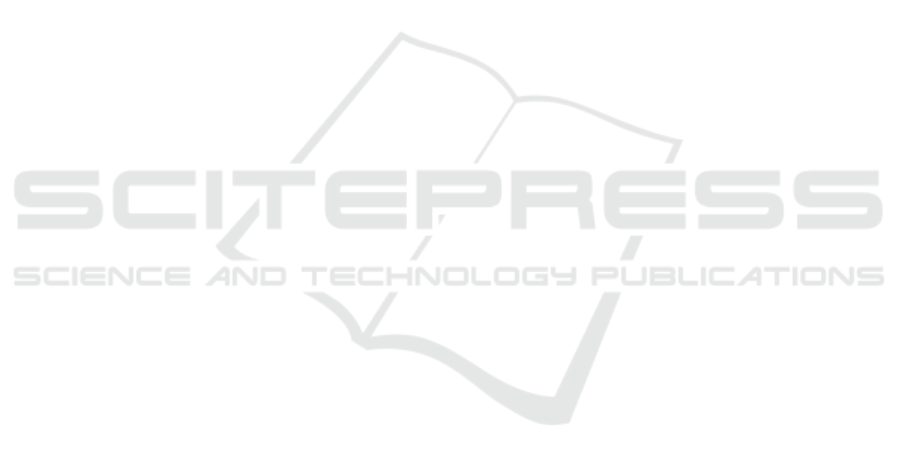
the first principal component captures the functional
grand mean. This helps to explain why by taking the
functional mean and the functional standard deviation
of power spectrum from set D as functional probes
also produce separable functional probe values.
We further investigate the effect of the number
of basis functions (i.e., K) on the separability of
extracted signal features (i.e., principal component
scores of power spectra). The obtained results are
displayed in Figure 8. We observe that the proposed
method is highly successful in separating the artifacts
(i.e., open/closed eyes) as the results did not depend
on how the number of basis functions was selected.
The feature separability increases with the decrease of
K, i.e., the number of basis functions. This may sug-
gest that the sparsity in approximation of the signal
power spectra plays an important role in the success
of applying functional principal component analysis.
When K=200, the extracted features for epileptic sig-
nals overlap significantly. This overlapping changes
when K decreases, and features start to be fully sepa-
rable when K is relatively small, for example, around
25. However, the overall separability between healthy
and epileptic signals is not affected by the number of
basis functions.
4 CONCLUDING REMARKS
Clustering and classification of highly dimensional
data are important tasks in pattern recognition and ar-
tificial intelligence. To be successful in using machine
learning techniques, including clustering and classifi-
cation, dimension reduction of data is a key approach.
In this work, we have conisdered an approach that
first transforms signals to the spectral domain and ob-
tains their power spectra. Next, we have applied the
functional data analysis techniques to further investi-
gate the charateristics of the signals. We have demon-
strated that functional data analysis in spectral domain
is useful for understanding the key features of differ-
ent types of EEG signals. Especially, the extracted
features, using functional principal component anal-
ysis, can be used for classification of different types
of EEG signals. Also we have investigated the effect
of sparsity on the performance of separating signal
features. The obtained results demonstrate that the
proposed method may be useful for an epilepsy diag-
nosis. Future work will focus on the study of wavelet
spectral domain functional PCA and its application to
clustering random signals.
ACKNOWLEDGEMENT
The authors acknowledges partial support from
NSERC (Natural Sciences and Engineering Research
Council of Canada).
REFERENCES
Alickovic, E., Kevric, J., and Subasi, A. (2018). Perfor-
mance evaluation of empirical mode decomposition,
discrete wavelet transform, and wavelet packed de-
composition for automated epileptic seizure detection
and prediction. Biomedical Signal Processing and
Control, 39:94–102.
Bouveyron, C., Girard, S., and Schmid, C. (2007). High-
dimensional data clustering. Computational Statistics
& Data Analysis, 52(1):502–519.
De Boor, C., De Boor, C., Math
´
ematicien, E.-U., De Boor,
C., and De Boor, C. (1978). A practical guide to
splines, volume 27. Springer-Verlag New York.
Fergus, P., Hussain, A., Hignett, D., Al-Jumeily, D., Abdel-
Aziz, K., and Hamdan, H. (2016). A machine learning
system for automated whole-brain seizure detection.
Applied Computing and Informatics, 12(1):70–89.
Gandhi, T., Panigrahi, B. K., and Anand, S. (2011). A com-
parative study of wavelet families for eeg signal clas-
sification. Neurocomputing, 74(17):3051–3057.
Garcia, G. N., Ebrahimi, T., and Vesin, J.-M. (2003).
Support vector eeg classification in the fourier and
time-frequency correlation domains. In Neural En-
gineering, 2003. Conference Proceedings. First Inter-
national IEEE EMBS Conference on, pages 591–594.
IEEE.
Jimenez, L. O. and Landgrebe, D. A. (1998). Supervised
classification in high-dimensional space: geometrical,
statistical, and asymptotical properties of multivariate
data. IEEE Transactions on Systems, Man, and Cyber-
netics, Part C (Applications and Reviews), 28(1):39–
54.
Kriegel, H.-P., Kr
¨
oger, P., and Zimek, A. (2009). Clustering
high-dimensional data: A survey on subspace cluster-
ing, pattern-based clustering, and correlation cluster-
ing. ACM Transactions on Knowledge Discovery from
Data (TKDD), 3(1):1.
Li, D., Pedrycz, W., and Pizzi, N. J. (2005). Fuzzy wavelet
packet based feature extraction method and its appli-
cation to biomedical signal classification. IEEE Trans-
actions on biomedical engineering, 52(6):1132–1139.
Liang, S.-F., Wang, H.-C., and Chang, W.-L. (2010). Com-
bination of eeg complexity and spectral analysis for
epilepsy diagnosis and seizure detection. EURASIP
Journal on Advances in Signal Processing, 2010:62.
Lima, C. A., Coelho, A. L., and Chagas, S. (2009). Auto-
matic eeg signal classification for epilepsy diagnosis
with relevance vector machines. Expert Systems with
Applications, 36(6):10054–10059.
ICPRAM 2019 - 8th International Conference on Pattern Recognition Applications and Methods
126