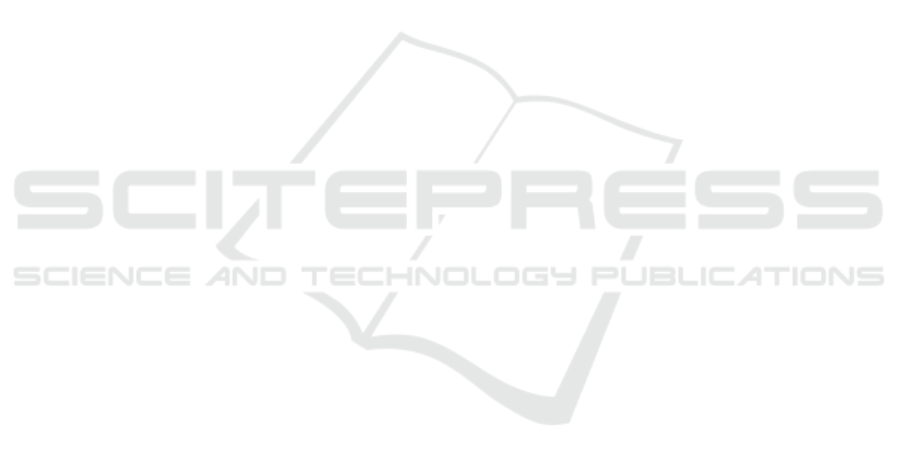
4 DISCUSSION AND
CONCLUSIONS
The experiment was run to test for math improvement
in eighth and ninth grade students using the educa-
tional video game. In this sense, a BP model was
used to predict the percentage of learning growth of
students with specific characteristics.
The resulting characteristics (or attributes) gath-
ered were those that may show a significant relation-
ship with the performance of the students in math-
ematics which may determine the efficiency of the
game in such students. As such, the BP algorithm
can be used to predict how much the students are able
to improve their skills in mathematics by playing the
video game.
The characteristics of each student were used to
train an artificial neural network model using a nor-
malization of the data and a cross-validation proce-
dure in order to obtain the MSE of the prediction with
different architectures.
The MSE obtained in Table 1 and Table 2 is lower
than 0.0069 in most of the cases, therefore, we ob-
tained an efficient predictor. As shown in Table 1, the
one layer 10 neurons and two layers 5 and 20 neurons
architecture models have better results (smaller MSE)
in the prediction of the percentage of improvement in
comparison with the other ones used, when using the
twelve attributes.
From the results obtained in Table 2, the model
that gets better predictions is the one that uses an ar-
chitecture of two layers with 5 and 20 neurons, with
the geographical attribute position removed. We were
able to remove the geographical attribute because it
increased the MSE with this architecture.
Fig. 5 shows how as the training dataset (students
number) increases the MSE diminishes and then starts
to have a decrease in the 1%, reaching the smallest
MSE when the training dataset was 98% equivalent to
approximately 353 students, to then obtain an overfit-
ting problem when the training dataset was 99%.
For future work, the data collected can be used to
do a classification of the percentage learning rate us-
ing the BP algorithm taking a multi-class output de-
pending on an interval learning rate. The attributes
that were evaluated can be studied in more detail with
the use of a Self-Organizing Map (SOM), to estab-
lish what attribute or combination of attributes can
enhance the prediction in student learning.
As an overall conclusion, the results presented in
this work show that when the BP algorithm with all
the attributes was used, the best neurocomputational
model was one layer with 10 neurons, in comparison
when the geographical position was deleted, the best
architecture was two layers with 5 and 20 neurons,
to predict the student percentage of improvement in
mathematics after the use of a video game, and there-
fore, claiming if the video game is recommendable or
not for certain students.
ACKNOWLEDGEMENTS
The authors acknowledge support from Unidad
Educativa “Eloy Alfaro”, from Unidad Educativa
“Teodoro G
´
omez de la Torre”, and from Universidad
Yachay Tech, School of Mathematical Science and In-
formation Technology.
REFERENCES
Andersson, A., Valero, P., and Meaney, T. (2015). I am
[not always] a maths hater: Shifting students’ identity
narratives in context. Educational Studies in Mathe-
matics, pages 143–161.
Boot, W. R., Kramer, A. F., Simons, D. J., Fabiani, M., and
Gratton, G. (2008). The effects of video game play-
ing on attention, memory, and executive control. Acta
Psychologica, pages 387 – 398.
Borba, M., Askar, P., Engelbrecht, J., Gadanidis, G.,
Llinares, S., and Sanchez Aguilar, M. (2016). Blended
learning, e-learning and mobile learning in mathemat-
ics education. ZDM Mathematics Education, pages
589–610.
Castellar, E. N., All, A., de Marez, L., and Looy, J. V.
(2015). Cognitive abilities, digital games and arith-
metic performance enhancement: A study comparing
the effects of a math game and paper exercises. Com-
puters and Education, 85:123 – 133.
Comi, L., Argentin, G., Gui, M., Origoc, F., and Pagani, L.
(2017a). Is it the way they use it? teachers, ict and stu-
dent achievement. Economics of Education Review,
pages 24–39.
Comi, S. L., Argentin, G., Gui, M., Origo, F., and Pagani, L.
(2017b). Is it the way they use it? teachers, ict and stu-
dent achievement. Economics of Education Review,
pages 24 – 39.
Hurst, M. and Cordes, S. (2017). Understanding Emotions
in Mathematical Thinking and Learning. Academic
Press, 1st edition.
Musso, M., Kynd, E., Cascallar, E., and Dochy, F. (2013).
Predicting general academic performance and iden-
tifying the fifferential contribution of participating
variables using artificial neural networks. Frontline
Learning Research, pages 42–71.
Pachemska, S., Atanasova-Pachemska, T., Iliev, D., and
Seweryn-Kuzmanovska, M. (2014). Analyses of stu-
dent’s achievement depending on math teaching meth-
ods. Procedia - Social and Behavioral Sciences, pages
4035 – 4039.
ICAART 2019 - 11th International Conference on Agents and Artificial Intelligence
558