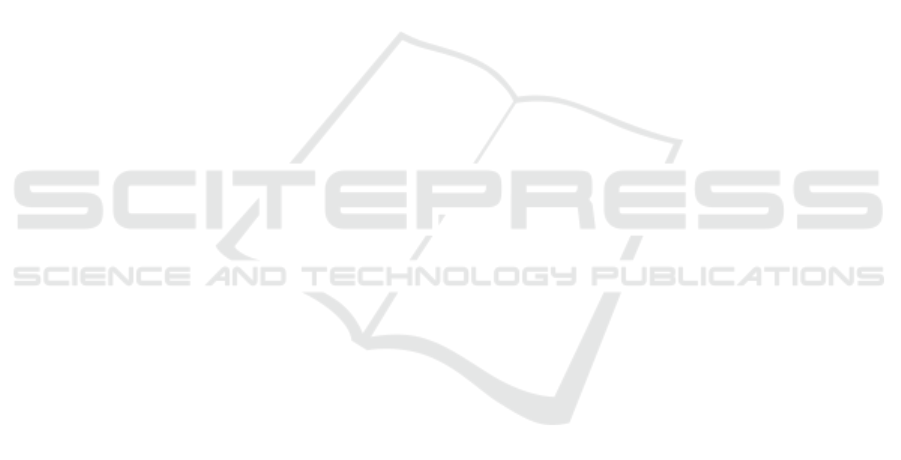
Charles-Smith, L. E., Reynolds, T. L., Cameron, M. A.,
Conway, M., Lau, E. H., Olsen, J. M., Pavlin, J. A.,
Shigematsu, M., Streichert, L. C., Suda, K. J., et al.
(2015). Using social media for actionable disease
surveillance and outbreak management: a systematic
literature review. PloS one, 10(10):e0139701.
Chretien, J.-P., Burkom, H. S., Sedyaningsih, E. R.,
Larasati, R. P., Lescano, A. G., Mundaca, C. C.,
Blazes, D. L., Munayco, C. V., Coberly, J. S., Ashar,
R. J., et al. (2008). Syndromic surveillance: adapting
innovations to developing settings. PLoS medicine,
5(3):e72.
Elliot, A. J., Smith, S., Dobney, A., Thornes, J., Smith,
G. E., and Vardoulakis, S. (2016). Monitoring the
effect of air pollution episodes on health care con-
sultations and ambulance call-outs in england during
march/april 2014: A retrospective observational anal-
ysis. Environmental Pollution, 214:903–911.
S
,
erban, O., Thapen, N., Maginnis, B., Hankin, C., and Foot,
V. (2018). Real-time processing of social media with
sentinel: A syndromic surveillance system incorporat-
ing deep learning for health classification. Information
Processing & Management.
Fennell, K. (2017). Everything you need to know about
repeating social media posts. "[Online; posted 12-
March-2017]".
Ginsberg, J., Mohebbi, M. H., Patel, R. S., Brammer, L.,
Smolinski, M. S., and Brilliant, L. (2009). Detecting
influenza epidemics using search engine query data.
Nature, 457(7232):1012.
Graves, A. and Schmidhuber, J. (2005). Framewise
phoneme classification with bidirectional lstm and
other neural network architectures. Neural Networks,
18(5-6):602–610.
Haykin, S. (1994). Neural networks: a comprehensive foun-
dation. Prentice Hall PTR.
Hornik, K. (1991). Approximation capabilities of mul-
tilayer feedforward networks. Neural networks,
4(2):251–257.
Hripcsak, G. and Rothschild, A. S. (2005). Agreement,
the F-measure, and reliability in information retrieval.
Journal of the American Medical Informatics Associ-
ation, 12(3):296–298.
Jin, L. and Schuler, W. (2015). A comparison of word
similarity performance using explanatory and non-
explanatory texts. In Proceedings of the 2015 Con-
ference of the North American Chapter of the Associa-
tion for Computational Linguistics: Human Language
Technologies, pages 990–994.
Krizhevsky, A., Sutskever, I., and Hinton, G. E. (2012). Im-
agenet classification with deep convolutional neural
networks. In Advances in neural information process-
ing systems, pages 1097–1105.
Lamb, A., Paul, M. J., and Dredze, M. (2013). Separat-
ing fact from fear: Tracking flu infections on twitter.
In Proceedings of the 2013 Conference of the North
American Chapter of the Association for Computa-
tional Linguistics: Human Language Technologies,
pages 789–795.
Le, Q. and Mikolov, T. (2014). Distributed representations
of sentences and documents. In International Confer-
ence on Machine Learning, pages 1188–1196.
Li, J. and Cardie, C. (2013). Early stage influenza detection
from twitter. arXiv preprint arXiv:1309.7340.
Maaten, L. v. d. and Hinton, G. (2008). Visualizing data
using t-sne. Journal of machine learning research,
9(Nov):2579–2605.
Mikolov, T., Chen, K., Corrado, G., and Dean, J. (2013).
Efficient estimation of word representations in vector
space. arXiv preprint arXiv:1301.3781.
Mikolov, T., Karafiát, M., Burget, L.,
ˇ
Cernock
`
y, J., and
Khudanpur, S. (2010). Recurrent neural network
based language model. In Eleventh Annual Confer-
ence of the International Speech Communication As-
sociation.
Pennington, J., Socher, R., and Manning, C. (2014). Glove:
Global vectors for word representation. In Proceed-
ings of the 2014 conference on empirical methods in
natural language processing (EMNLP), pages 1532–
1543.
Requia, W. J., Adams, M. D., Arain, A., Papatheodorou, S.,
Koutrakis, P., and Mahmoud, M. (2018). Global asso-
ciation of air pollution and cardiorespiratory diseases:
a systematic review, meta-analysis, and investigation
of modifier variables. volume 108, pages S123–S130.
American Public Health Association.
Roeder, L. (2018). What twitter’s new rules mean for social
media scheduling. "[Online; posted 13-March-2018]".
Severyn, A. and Moschitti, A. (2015a). Twitter sentiment
analysis with deep convolutional neural networks. In
Proceedings of the 38th International ACM SIGIR
Conference on Research and Development in Infor-
mation Retrieval, pages 959–962. ACM.
Severyn, A. and Moschitti, A. (2015b). Unitn: Training
deep convolutional neural network for twitter senti-
ment classification. In Proceedings of the 9th inter-
national workshop on semantic evaluation (SemEval
2015), pages 464–469.
Triple, S. (2011). Assessment of syndromic surveillance in
europe. Lancet (London, England), 378(9806):1833.
Yin, W., Kann, K., Yu, M., and Schütze, H. (2017). Com-
parative study of cnn and rnn for natural language pro-
cessing. arXiv preprint arXiv:1702.01923.
Zeng, D., Chen, H., Lusch, R., and Li, S.-H. (2010). Social
media analytics and intelligence. volume 25, pages
13–16. IEEE.
ICPRAM 2019 - 8th International Conference on Pattern Recognition Applications and Methods
500