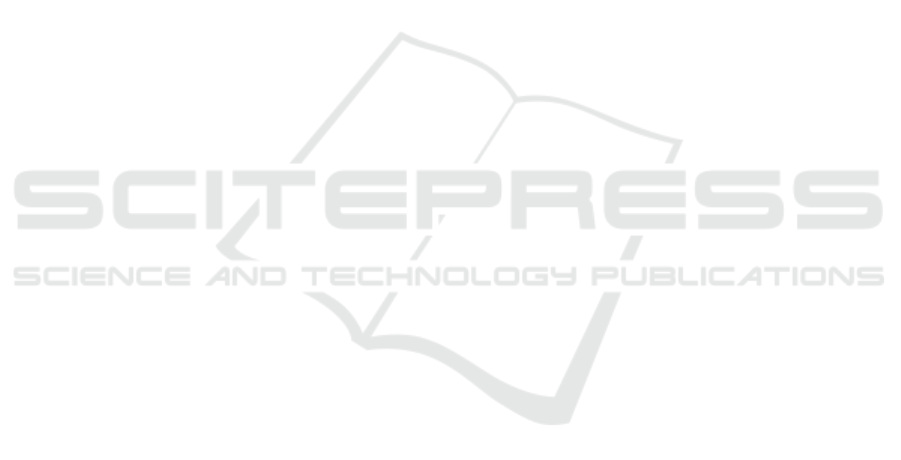
targets; such KPIs are commonly used in Healthcare
as well as for Business and Industrial processes.
Our current framework represents initial work to-
wards developing integrated models for processes,
with the aim of supporting cohesive management and
planning. However, we believe that it also has con-
siderable potential to be extended to include more de-
tailed and explicit models that allow us to assess com-
plex scenarios involving interactions between pro-
cesses. Also, our current analytic model has the ad-
vantage that the results are based on routinely avail-
able data. Another important aspect of extending our
current framework is to consider the distributions and
moments of numbers of process instances complying
with targets for multiple absorbing states, including
processes using Poisson arrivals to describe streamed
data of independent instances; costs can also be asso-
ciated with various options within the model. We plan
to explore such options in further work.
The experience gained and techniques learned are
likely to be relevant to business processes in gen-
eral. Phase-type models have an important role in this
work.
ACKNOWLEDGEMENT
This research is partly supported by BTIIC (BT Ire-
land Innovation Centre), funded by BT and Invest
Northern Ireland and by the Natural Sciences and En-
gineering Research Council of Canada (NSERC).
REFERENCES
Agrawal, R., Imieli
´
nski, T., and Swami, A. (1993). Min-
ing association rules between sets of items in large
databases. In Acm sigmod record, volume 22, pages
207–216. ACM.
Barron, Y., Perry, D., and Stadje, W. (2016). A make-to-
stock production/inventory model with map arrivals
and phase-type demands. Annals of Operations Re-
search, 241(1-2):373–409.
Dobson, A. J. and Barnett, A. G. (2008). An Introduction to
Generalized Linear Models. Chapman and Hall.
Dudin, A., Kim, C., Dudina, O., and Dudin, S. (2016).
Multi-server queueing system with a generalized
phase-type service time distribution as a model of call
center with a call-back option. Annals of Operations
Research, 239(2):401–428.
Dudin, S. A. and Lee, M. H. (2016). Analysis of single-
server queue with phase-type service and energy har-
vesting. Mathematical Problems in Engineering,
2016.
Duong, T., Phung, D., Bui, H. H., and Venkatesh, S.
(2009). Efficient duration and hierarchical modeling
for human activity recognition. Artificial intelligence,
173(7-8):830–856.
Fackrell, M. (2009). Modelling healthcare systems with
phase-type distributions. Health care management
science, 12(1):11.
Faddy, M. and McClean, S. (2005). Markov chain mod-
elling for geriatric patient care. Methods of informa-
tion in medicine, 44(03):369–373.
Fine, S., Singer, Y., and Tishby, N. (1998). The hierarchi-
cal hidden markov model: Analysis and applications.
Machine learning, 32(1):41–62.
Garg, L., McClean, S., Meenan, B., and Millard, P. (2009).
Non-homogeneous markov models for sequential pat-
tern mining of healthcare data. IMA Journal of Man-
agement Mathematics, 20(4):327–344.
Garg, L., McClean, S. I., Barton, M., Meenan, B. J., and
Fullerton, K. (2012). Intelligent patient management
and resource planning for complex, heterogeneous,
and stochastic healthcare systems. IEEE Transactions
on Systems, Man, and Cybernetics-Part A: Systems
and Humans, 42(6):1332–1345.
Gillespie, J., McClean, S., Garg, L., Barton, M., Scotney,
B., and Fullerton, K. (2016). A multi-phase des mod-
elling framework for patient-centred care. Journal of
the Operational Research Society, 67(10):1239–1249.
Griffiths, J. D., Williams, J. E., and Wood, R. (2013).
Modelling activities at a neurological rehabilitation
unit. European Journal of Operational Research,
226(2):301–312.
Haas, P. J. (2006). Stochastic petri nets: Modelling, stabil-
ity, simulation. Springer Science & Business Media.
Jones, B., McClean, S., and Stanford, D. (2018). Modelling
mortality and discharge of hospitalized stroke patients
using a phase-type recovery model. Health care man-
agement science, pages 1–19.
Knight, V. A. and Harper, P. R. (2012). Modelling emer-
gency medical services with phase-type distributions.
Health Systems, 1(1):58–68.
Marshall, A. H. and Zenga, M. (2012). Experimenting with
the coxian phase-type distribution to uncover suitable
fits. Methodology and computing in applied probabil-
ity, 14(1):71–86.
McChesney, I. (2016). Process support for continuous,
distributed, multi-party healthcare processes-applying
workflow modelling to an anticoagulation monitoring
protocol. In International Conference on Ubiquitous
Computing and Ambient Intelligence, pages 255–266.
Springer.
McClean, S., Barton, M., Garg, L., and Fullerton, K.
(2011). A modeling framework that combines markov
models and discrete-event simulation for stroke pa-
tient care. ACM Transactions on Modeling and Com-
puter Simulation (TOMACS), 21(4):25.
Peterson, J. L. (1981). Petri net theory and the modeling of
systems.
Rabiner, L. R. (1989). A tutorial on hidden markov models
and selected applications in speech recognition. Pro-
ceedings of the IEEE, 77(2):257–286.
Tang, X., Luo, Z., and Gardiner, J. C. (2012). Model-
ing hospital length of stay by coxian phase-type re-
Using Phase-type Models to Monitor and Predict Process Target Compliance
89