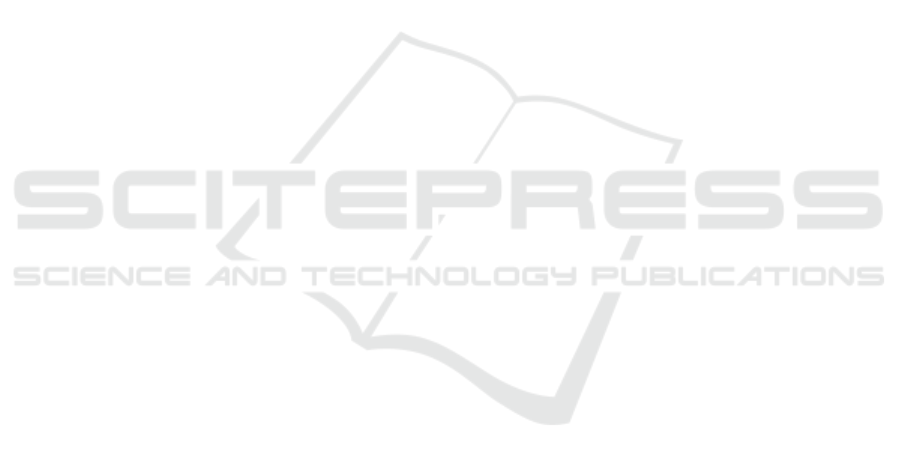
except in P@15 for the CRAN dataset for which their
approach outperformed the APMM (see bold values
in Table 7).
6 CONCLUSION
The success of analogical proportions in a variety of
domains, such as in classification and language pro-
cessing, led us to wonder whether it may be a suc-
cessful tool for building an IR matching model. We
are mainly interested to this last field in this paper. We
have first studied the way to apply analogy between
queries and documents. Then, given a particular in-
dexing query term, we formalize two logical propor-
tions linking queries and their corresponding relevant
documents for an analogical inference. These propor-
tions form the basis for our matching model.
The two proposed analogical proportions help to
define agreement and disagreement scores useful to
estimate to what extent any document, from the col-
lection, is to be accepted or rejected given a new
query. The agreement score is calculated according
to the common terms between the query and the doc-
ument while the disagreement is computed using the
number of terms they differ. The two scores treat doc-
uments differently: the disagreement allows you to
exclude irrelevant documents from the returned list,
while the agreement score strengthen the relevance of
the remaining documents not eliminated by the dis-
agreement. Based on these two scores, we have pro-
posed and tested a new analogy-based IR matching
model on three IR Glasgow test collections. The ex-
perimental results highlighted the effectiveness of the
model compared to the well known efficient Okapi IR
model.
The analogy-based IR matching model can be ap-
plied in different IR/CLIR tasks such as in query
expansion, disambiguation and translation tasks that
will be our future interest.
REFERENCES
Amit, S. (2001). Modern information retrieval: A brief
overview. Bulletin of the IEEE Computer Society
Technical Committee on Data Engineering, 24:35–43.
Bayoudh, S., Miclet, L., and Delhay, A. (2007). Learning
by analogy: A classification rule for binary and nomi-
nal data. In Proc. IJCAI 2007, pages 678–683.
Bounhas, M., Prade, H., and Richard, G. (2014). Analogical
classification: A new way to deal with examples. In
Proc. ECAI, pages 135–140.
Bounhas, M., Prade, H., and Richard, G. (2017a). Analogy-
based classifiers for nominal or numerical data. IJAR,
91:36–55.
Bounhas, M., Prade, H., and Richard, G. (2017b).
Oddness/evenness-based classifiers for boolean or nu-
merical data. IJAR, 82:81–100.
Bounhas, M., Prade, H., and Richard, G. (2018). Oddness-
based classification: A new way of exploiting neigh-
bors. IJIS, 33(12):2379–2401.
Demsar, J. (2006). Statistical comparisons of classifiers
over multiple data sets. Journal of Machine Learning
Research, 7:1–30.
Fuhr, N. and Buckley, C. (1991). A probabilistic learning
approach for document indexing. ACM Trans. on Inf.
Sys., 9(3):223–248.
Hesse, M. (1959). On defining analogy. Proceedings of the
Aristotelian Society, 60:79–100.
Lepage, Y. (2001). Analogy and formal languages. In Proc.
FG/MOL 2001, pages 373–378.
Miclet, L., Bayoudh, S., and Delhay, A. (2008). Analog-
ical dissimilarity: Definition, algorithms and two ex-
periments in machine learning. Journal of Artificial
Intelligence Research, 32:793–824.
Miclet, L. and Prade, H. (2009). Handling analogical pro-
portions in classical logic and fuzzy logics settings. In
Proc. ECSQARU’09, pages 638–650. Springer, LNCS
5590.
Prade, H. and Richard, G. (2010). Reasoning with logical
proportions. In Proc. KR 2010, pages 545–555.
Prade, H. and Richard, G. (2012). Homogeneous logi-
cal proportions: Their uniqueness and their role in
similarity-based prediction. In Proc. KR 2012, pages
402–412.
Prade, H. and Richard, G. (2013). From analogical pro-
portion to logical proportions. Logica Universalis,
7(4):441–505.
Prade, H., Richard, G., and Yao, B. (2012). Enforcing regu-
larity by means of analogy-related proportions-a new
approach to classification. Int. J. of Comp. Inf. Sys.
and Indus. Management App., 4:648–658.
Stroppa, N. and Yvon, F. (2005a). An analogical learner for
morphological analysis. In Proc. CoNLL 2005, pages
120–127.
Stroppa, N. and Yvon, F. (2005b). Analogical learning and
formal proportions: Definitions and methodological
issues. Technical report.
Yvon, F., Stroppa, N., Delhay, A., and Miclet, L.
(2004). Solving analogical equations on words.
Technical report, Ecole Nationale Sup
´
erieure des
T
´
el
´
ecommunications.
ICAART 2019 - 11th International Conference on Agents and Artificial Intelligence
504