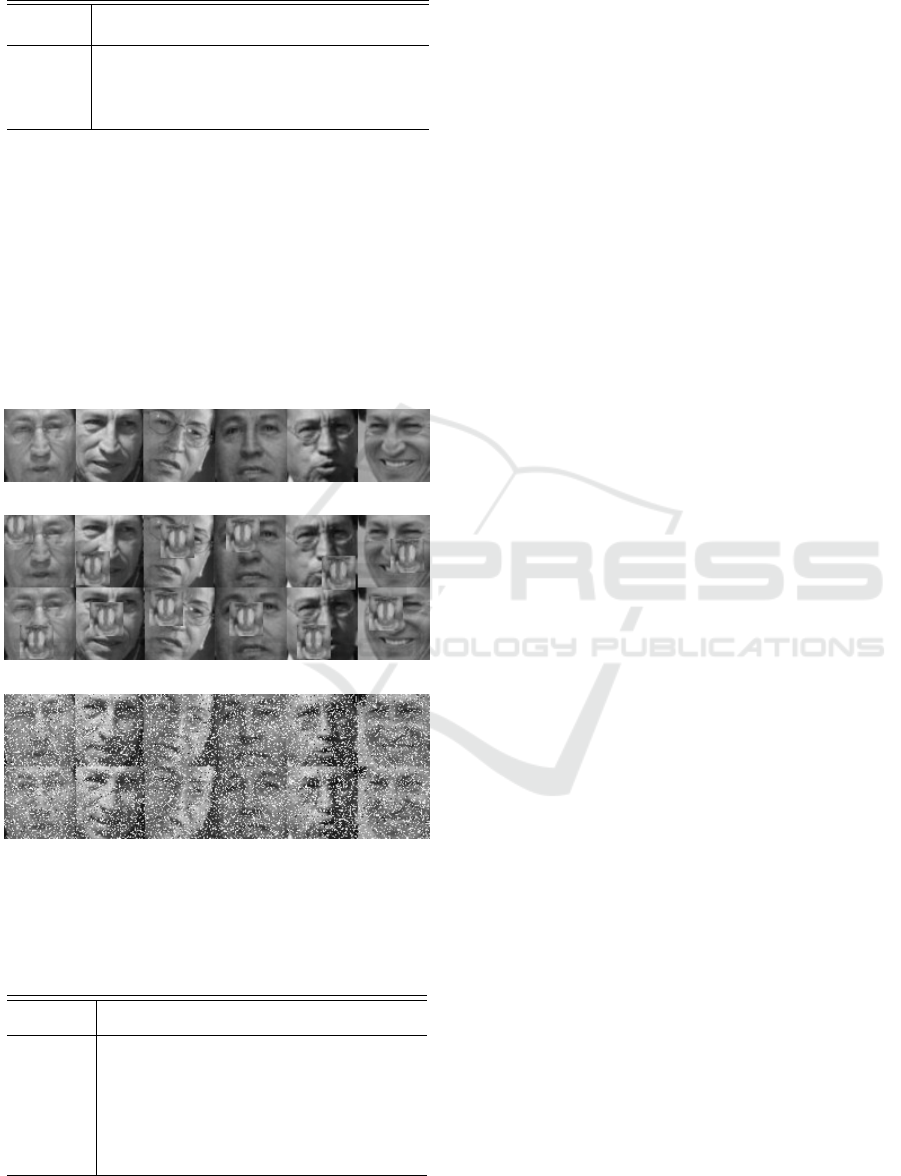
Table 5: Recognition Accuracy (%) on Extended YaleB face
database with 20% corruption.
Training
samples
PCA LFDA LPP SPP CRP
Proposed
method
l=8 53.6 73.8 71.8 72.8 72.3 73.1
l=16 64.3 84.1 83.9 82.3 91.1 91.6
3.4 LFW Database
For this database, 100 subjects of LFWa database (Liu
et al., 2015) were used. For each person, 6 images
are selected. The images are cropped to eliminate the
background, and resized to 64 × 64. Samples of one
subject are shown in figure 4.
As in experiments with the privious databases, our
method gets the highest recognition rates among all
methods with LFW database as shown in table 6.
(a)
(b)
(c)
Figure 4: Sample images of LFW databasse of (a) Samples
for one peron. (b) Samples with 20% occlusion . (c) Sam-
ples with 20% corruption.
Table 6: Recognition Accuracy (%) on LFW database.
Database PCA LFDA LPP SPP CRP
Proposed
method
LFW 66.9 94.1 92.5 93.8 95.1 95.3
Occluded
LFW
60.9 86.8 81.3 86.1 83.2 89.1
Corrupted
LFW
61.5 88.7 85.8 87.4 84.1 89.4
4 CONCLUSION
A new regression based face recognition algorithm
is proposed. The method uses `
0
-norm to transform
the image into a sparse vector. It uses `
2
-norm regu-
larization to prevent the overlapping of nonzero cof-
fecients that belongs to different subjects. The pro-
posed method is tested with different face databases.
It is compared with other well known face recognition
methods. The experimental results show the superior-
ity of accuracy of the proposed method. They also
show the robustness of the method under occlusion
and corruption. Another advantage of the proposed
method is the low computational cost, since it only
contains matrix vector multiplication and norm com-
putation to achieve the classification task.
REFERENCES
Belhumeur, P. N., Hespanha, J. P., and Kriegman, D. J.
(1997). Eigenfaces vs. fisherfaces: recognition us-
ing class specific linear projection. IEEE Transac-
tions on Pattern Analysis and Machine Intelligence,
19(7):711–720.
Georghiades, A. S., Belhumeur, P. N., and Kriegman, D. J.
(2001). From few to many: illumination cone models
for face recognition under variable lighting and pose.
IEEE Transactions on Pattern Analysis and Machine
Intelligence, 23(6):643–660.
Gu, Q., Li, Z., and Han, J. (2011). Joint feature selection
and subspace learning. In Proceedings of the Twenty-
Second International Joint Conference on Artificial
Intelligence - Volume Volume Two, IJCAI’11, pages
1294–1299. AAAI Press.
He, X., Yan, S., Hu, Y., Niyogi, P., and Zhang, H.-J. (2005).
Face recognition using laplacianfaces. IEEE Trans-
actions on Pattern Analysis and Machine Intelligence,
27(3):328–340.
Huang, G. B., Ramesh, M., Berg, T., and Learned-Miller,
E. (2007). Labeled faces in the wild: A database for
studying face recognition in unconstrained environ-
ments. Technical Report 07-49, University of Mas-
sachusetts, Amherst.
Lai, Z., Xu, Y., Yang, J., Shen, L., and Zhang, D.
(2017). Rotational invariant dimensionality reduc-
tion algorithms. IEEE Transactions on Cybernetics,
47(11):3733–3746.
Liu, Z., Luo, P., Wang, X., and Tang, X. (2015). Deep learn-
ing face attributes in the wild. In Proceedings of the
2015 IEEE International Conference on Computer Vi-
sion (ICCV), ICCV ’15, pages 3730–3738, Washing-
ton, DC, USA. IEEE Computer Society.
Ma, Z., Nie, F., Yang, Y., Uijlings, J. R. R., Sebe, N., and
Hauptmann, A. G. (2012). Discriminating joint fea-
ture analysis for multimedia data understanding. IEEE
Transactions on Multimedia, 14(6):1662–1672.
Sparse l2-norm Regularized Regression for Face Recognition
457