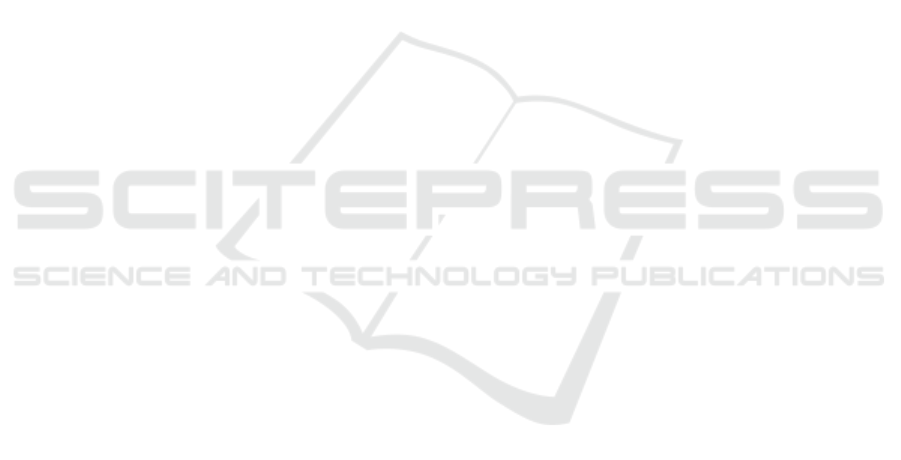
improved skill, it may be possible to achieve a skill
that is one step higher than the current skill. However,
this method is not perfect because we cannot confirm
it. For this reason, we would like to conduct other
experiments in order to verify our method using the
results obtained in this paper.
5.3 Remaining Issues
It is difficult to find a peak of each cluster, which is
aimed at improving running motion, because the peak
is not clear using our dataset alone. In other words,
we do not know which direction is the peak for the
subjects to improve the skill of their running motion.
We believe that this problem can be solved to
improve the running motion by not only subjects who
have no experience of athletics but also subjects who
have experience of athletics. The reason is that, using
our method, the running motion of experienced
people may be a peak that is a few steps higher than
that of non-experienced people, and their motion may
be the peak for the motion of non-experienced people
in the same cluster .
Second, in this paper, we evaluate the running
motion using the evaluation items in Table 1, but it
will be necessary to automatically output a score for
running motion in the future. For this reason, it is
possible to find the score for a performance one step
higher than the current one in the same cluster. In
particular, the evaluation items in Table 1 focus only
on the upper limbs and lower limbs, yet other items
are needed, such as a forward-bent posture, which is
important in running motion. We plan to expand these
items by using a method such as dynamically
analysing each cluster’s features obtained as describ-
ed in Section 3.3.
6 CONCLUSIONS
This paper has proposed a system that can let the
viewer understand the skill of a performer and can
output feedback for achieving one step higher
performance aimed at by the performer. Among them,
we proposed CDIV as a method for analysing the
input component of the features obtained by an
autoencoder in which the middle layer is replaced
with an LSTM layer. From the CDIV, the validity of
the running skill, in which five clusters were obtained
by hierarchical clustering, was confirmed by
comparing with the evaluation items in Table 1. In
addition, we showed the possibility of detecting skill
involving aspects such as the individual’s charact-
eristics. Then, we demon-strated the possibility of a
method of feedback for improving the performance to
a level one step higher than the current one using the
CDIV of each cluster in Cluster 1.
As the future work, we will further clarify the skill
of running motion by adding the running motions of
experienced athletes. Also, we will improve the
evaluation items by dynamically analysing the runn-
ing motion in each cluster. Moreover, we would like
to conduct other experi-ments in order to verify our
method.
ACKNOWLEDGEMENTS
This study was part of research activities of the
Human Performance Laboratory, Organization for
University Research Initiatives, Waseda University.
REFERENCES
Andriluka, M., Pishchulin, L., Gehler, V. P. and Schiele, B.,
2014. 2D human pose estimation: New benchmark and
State of the Art Analysis. In the IEEE Conference on
Computer Vision and Pattern Recognition(CVPR),
3686-3693.
Chu, X., Yang, W., Ouyang, W., Ma, C., Yuillw, L. A. and
Wang, X., 2017. Multi-Context Attention for Human
Pose Estimation. In the IEEE Conference on Computer
Vision and Pattern Recognition(CVPR), 1831-1840.
Fragkiadaki, K., Levine, S., Felsen, P. and Malik, J., 2015.
Recurrent Network Models for Human Dynamics.
International Conference on Computer Vision(ICCV),
4346-4354.
Jia, Y., Shelhamer, E., Donohue, J., Karayev, S., Long, J.,
Girshick, R., Guadarrama, S. and Darrell, T., 2014.
Caffe: Convolutional architecture for fast feature
embedding. arXiv:1408.5093.
Newell, A., Yang, K. and Deng, J., 2016. Stacked
Hourglass Networks for Human Pose Estimation.
arXiv:1603.06937.
Parmar, P. and Morris, T. B., 2016. Measuring the quality
of exercises. In IEEE International Conference
Engineering in Medicine and Biology Society, 556-
571.
Pirsiavash, H., Vondrick, C. and Torralba, A., 2014.
Assessing the Quality of Actions. European Conference
on Computer Vision(ECCV), 556-571.
Redmon, J. and Farhadi, A., 2018. YOLOv3: An
Incremental Improvement. arXiv:1804.02767.
Selvaraju, R. R., Cogswell, M., Dasm, A., Vedantam, R.,
Parikh, D. and Batra, D., 2017. Grad-Cam: Visual
Explanations from Deep Network via Gradient-based
Localization. International Conference on Computer
Vision(ICCV), 618-626.
Suzuki, Y., Tomozoe, H., Yoshinaga, T. Kaji, M. and
Hirayama, K., 2016. Shissou dousa no
ICPRAM 2019 - 8th International Conference on Pattern Recognition Applications and Methods
474