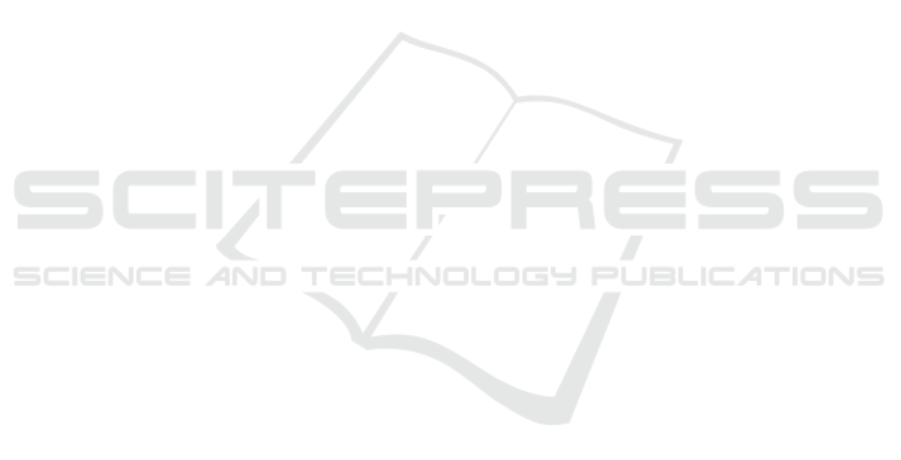
Bellas, A., Bouveyron, C., Cottrell, M., and Lacaille, J.
(2014). Anomaly detection based on confidence in-
tervals using som with an application to health mon-
itoring. In Advances in Self-Organizing Maps and
Learning Vector Quantization, pages 145–155, Cham.
Springer International Publishing.
Brugger, D., Bogdan, M., and Rosenstiel, W. (2008). Auto-
matic cluster detection in kohonen’s som. Trans. Neur.
Netw., 19(3):442–459.
Buczak, A. and Guven, E. (2016). A survey of data min-
ing and machine learning methods for cyber security
intrusion detection. IEEE Communications Surveys &
Tutorials, 18:1153–1176.
Di Pietro, R. and Mancini, L. (2008). Intrusion Detection
Systems. Advances in Information Security. Springer
US.
Ehrenfeld, J. (2017). Wannacry, cybersecurity and health
information technology: A time to act. Journal of
Medical Systems, 41(7):104.
Frank, H. (2009). Industrielle Kommunikation mit Profinet.
https://www.hs-heilbronn.de/1749571/profinet,
accessed on 11.07.2018.
Goldstein, M. and Uchida, S. (2016). A comparative eval-
uation of unsupervised anomaly detection algorithms
for multivariate data. PloS one, 11 4.
Hormann, R., Nikelski, S., Dukanovic, S., and Fischer,
E. (2018). Parsing and extracting features from opc
unified architecture in industrial environments. In
Proceedings of the 2Nd International Symposium on
Computer Science and Intelligent Control, ISCSIC
’18, pages 52:1–52:7, New York, NY, USA. ACM.
Hutchins, E., Cloppert, M., and Amin, R. (2011).
Intelligence-driven computer network defense in-
formed by analysis of adversary campaigns and intru-
sion kill chains. Leading Issues in Information War-
fare & Security Research, 1:80.
Ippoliti, D. and Zhou, X. (2012). A-GHSOM: An adap-
tive growing hierarchical self organizing map for net-
work anomaly detection. J. Parallel Distrib. Comput.,
72(12):1576–1590.
Knapp, E. (2011). Industrial Network Security: Secur-
ing Critical Infrastructure Networks for Smart Grid,
SCADA, and Other Industrial Control Systems. Syn-
gress Publishing.
Kohonen, T. (1982). Self-organized formation of topolog-
ically correct feature maps. Biological Cybernetics,
43(1):59–69.
Kohonen, T. (2014). MATLAB Implementations and Appli-
cations of the Self-Organizing Map. Unigrafia Oy.
Kohonen, T., Schroeder, M. R., and Huang, T. S., editors
(2001). Self-Organizing Maps. Springer-Verlag New
York, Inc., Secaucus, NJ, USA, 3rd edition.
Landress, A. D. (2016). A hybrid approach to reducing the
false positive rate in unsupervised machine learning
intrusion detection. In SoutheastCon 2016, pages 1–
6.
Langner, R. (2011). Stuxnet: Dissecting a cyberwarfare
weapon. IEEE Security Privacy, 9(3):49–51.
Mitrokotsa, A. and Douligeris, C. (2005). Detecting de-
nial of service attacks using emergent self-organizing
maps. In Proceedings of the Fifth IEEE Interna-
tional Symposium on Signal Processing and Informa-
tion Technology, 2005., pages 375–380.
Opolon, D. and Moutarde, F. (2004). Fast semi-automatic
segmentation algorithm for Self-Organizing Maps. In
European Symposium on Artifical Neural Networks
(ESANN’2004), Bruges, Belgium.
Petrovic, S. (2006). A comparison between the silhouette
index and the davies-bouldin index in labelling ids
clusters.
Rousseeuw, P. (1987). Silhouettes: A graphical aid to the in-
terpretation and validation of cluster analysis. J. Com-
put. Appl. Math., 20(1):53–65.
Schuster, F., Paul, A., and K
¨
onig, H. (2013). Towards learn-
ing normality for anomaly detection in industrial con-
trol networks. In Emerging Management Mechanisms
for the Future Internet, pages 61–72, Berlin, Heidel-
berg. Springer Berlin Heidelberg.
Schuster, F., Paul, A., Rietz, R., and Koenig, H. (2015). Po-
tentials of using one-class svm for detecting protocol-
specific anomalies in industrial networks. In 2015
IEEE Symposium Series on Computational Intelli-
gence, pages 83–90.
Sestito, G. S., Turcato, A. C., Dias, A. L., Rocha, M. S.,
da Silva, M. M., Ferrari, P., and Brandao, D. (2018). A
method for anomalies detection in real-time ethernet
data traffic applied to profinet. IEEE Transactions on
Industrial Informatics, 14(5):2171–2180.
Stouffer, K., Falco, J., and Scarfone, K. (2011). Guide to
industrial control systems (ICS) security. Technical
report, National Institute of Standards and Technology
USA, Gaithersburg, MD, United States.
Ultsch, A. (1995). Self-organizing-feature-maps versus sta-
tistical clustering methods: A benchmark.
Vesanto, J. and Alhoniemi, E. (2000). Clustering of the self-
organizing map. Trans. Neur. Netw., 11(3):586–600.
Y
¨
uksel, O., den Hartog, J., and Etalle, S. (2016). Reading
between the fields: Practical, effective intrusion de-
tection for industrial control systems. In Proceedings
of the 31st Annual ACM Symposium on Applied Com-
puting, SAC ’16, pages 2063–2070, New York, NY,
USA. ACM.
Zanero, S. and Savaresi, S. M. (2004). Unsupervised learn-
ing techniques for an intrusion detection system. In
Proceedings of the 2004 ACM Symposium on Applied
Computing, SAC ’04, pages 412–419, New York, NY,
USA. ACM.
ICISSP 2019 - 5th International Conference on Information Systems Security and Privacy
344