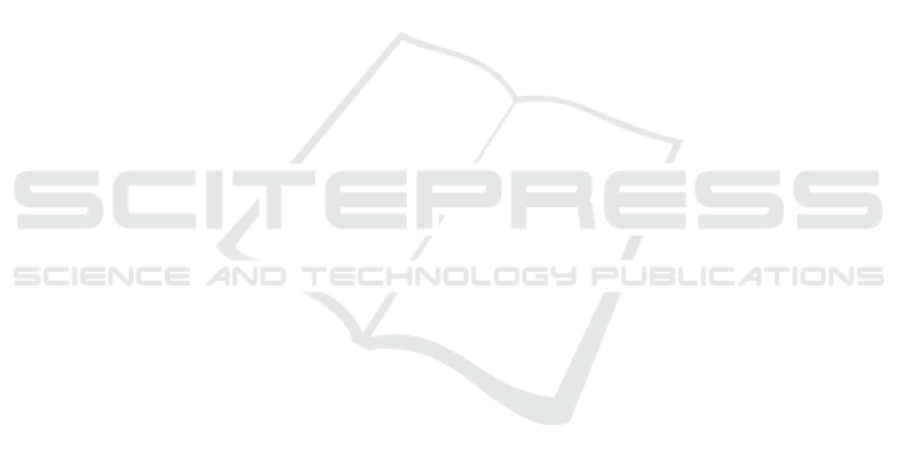
PFSBA. The BAUM model, employs user-specific
and goal encoding of attributes in order to capture the
coherence of interaction within its structure. In ad-
dition, two K-Nearest State Smoothing policies are
introduced and evaluated, achieving higher perfor-
mance than their single-state counterpart. The pro-
posed UM achieves results similar to current deep
learning approaches, using a lightweight model with
better performance in terms of computation time and
memory consumption.
Future work will involve developing a methodol-
ogy for the automatic inference of dialogue attributes
and testing the presented approach on other goal-
oriented dialogue corpora. In addition, the robustness
of the proposed simulated user modeling approach
will be tested regarding its applicability to train and
evaluate statistical spoken dialogue systems.
ACKNOWLEDGEMENTS
This work has been partially funded by the Span-
ish Ministry of Science under grants TIN2014-54288-
C4-4-R and TIN2017-85854-C4-3-R and by the Eu-
ropean Commission H2020 SC1-PM15 EMPATHIC
project, RIA grant 69872.
REFERENCES
Casanueva, I., Budzianowski, P., Su, P.-H., Mrk
ˇ
sic, N.,
Wen, T.-H., Ultes, S., Rojas-Barahona, L., Young,
S., and Ga
ˇ
sic, M. (2017). A benchmarking environ-
ment for reinforcement learning based task oriented
dialogue management. stat, 1050:29.
Chandramohan, S., Geist, M., Lefevre, F., and Pietquin, O.
(2011). User simulation in dialogue systems using
inverse reinforcement learning. In Interspeech 2011,
pages 1025–1028.
Chen, L., Zhou, X., Chang, C., Yang, R., and Yu, K. (2017).
Agent-aware dropout dqn for safe and efficient on-
line dialogue policy learning. In Proceedings of the
2017 Conference on Empirical Methods in Natural
Language Processing, pages 2444–2454.
Crook, P. and Marin, A. (2017). Sequence to sequence mod-
eling for user simulation in dialog systems. In Pro-
ceedings of the 18th Annual Conference of the Inter-
national Speech Communication Association (INTER-
SPEECH 2017), pages 1706–1710.
Cuay
´
ahuitl, H., Renals, S., Lemon, O., and Shimodaira, H.
(2005). Human-computer dialogue simulation using
hidden markov models. In Automatic Speech Recog-
nition and Understanding, 2005 IEEE Workshop on,
pages 290–295. IEEE.
Eckert, W., Levin, E., and Pieraccini, R. (1997). User mod-
eling for spoken dialogue system evaluation. In Auto-
matic Speech Recognition and Understanding, 1997.
Proceedings., 1997 IEEE Workshop on, pages 80–87.
IEEE.
Eshghi, A., Shalyminov, I., and Lemon, O. (2017). Boot-
strapping incremental dialogue systems from minimal
data: the generalisation power of dialogue grammars.
In Proceedings of the 2017 Conference on Empiri-
cal Methods in Natural Language Processing, pages
2220–2230.
Ga
ˇ
si
´
c, M., Jur
ˇ
c
´
ı
ˇ
cek, F., Keizer, S., Mairesse, F., Thomson,
B., Yu, K., and Young, S. (2010). Gaussian processes
for fast policy optimisation of pomdp-based dialogue
managers. In Proceedings of the 11th Annual Meeting
of the Special Interest Group on Discourse and Dia-
logue, pages 201–204. Association for Computational
Linguistics.
Ga
ˇ
si
´
c, M., Mrk
ˇ
si
´
c, N., Rojas-Barahona, L. M., Su, P.-H.,
Ultes, S., Vandyke, D., Wen, T.-H., and Young, S.
(2017). Dialogue manager domain adaptation using
gaussian process reinforcement learning. Computer
Speech & Language, 45:552–569.
Ghigi, F. and Torres, M. I. (2015). Decision making strate-
gies for finite-state bi-automaton in dialog manage-
ment. In Natural Language Dialog Systems and In-
telligent Assistants, pages 209–221. Springer.
Henderson, M., Thomson, B., and Williams, J. (2013). Dia-
log state tracking challenge 2 & 3 handbook. camdial.
org/mh521/dstc.
Layla, E. A., Jing, H., and Suleman, K. (2016). A sequence-
to-sequence model for user simulation in spoken dia-
logue systems. In Interspeech.
Levin, E., Pieraccini, R., and Eckert, W. (2000). A stochas-
tic model of human-machine interaction for learning
dialog strategies. IEEE Transactions on speech and
audio processing, 8(1):11–23.
Orozko, O. R. and Torres, M. I. (2015). Online learning of
stochastic bi-automaton to model dialogues. In Pat-
tern Recognition and Image Analysis - 7th Iberian
Conference, IbPRIA 2015, Santiago de Compostela,
Spain, June 17-19, 2015, Proceedings, pages 441–
451.
Pietquin, O. (2005). A framework for unsupervised learning
of dialogue strategies. Presses univ. de Louvain.
Pietquin, O. and Dutoit, T. (2006). A probabilistic frame-
work for dialog simulation and optimal strategy learn-
ing. IEEE Transactions on Audio, Speech, and Lan-
guage Processing, 14(2):589–599.
Quarteroni, S., Gonz
´
alez, M., Riccardi, G., and Varges, S.
(2010). Combining user intention and error modeling
for statistical dialog simulators. In INTERSPEECH,
pages 3022–3025.
Schatzmann, J., Thomson, B., Weilhammer, K., Ye, H., and
Young, S. (2007). Agenda-based user simulation for
bootstrapping a pomdp dialogue system. In Human
Language Technologies 2007: The Conference of the
North American Chapter of the Association for Com-
putational Linguistics; Companion Volume, Short Pa-
pers, pages 149–152. Association for Computational
Linguistics.
Schatzmann, J., Weilhammer, K., Stuttle, M., and Young,
S. (2006). A survey of statistical user simulation tech-
niques for reinforcement-learning of dialogue man-
Goal-conditioned User Modeling for Dialogue Systems using Stochastic Bi-Automata
133