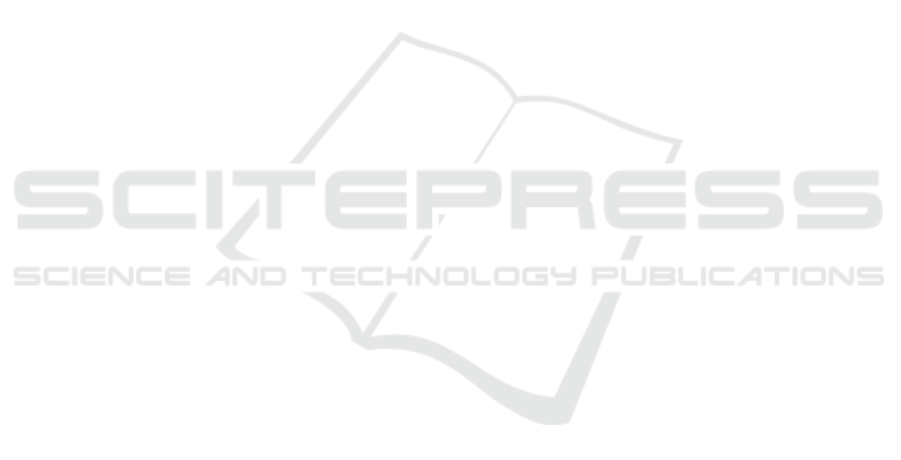
Federal Ministry for Economic Affairs and Energy,
editor (2016). Ergebnispapier - Aspekte der
Forschungsroadmap in den Anwendungsszenarien.
Berlin.
Ferdous, R., Khan, F., Sadiq, R., Amyotte, P., and Veitch,
B. (2009). Handling data uncertainties in event tree
analysis. Process safety and environmental protection,
87(5):283–292.
Frey, C. W. (2012). Monitoring of complex industrial
processes based on self-organizing maps and water-
shed transformations. In Industrial Technology (ICIT),
2012 IEEE International Conference on, pages 1041–
1046. IEEE.
Fullen, M., Sch
¨
uller, P., and Niggemann, O. (2017). Defin-
ing and validating similarity measures for industrial
alarm flood analysis. In Industrial Informatics (IN-
DIN), 2017 IEEE 15th International Conference on,
pages 781–786. IEEE.
Granger, C. W. (1969). Investigating causal relations
by econometric models and cross-spectral methods.
Econometrica: Journal of the Econometric Society,
pages 424–438.
Horch, A. (2000). Condition monitoring of control loops.
PhD thesis, Signaler, sensorer och system.
Jaber, A. A. and Bicker, R. (2016). Industrial robot back-
lash fault diagnosis based on discrete wavelet trans-
form and artificial neural network. American Journal
of Mechanical Engineering, 4(1):21–31.
Kolodner, J. (1993). Case-based Reasoning. Artificial in-
telligence. Morgan Kaufmann Publishers.
Maier, A. (2014). Online passive learning of timed au-
tomata for cyber-physical production systems. In The
12th IEEE International Conference on Industrial In-
formatics (INDIN 2014). Porto Alegre, Brazil.
Maier, A., Vodencarevic, A., Niggemann, O., Just, R., and
Jaeger, M. (2011). Anomaly detection in produc-
tion plants using timed automata. In 8th Interna-
tional Conference on Informatics in Control, Automa-
tion and Robotics (ICINCO), pages 363–369.
Murphy, K. P. (2012). Machine Learning. A Probabilistic
Perspective. Massachusetts Institute of Technology.
Niggemann, O. and Lohweg, V. (2015). On the diagno-
sis of cyber-physical production systems: state-of-
the-art and research agenda. In Proceedings of the
Twenty-Ninth AAAI Conference on Artificial Intelli-
gence, pages 4119–4126. AAAI Press.
Niggemann, O., Stein, B., Voden
ˇ
carevi
´
c, A., Maier, A., and
Kleine Buning, H. (2012). Learning behavior models
for hybrid timed systems. In Twenty-Sixth Conference
on Artificial Intelligence (AAAI-12).
Niggemann, O., Vodencarevic, A., Maier, A., Windmann,
S., and B
¨
uning, H. K. (2013). A learning anomaly de-
tection algorithm for hybrid manufacturing systems.
In The 24th International Workshop on Principles of
Diagnosis (DX-2013), Jerusalem, Israel.
Pedregosa, F., Varoquaux, G., Gramfort, A., Michel, V.,
Thirion, B., Grisel, O., Blondel, M., Prettenhofer,
P., Weiss, R., Dubourg, V., Vanderplas, J., Passos,
A., Cournapeau, D., Brucher, M., Perrot, M., and
Duchesnay, E. (2011). Scikit-learn: Machine learning
in Python. Journal of Machine Learning Research,
12:2825–2830.
Quinlan, J. R. (1986). Induction of decision trees. Machine
learning, 1(1):81–106.
Quinlan, J. R. (1993). C4.5: Programs for Machine Learn-
ing. Morgan Kaufmann Publishers Inc., San Fran-
cisco, CA, USA.
Runkler, T. A. (2012). Data Analytics. Springer.
Sauer, O. (2014). Information technology for the factory of
the future–state of the art and need for action. Proce-
dia CIRP, 25:293–296.
Schilling, S. J. (2015). Contribution to temporal fault tree
analysis without modularization and transformation
into the state space. arXiv preprint arXiv:1505.04511.
Schreiber, T. (2000). Measuring information transfer. Phys-
ical review letters, 85(2):461.
Van Harmelen, F., Lifschitz, V., and Porter, B. (2008).
Handbook of knowledge representation, volume 1. El-
sevier.
Verwer, S. (2010). Efficient Identification of Timed Au-
tomata: Theory and Practice. PhD thesis, Delft Uni-
versity of Technology.
Voigt, T., Flad, S., and Struss, P. (2015). Model-based fault
localization in bottling plants. Advanced Engineering
Informatics, 29(1):101–114.
Wang, T., Zhang, G., Zhao, J., He, Z., Wang, J., and P
´
erez-
Jim
´
enez, M. J. (2015). Fault diagnosis of electric
power systems based on fuzzy reasoning spiking neu-
ral p systems. IEEE Transactions on Power Systems,
30(3):1182–1194.
Xu, L. D. (1994). Case based reasoning. IEEE potentials,
13(5):10–13.
Data-driven Identification of Causal Dependencies in Cyber-Physical Production Systems
601