.-J.
(2017). Toward robustness against label noise in train-
ing deep discriminative neural networks. In Interna-
tional Conference on Computer Vision (ICCV).
Ma, X., Wang, Y., Houle, M. E., Zhou, S., Erfani,
S. M., Xia, S.-T., Wijewickrema, S., and Bailey, J.
(2018). Dimensionality-driven learning with noisy la-
bels. IEEE.
Patrini, G., Rozza, A., Menon, A. K., Nock, R., and Qu, L.
(2017). Making deep neural networks robust to label
noise: a loss correction approach. In Computer Vision
and Pattern Recognition (CVPR).
Reed, S. E., Lee, H., Anguelov, D., Szegedy, C., Erhan, D.,
and Rabinovich, A. (2015). Training deep neural net-
works on noisy labels with bootstrapping. In ICLR, In-
ternational Conference on Learning Representations.
Sukhbaatar, S., Bruna, J., Paluri, M., Bourdev, L., and Fer-
gus, R. (2015). Training convolutional networks with
noisy labels. In ICLR, International Conference on
Learning Representations.
Tanaka, D., Ikami, D., Yamasaki, T., and Aizawa, K.
(2018). Joint optimization framework for learning
with noisy labels. In Computer Vision and Pattern
Recognition (CVPR).
Vahdat, A. (2017). Toward robustness against label noise
in training deep discriminative neural networks. In
Neural Information Processing Systems (NIPS).
Veit, A., Alldrin, N., Chechik, G., Krasin, I., Gupta, A.,
and Belongie, S. (2017). Learning from noisy large-
scale datasets with minimal supervision. In Computer
Vision and Pattern Recognition (CVPR).
Wang, Y., Liu, W., Ma, X., Bailey, J., Zha, H., Song, L.,
and Xia, S.-T. (2018). Iterative learning with open-set
noisy labels. In Computer Vision and Pattern Recog-
nition (CVPR).
Xiao, T., Xia, T., Yang, Y., Huang, C., and Wang, X. (2015).
Learning from massive noisy labeled data for image
classification. In Computer Vision and Pattern Recog-
nition (CVPR).
Zhang, C., Bengio, S., Hardt, M., Recht, B., and Vinyals, O.
(2017). Understanding deep learning requires rethink-
ing generalization. In ICLR, International Conference
on Learning Representations.
Overcoming Labeling Ability for Latent Positives: Automatic Label Correction along Data Series
413